Differences between Kidney Transplant Recipients from Deceased Donors with Diabetes Mellitus as Identified by Machine Learning Consensus Clustering
JOURNAL OF PERSONALIZED MEDICINE(2023)
摘要
Clinical outcomes of deceased donor kidney transplants coming from diabetic donors currently remain inconsistent, possibly due to high heterogeneities in this population. Our study aimed to cluster recipients of diabetic deceased donor kidney transplants using an unsupervised machine learning approach in order to identify subgroups with high risk of inferior outcomes and potential variables associated with these outcomes. Consensus cluster analysis was performed based on recipient-, donor-, and transplant-related characteristics in 7876 recipients of diabetic deceased donor kidney transplants from 2010 to 2019 in the OPTN/UNOS database. We determined the important characteristics of each assigned cluster and compared the post-transplant outcomes between the clusters. Consensus cluster analysis identified three clinically distinct clusters. Recipients in cluster 1 (n = 2903) were characterized by oldest age (64 & PLUSMN; 8 years), highest rate of comorbid diabetes mellitus (55%). They were more likely to receive kidney allografts from donors that were older (58 & PLUSMN; 6.3 years), had hypertension (89%), met expanded criteria donor (ECD) status (78%), had a high rate of cerebrovascular death (63%), and carried a high kidney donor profile index (KDPI). Recipients in cluster 2 (n = 687) were younger (49 & PLUSMN; 13 years) and all were re-transplant patients with higher panel reactive antibodies (PRA) (88 [IQR 46, 98]) who received kidneys from younger (44 & PLUSMN; 11 years), non-ECD deceased donors (88%) with low numbers of HLA mismatch (4 [IQR 2, 5]). The cluster 3 cohort was characterized by first-time kidney transplant recipients (100%) who received kidney allografts from younger (42 & PLUSMN; 11 years), non-ECD deceased donors (98%). Compared to cluster 3, cluster 1 had higher incidence of primary non-function, delayed graft function, patient death and death-censored graft failure, whereas cluster 2 had higher incidence of delayed graft function and death-censored graft failure but comparable primary non-function and patient death. An unsupervised machine learning approach characterized diabetic donor kidney transplant patients into three clinically distinct clusters with differing outcomes. Our data highlight opportunities to improve utilization of high KDPI kidneys coming from diabetic donors in recipients with survival-limiting comorbidities such as those observed in cluster 1.
更多查看译文
关键词
clustering,diabetic donors,kidney transplant,kidney transplantation,transplantation
AI 理解论文
溯源树
样例
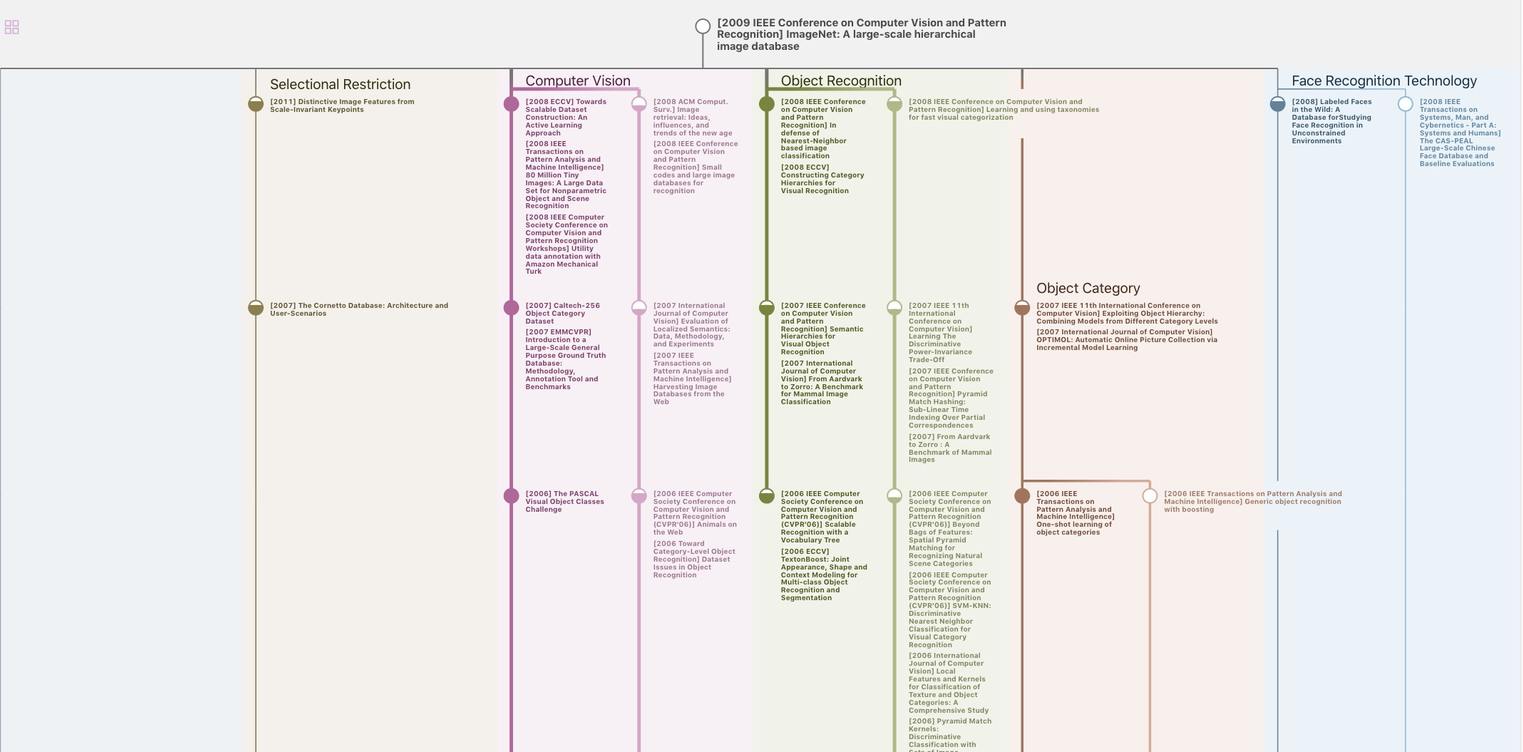
生成溯源树,研究论文发展脉络
Chat Paper
正在生成论文摘要