Data-Driven Modeling of Air Traffic Controllers' Policy to Resolve Conflicts
AEROSPACE(2023)
摘要
With the aim to enhance automation in conflict detection and resolution (CD & R) tasks in the air traffic management (ATM) domain, this article studies the use of artificial intelligence and machine learning (AI/ML) methods to learn air traffic controllers' (ATCOs) policy in resolving conflicts among aircraft assessed to violate separation minimum constraints during the en route phase of flights, in the tactical phase of operations. The objective is to model how conflicts are being resolved by ATCOs. Towards this goal, the article formulates the ATCO policy learning problem for conflict resolution, addresses the challenging issue of an inherent lack of information in real-world data, and presents AI/ML methods that learn models of ATCOs' behavior. The methods are evaluated using real-world datasets. The results show that AI/ML methods can achieve good accuracy on predicting ATCOs' actions given specific conflicts, revealing the preferences of ATCOs for resolution actions in specific circumstances. However, the high accuracy of predictions is hindered by real-world data-inherent limitations.
更多查看译文
关键词
air traffic management,conflict detection and resolution,machine learning
AI 理解论文
溯源树
样例
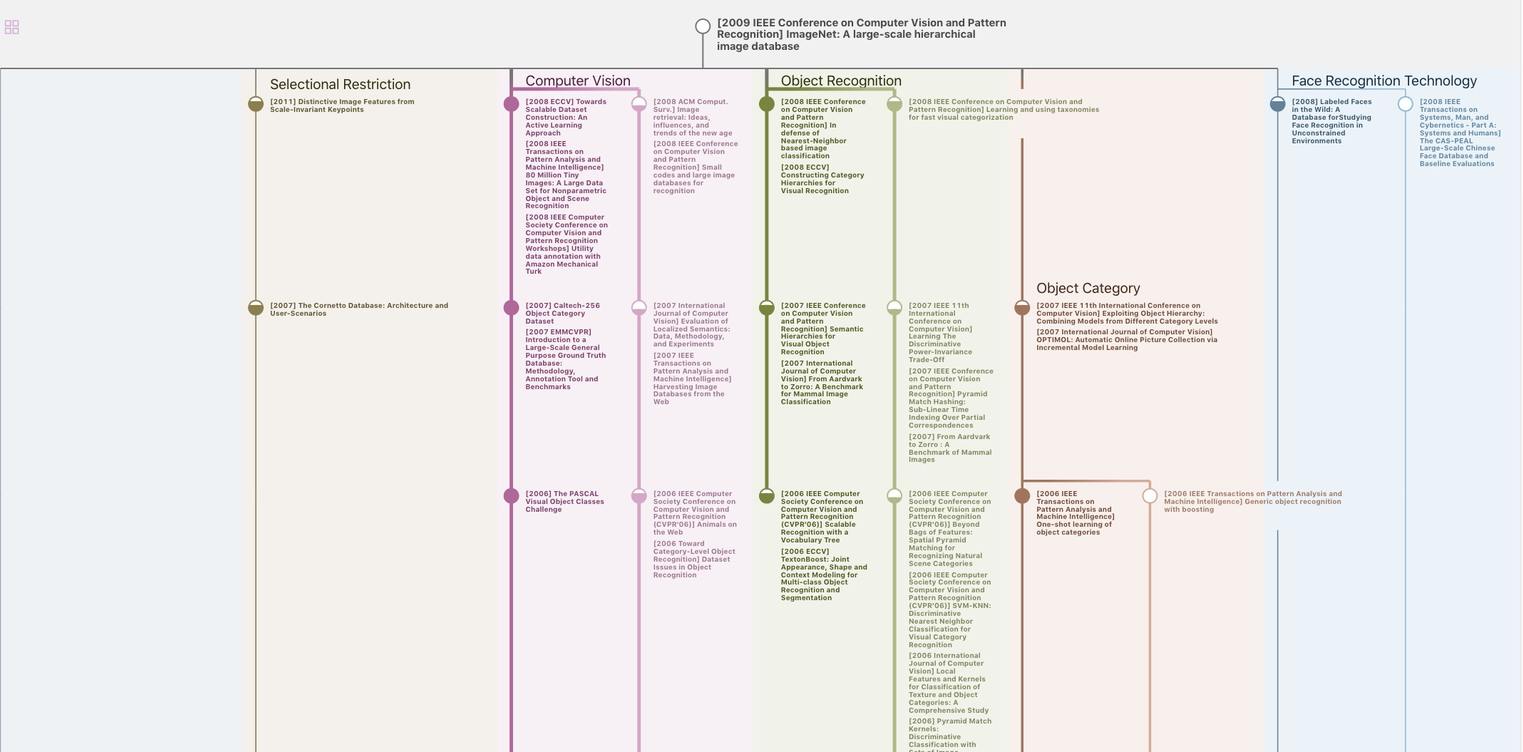
生成溯源树,研究论文发展脉络
Chat Paper
正在生成论文摘要