Direct head-to-head comparison of convolutional long short-term memory and transformer networks for artificial Intelligence-based quantification of atherosclerotic plaque and stenosis from coronary CT angiography
MEDICAL IMAGING 2023(2023)
摘要
Background: Coronary computed tomography angiography (CCTA) enables non-invasive assessment of luminal stenosis and coronary atherosclerotic plaque. We aimed to directly compare the performance of 2 novel deep learning networks-convolutional long short-term memory and transformer network-for artificial intelligence-based quantification of plaque volume and stenosis severity from CCTA. Methods: This was an international multicenter study of patients undergoing CCTA at 11 sites. The deep learning (DL) convolutional neural networks were trained to segment coronary plaque in 921 patients (5,045 lesions). The training dataset was further split temporally into training (80%) and internal validation (20%) datasets. The primary DL architecture was a hierarchical convolutional long short-term memory (ConvLSTM) network. This was compared against a TransUNet network, which combines the abilities of Vision Transformer with U-Net, enabling the capture of in-depth localization information while modeling long-range dependencies. Following training and internal validation, the both DL networks were applied to an external validation cohort of 162 patients (1,468 lesions) from the SCOT-HEART trial. Results: In the external validation cohort, agreement between DL and expert reader measurements was stronger when using the ConvLSTM network than with TransUNet, for both per-lesion total plaque volume (ICC 0.953 vs 0.830) and percent diameter stenosis (ICC 0.882 vs 0.735; both p<0.001). The ConvLSTM network showed higher per-cross-section overlap with expert reader segmentations (as measured by the Dice coefficient) compared to TransUnet, for vessel wall (0.947 vs 0.946), lumen (0.93 vs 0.92), and calcified plaque (0.87 vs 0.86; p<0.0001 for all), with similar execution times. Conclusions: In a direct comparison with external validation, the ConvLSTM network yielded higher agreement with expert readers for quantification of total plaque volume and stenosis severity compared to TransUnet, with faster execution times.
更多查看译文
关键词
artificial intelligence, deep learning, convolutional long short-term memory network, transformers, coronary computed tomography angiography, atherosclerosis
AI 理解论文
溯源树
样例
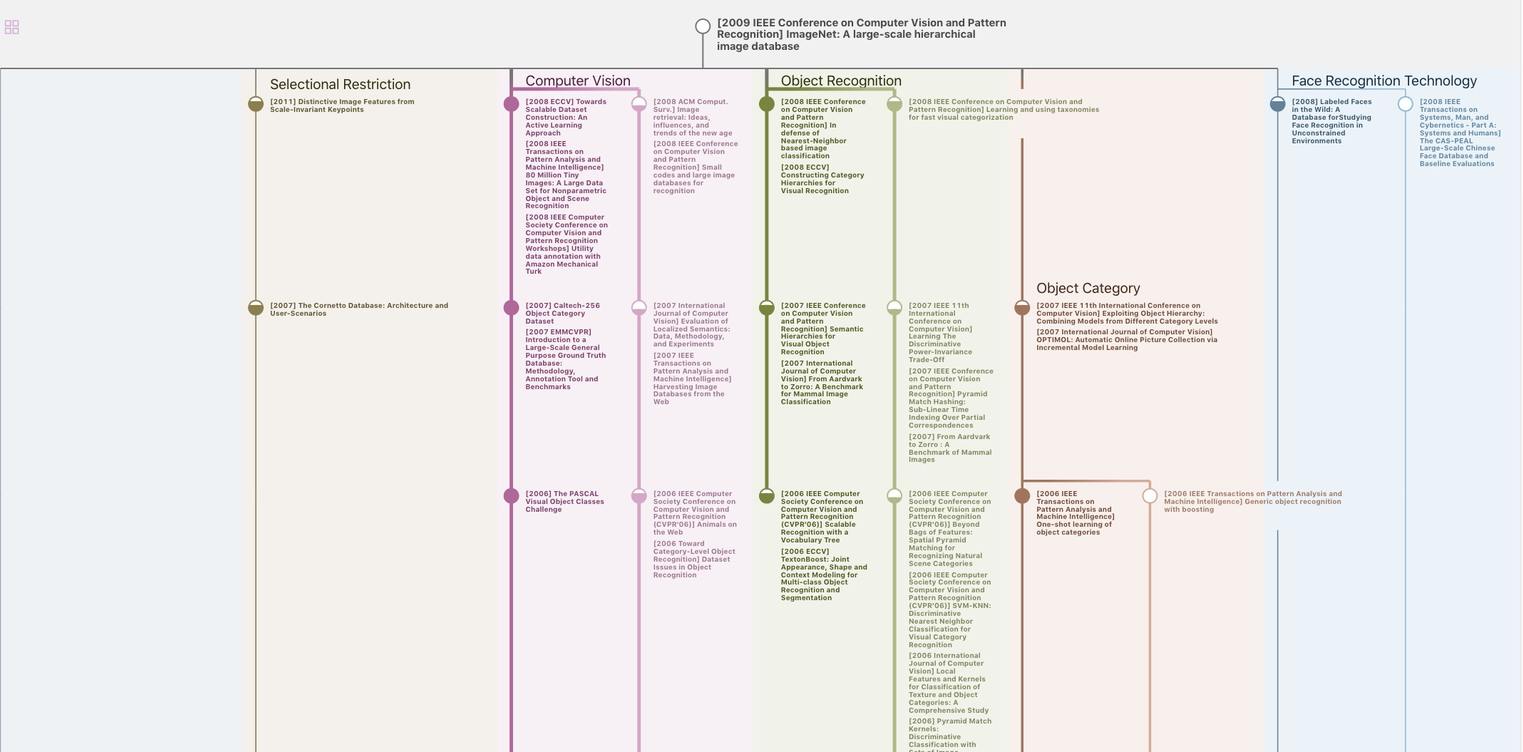
生成溯源树,研究论文发展脉络
Chat Paper
正在生成论文摘要