Fast detection and localization of mitosis using a semi-supervised deep representation
MEDICAL IMAGING 2023(2023)
摘要
Detection, characterization and counting of mitosis is a main biomarker in cancer, allowing diagnosis, histological grading and prognosis. Nonetheless, mitosis identification remains as a challenging task (inter-observer variability up to 20%). Even, the computational support strategies remain limited to include wide visual variability of mitotic patterns, with an inherent bias because labeled observations. This work introduces a semi-supervised scheme that learns to identify mitotic cells from an initial limited amount of labeled data. Then, the initial trained backbone is used to propagate pseudo-labels into training samples. The most challenging samples, i.e., false positive and false negative pseudo-labeled samples, are included in further batches to re-train the model. At each iteration, a set of complementary non-mitotic patches are generated from an auxiliary net. The proposed approach was validated with the public ICPR dataset, achieving competitive results of 0.74 accuracy and 0.78 sensitivity. In addition, the proposed approach achieves an average inference time of 5.21 seconds (on a batch of 240 candidate patches).
更多查看译文
关键词
Mitosis, Deep learning, semi-supervised approach, Histology, Cell nuclei
AI 理解论文
溯源树
样例
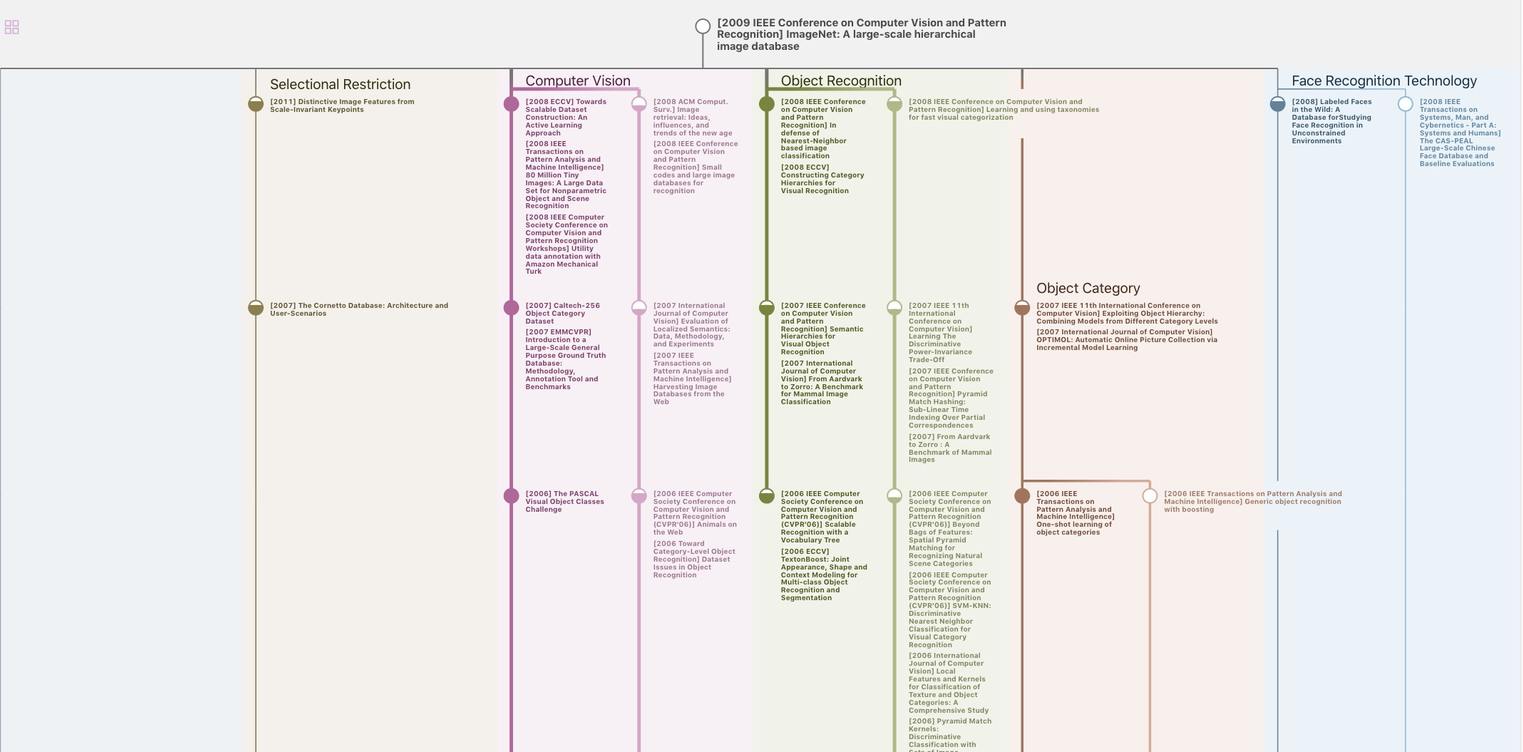
生成溯源树,研究论文发展脉络
Chat Paper
正在生成论文摘要