Deep learning techniques for noise annoyance detection: Results from an intensive workshop at the Alan Turing Institute
JOURNAL OF THE ACOUSTICAL SOCIETY OF AMERICA(2023)
摘要
Advancements in AI and ML have enabled us to combine automated sound source recognition and deep learning models for predicting subjective soundscape perception. We held a multidisciplinary, cross-institutional Data Study Group (DSG) to investigate how sound source information could be incorporated into deep learning models for predicting urban noise annoyance. We used a large-scale dataset of 2980 15-s recordings paired with 12 210 annoyance ratings (from 1 to 10) and sound source labels. A total of 14 neural networks and 4 conventional ML models were built. The best model was trained to simultaneously predict sound source labels and annoyance rating. It achieved an RMSE = 1.07 for annoyance prediction and AUROC = 0.88 for label classification, while a similarly structured model trained to predict annoyance ratings only (i.e., no sound source information) achieved RMSE = 1.13. Results showed that including sound source labels as a simultaneous training output, rather than as an explicit model input resulted in the best performance. Overall, these models performed very well at predicting both annoyance ratings and identifying sources, providing a starting bed for automated annoyance detection systems. This presentation will provide context for the DSG and present conclusions drawn regarding approaches to applying deep learning techniques to noise annoyance detection.
更多查看译文
关键词
noise annoyance detection,deep learning
AI 理解论文
溯源树
样例
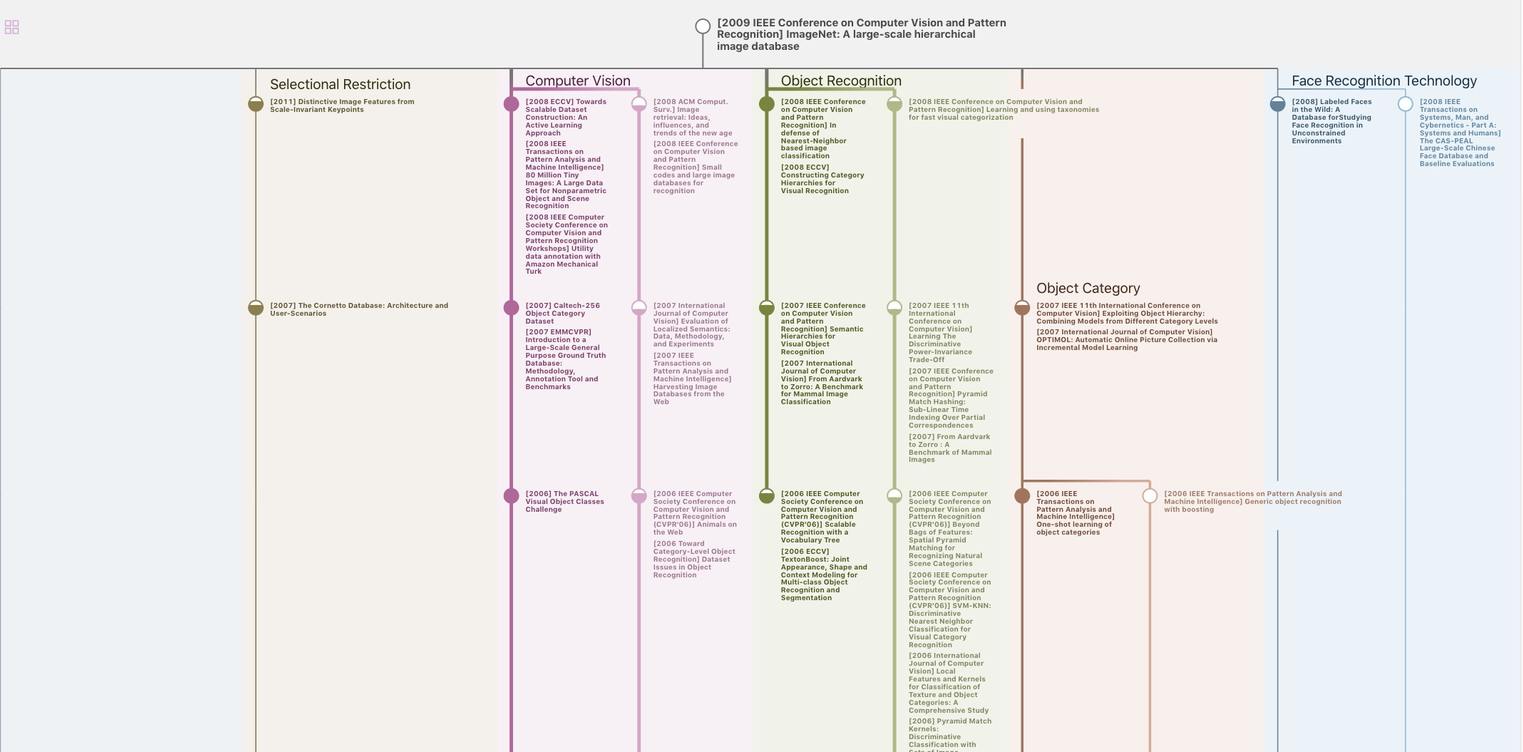
生成溯源树,研究论文发展脉络
Chat Paper
正在生成论文摘要