Dual-aware Domain Mining and Cross-aware Supervision forWeakly-supervised Semantic Segmentation
ACM TRANSACTIONS ON KNOWLEDGE DISCOVERY FROM DATA(2023)
摘要
Weakly Supervised Semantic Segmentation with image-level annotation uses localization maps from the classifier to generate pseudo labels. However, such localization maps focus only on sparse salient object regions, it is difficult to generate high-quality segmentation labels, which deviates from the requirement of semantic segmentation. To address this issue, we propose a dual-aware domain mining and cross-aware supervision (DDMCAS) method for weakly-supervised semantic segmentation. Specifically, we propose a dual-aware domain mining (DDM) module consisting of graph-based global reasoning unit and salient-region extension controller, which produces dense localization maps by exploring object features in salient regions and adjacent non-salient regions simultaneously. In order to further bridge the gap between salient regions and adjacent non-salient regions to generate more refined localization maps, we propose a cross-aware supervision (CAS) strategy to recover missing parts of the target objects and enhance weak attention in adjacent non-salient regions, leading to pseudo labels of higher quality for training the segmentation network. Based on the generated pseudo-labels, extensive experiments on PASCAL VOC 2012 dataset demonstrate that our method outperforms state-of-the-art methods using image-level labels for weakly supervised semantic segmentation.
更多查看译文
关键词
Weakly-supervised semantic segmentation, image-level label, dual-aware domain mining, cross-aware supervision
AI 理解论文
溯源树
样例
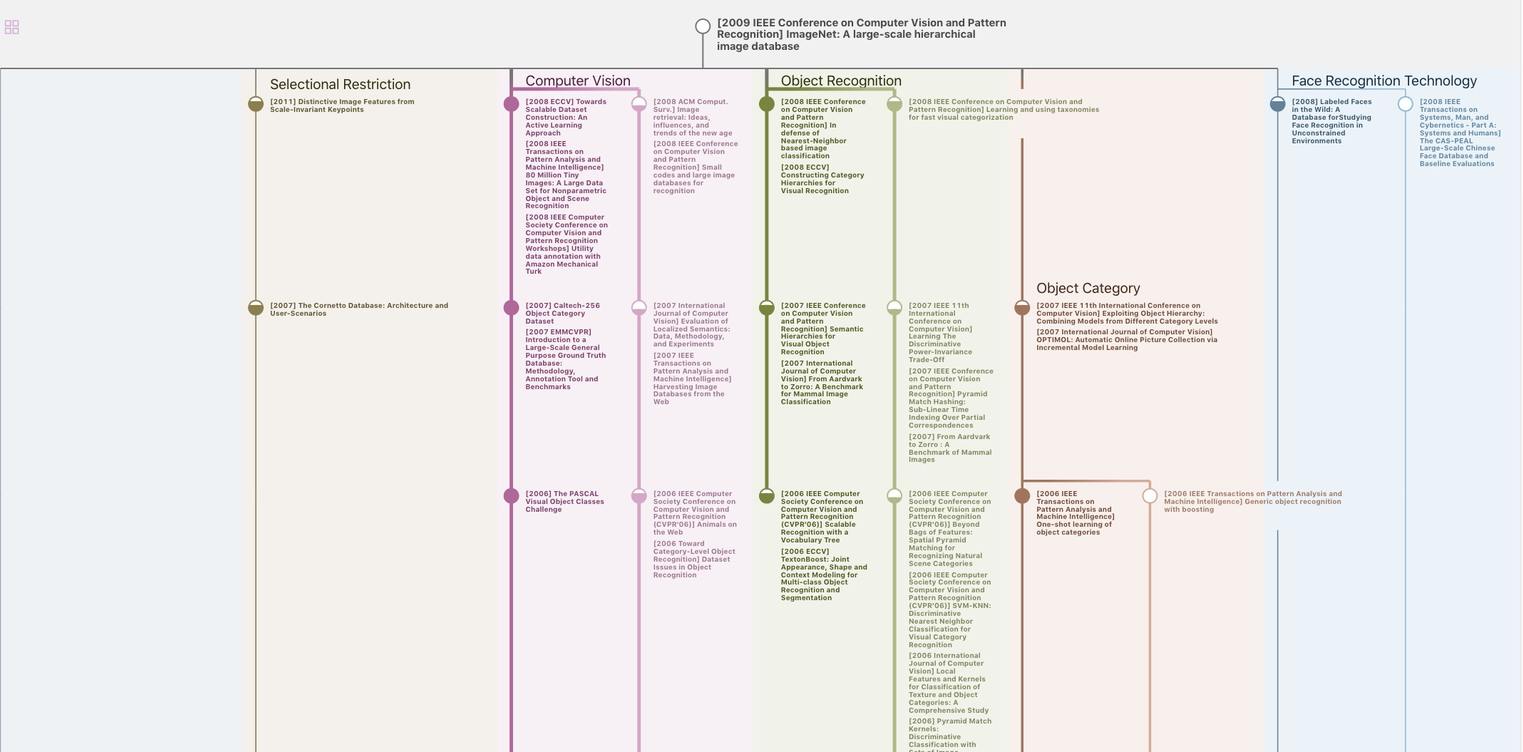
生成溯源树,研究论文发展脉络
Chat Paper
正在生成论文摘要