Performance analysis of state-of-the-art CNN architectures for brain tumour detection
INTERNATIONAL JOURNAL OF IMAGING SYSTEMS AND TECHNOLOGY(2024)
摘要
Deep learning models, such as convolutional neural network (CNN), are popular now a day to solve various complex problems in medical and other fields, such as image classification, object detection, recommendation of images, processing of natural languages and video and image analysis. So, the idea of studying the architecture of CNNs has gotten a lot of attention and become popular. This study analysed and contrasted the performance of many different CNN models trained on the publicly accessible Br35h dataset for the detection of brain tumours. These models included the LeNet, AlexNet, VGG16, VGG19 and ResNet50. Several optimisers were used in this research to fine-tune the performance of the CNN model. These included Adam (adaptive moment estimation), SGD (stochastic gradient descent) and RMSprop (root-mean-square propagation). Accuracy, miss-classification rate, sensitivity, specificity, NPV (negative predictive value), PPV (positive predictive value), F1-score and false omission rate (FOR) were used to assess the efficacy of five different CNN architectures trained using three different optimisers. The experimental results showed that AlexNet architecture with SGD optimiser performed better than other CNN architecture with different optimisers and achieved the highest accuracy of 98.79% with a miss classification rate of 1.20%. It also achieved 98.98% sensitivity, 98.58% specificity, 98.93% NPV, 98.65% PPV, 98.82% F1-score and 1.06% FOR.
更多查看译文
关键词
artificial intelligence,Br35h,brain tumour,deep learning,machine learning,medical image analysis
AI 理解论文
溯源树
样例
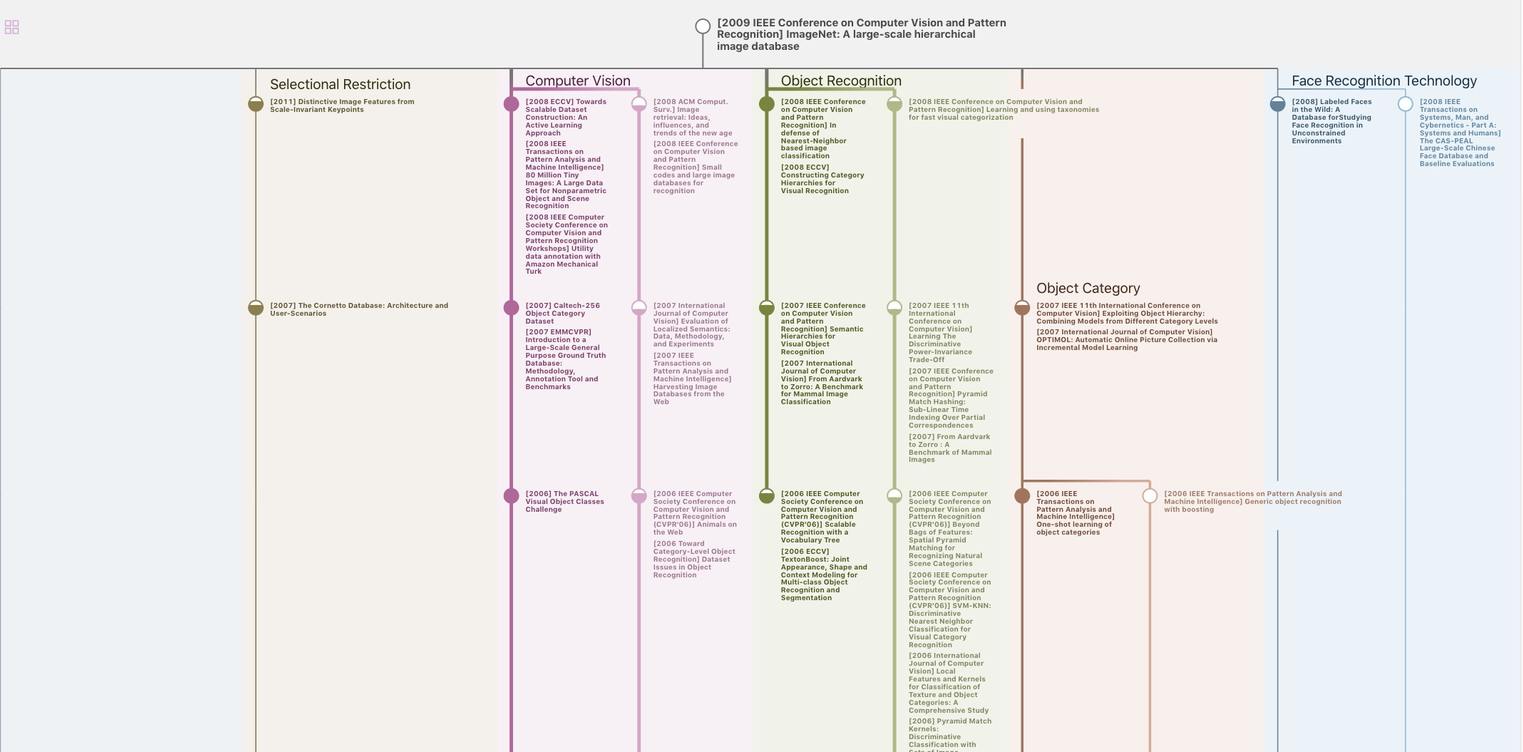
生成溯源树,研究论文发展脉络
Chat Paper
正在生成论文摘要