Investigating the potential of untrained convolutional layers and pruning in computational pathology
MEDICAL IMAGING 2023(2023)
摘要
The effectiveness of untrained convolutional layers for feature extraction in a computational pathology task using real-world data from a necrosis detection dataset is investigated. The study aims to determine whether ImageNet pretrained layers from deep CNNs combined with frozen untrained weights are sufficient for effective necrosis detection in canine Perivascular Wall Tumour (cPWT) whole slide images. Additionally, the authors investigate the impact of pruning CNNs, and whether it can be effective for necrosis detection as this technique can contribute towards reducing memory requirements and improve inference speed in diagnostic settings. The study found that fine-tuning the last (deepest) layers of a pretrained ImageNet model for necrosis detection in cPWT produces the highest test F1-score (0.715) when compared to alternative set ups. This score is further improved to 0.754 when the results are optimised using an optimal threshold predetermined on maximising the validation set F1-score. Resetting weights (untrained) and freezing the last few convolutional layers in the last dense block also demonstrated some capability in necrosis detection with an optimised F1-score of 0.747, still outperforming models trained from scratch as well as an ImageNet pretrained feature extraction model. Pruning the fine-tuned model using lower thresholds also showed the potential to improve performance, however thresholds higher than 0.40 negatively impacted performance.
更多查看译文
关键词
deep learning, digital pathology, computational pathology, necrosis, pruning, untrained convolutional layers, densenet-161
AI 理解论文
溯源树
样例
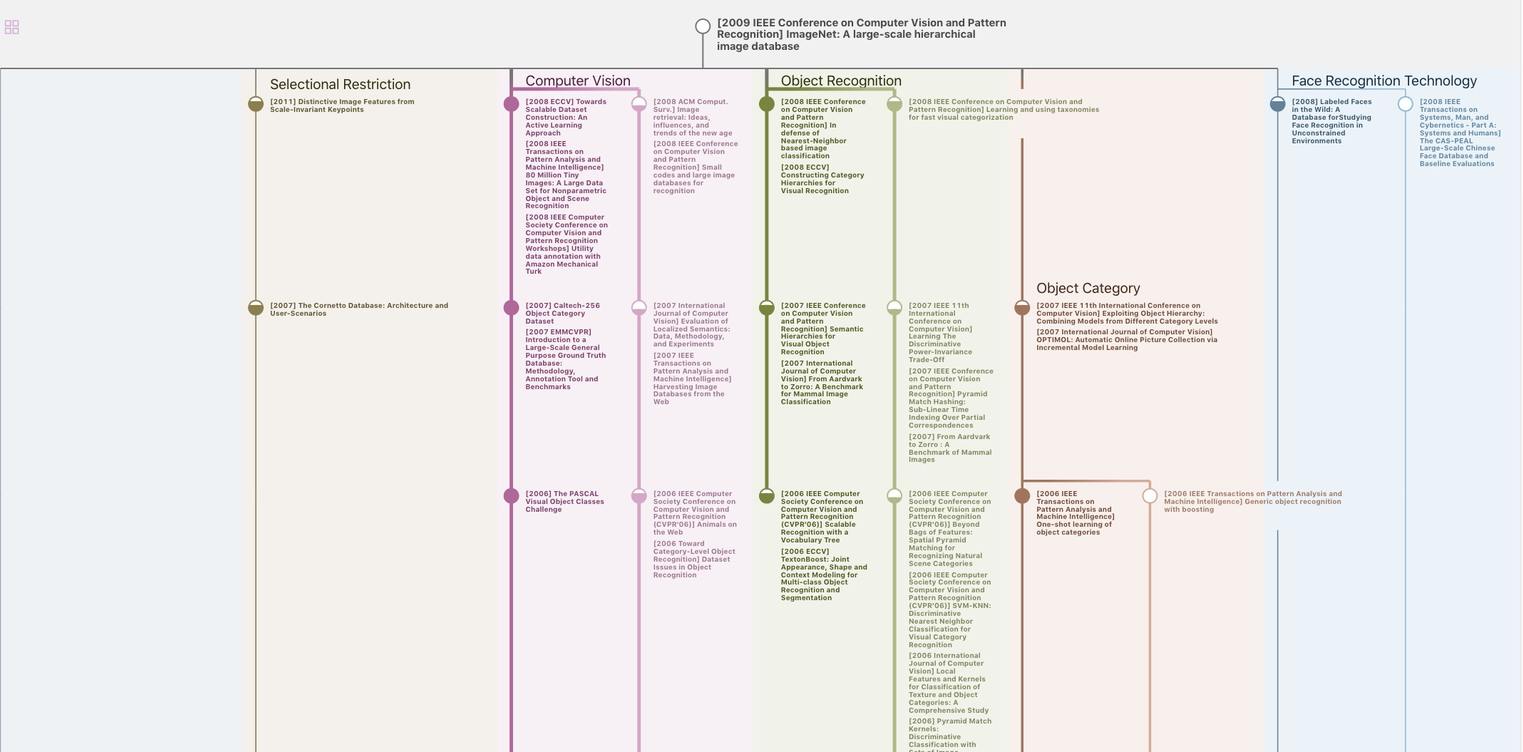
生成溯源树,研究论文发展脉络
Chat Paper
正在生成论文摘要