Effect of Color-Normalization on Deep Learning Segmentation Models for Tumor-Infiltrating Lymphocytes Scoring Using Breast Cancer Histopathology Images
MEDICAL IMAGING 2023(2023)
摘要
Studies have shown that the increased presence of tumor-infiltrating lymphocytes (TILs) is associated with better long-term clinical outcomes and survival, which makes TILs a potentially useful quantitative biomarker. In clinics, pathologists' visual assessment of TILs in biopsies and surgical resections result in a quantitative score (TILs-score). The Tumor-infiltrating lymphocytes in breast cancer (TiGER) challenge is the first public challenge on automated TILs-scoring algorithms using whole slide images of hematoxylin and eosin-stained (H&E) slides of human epidermal growth factor receptor-2 positive (HER2+) and triple-negative breast cancer (TNBC) patients. We participated in the TiGER challenge and developed algorithms for tumor-stroma segmentation, TILs cell detection, and TILs-scoring. The whole slide images in this challenge are from three sources, each with apparent color variations. We hypothesized that color-normalization may improve the cross-source generalizability of our deep learning models. Here, we expand our initial work by implementing a color-normalization technique and investigate its effect on the performance of our segmentation model. We compare the segmentation performance before and after color-normalization by cross validating the models on the three datasets. Our results show a substantial increase in the performance of the segmentation model after color-normalization when trained and tested on different sources. This might potentially improve the model's generalizability and robustness when applied to the external sequestered test set from the TiGER challenge.
更多查看译文
关键词
Deep Learning, Segmentation, Whole Slide Image, Color-Normalization
AI 理解论文
溯源树
样例
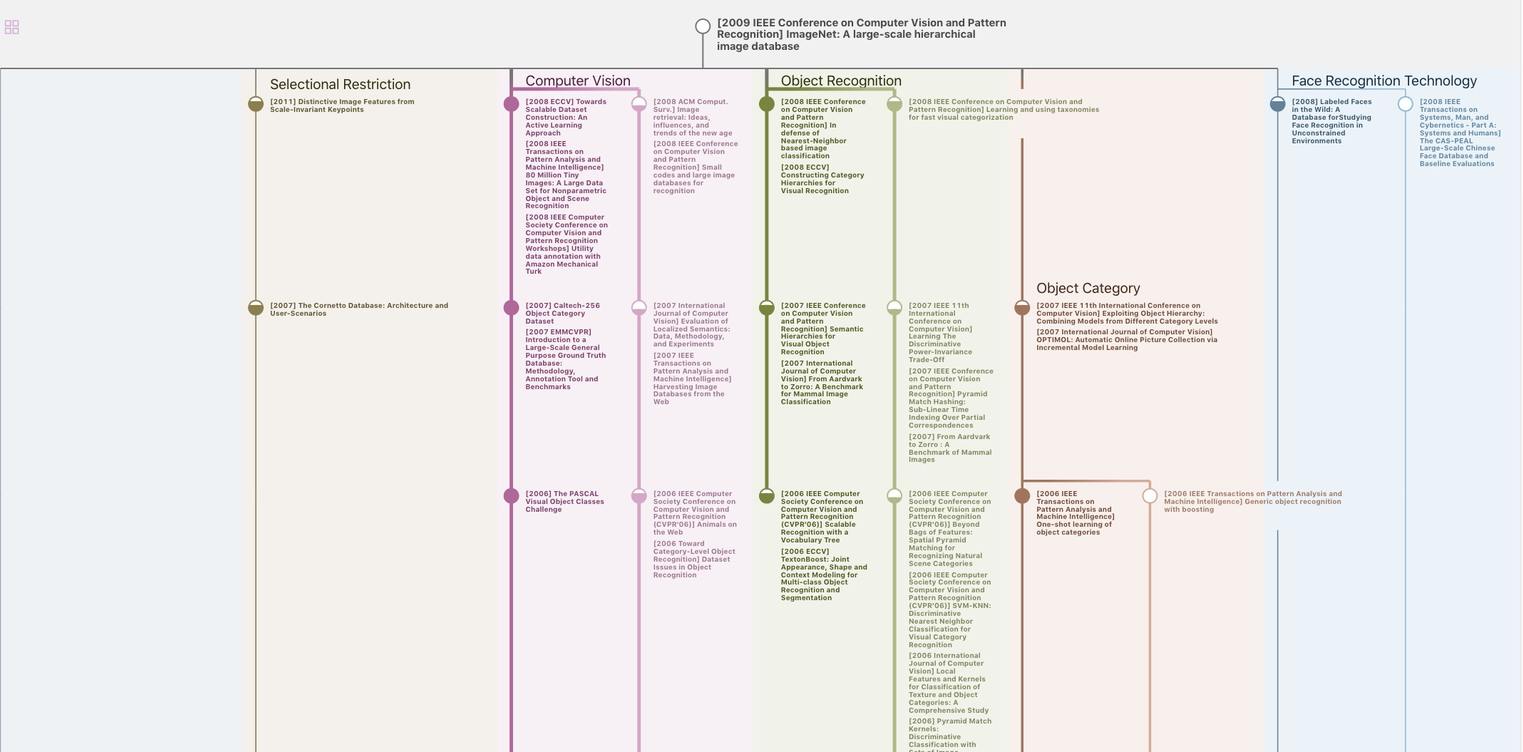
生成溯源树,研究论文发展脉络
Chat Paper
正在生成论文摘要