Unsupervised Quality Assurance for Brain MR Image Rigid Registration using Latent Shape Representation
MEDICAL IMAGING 2023(2023)
摘要
Linear registration to a standard space is a crucial early step in the processing of magnetic resonance images (MRIs) of the human brain. Thus an accurate registration is essential for subsequent image processing steps, as well as downstream analyses. Registration failures are not uncommon due to poor image quality, irregular head shapes, and bad initialization. Traditional quality assurance (QA) for registration requires a substantial manual assessment of the registration results. In this paper, we propose an automatic quality assurance method for the rigid registration of brain MRIs. A variational autoencoder (VAE) model is used to learn shape representations from MRIs after a rigid registration to an MNI standard space. The shape representations are then fed into an anomaly detection model to identify failed registration cases. Without using any manual annotations in the model training, our proposed QA method achieved 99.1% sensitivity and 86.7% specificity in a pilot study on 537 T1-weighted scans acquired from multiple imaging centers.
更多查看译文
关键词
Magnetic resonance imaging, Variational autoencoder, Linear registration, Quality assurance
AI 理解论文
溯源树
样例
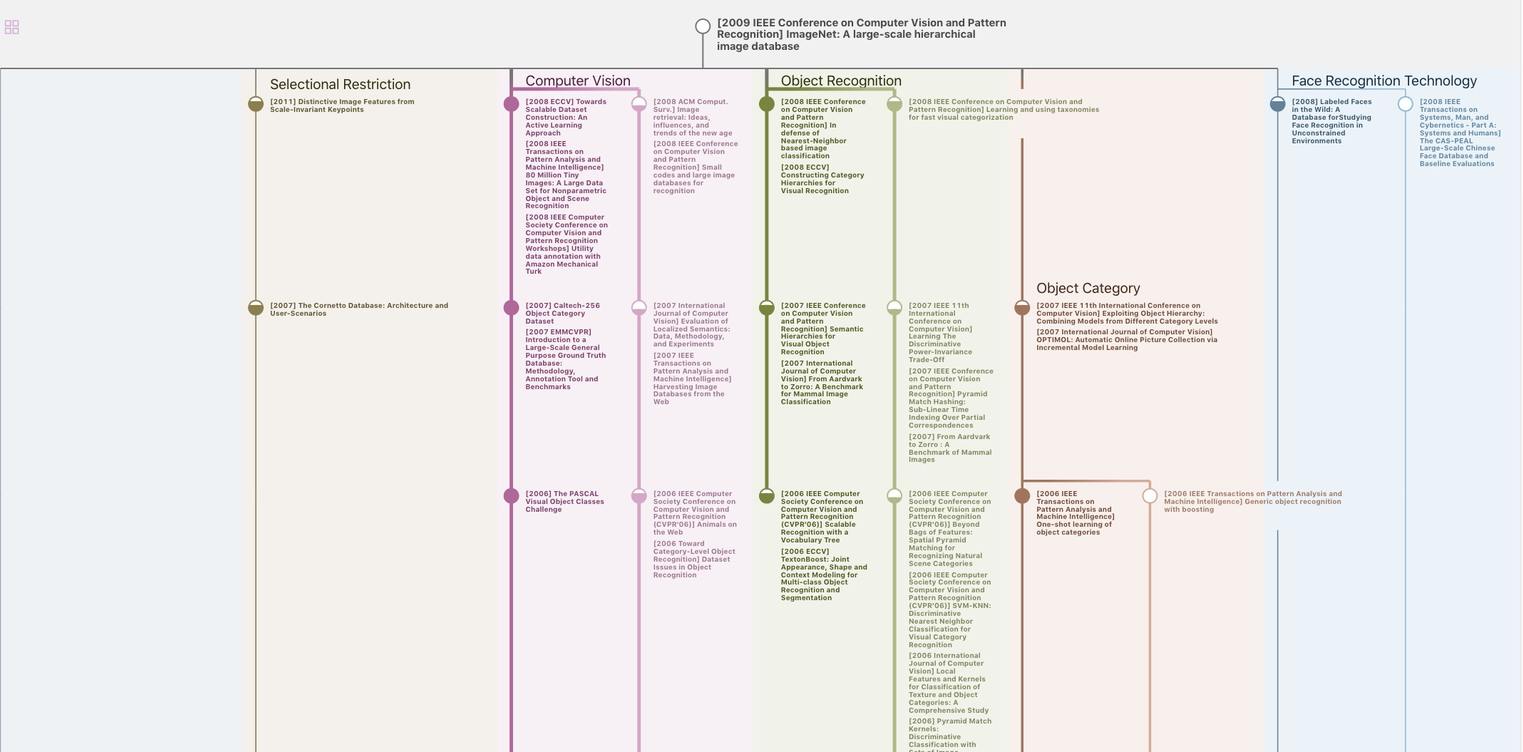
生成溯源树,研究论文发展脉络
Chat Paper
正在生成论文摘要