High-dimensional data mining algorithm based on spca-gwr
JOURNAL OF NONLINEAR AND CONVEX ANALYSIS(2023)
摘要
To improve data fitting accuracy and interpretability of results when analysing multi-spatial influencing factors of high-dimensional data, we propose an SPCA-GWR multi-spatial analysis algorithm for high-dimensional data integrating Sparse Principal Component Analysis (SPCA) and Geographically Weighted Regression (GWR). This algorithm uses Moran's I for spatial auto-correlation analysis, meanwhile, it combines Pearson correlation analysis and SPCA to reduce the data dimensionality efficiently. Then, based on the principal components, the spatial mechanism of each factor is excavated by GWR. The medical insurance dataset of the floating population in Northwest China is used for the experiment, and the comparison experiment is carried out by the PCA-GWR, OLS, and GR algorithms. The results prove that the proposed algorithm improves interpretability and accuracy of the main influencing factors of each space and has significant advantages when fitting data. The SPCA-GWR algorithm can be applied to intelligent information processing systems, which can effectively improve the accuracy of multi-spatial analysis in high-dimensional data.
更多查看译文
关键词
Spatial heterogeneity, data dimensionality reduction, spatial auto-correlation analysis, influencers analysis
AI 理解论文
溯源树
样例
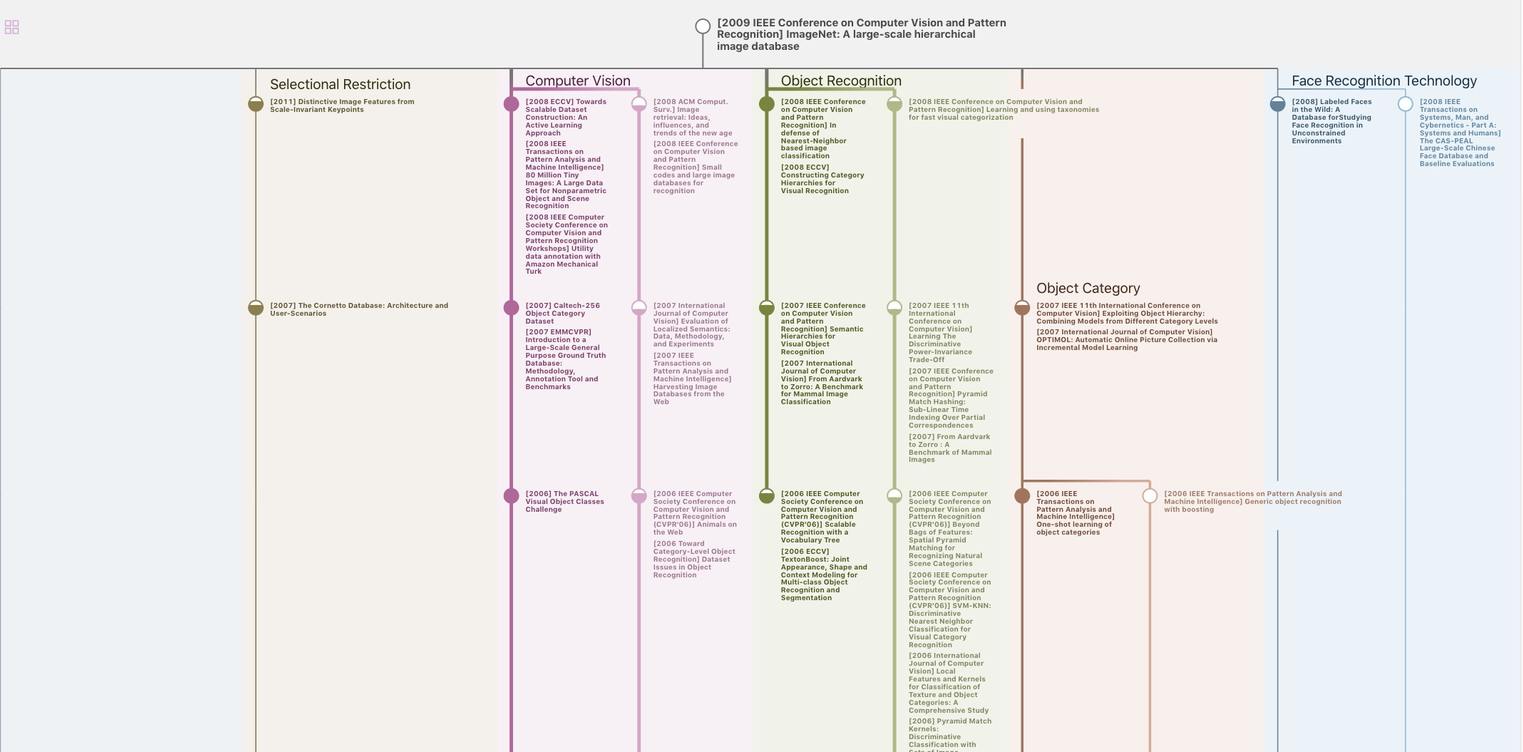
生成溯源树,研究论文发展脉络
Chat Paper
正在生成论文摘要