CAMV: A Crash Alarm Model for Vehicles Based on Internet of Vehicles Data
IEEE TRANSACTIONS ON INTELLIGENT TRANSPORTATION SYSTEMS(2023)
摘要
Predicting vehicle crashes is critical to improve urban transportation safety. It is however challenging to alarm impending crashes accurately as the volume of non-crash data dominates that of crash data. Most existing studies formulate the crash prediction as retrospective study and a binary classification problem, which offers the limited capacity of alarming crashes in advance. To fill such gap, this paper proposes a crash alarm model for vehicles (CAMV) developed via learning vehicle operational data. First, a non-crash learning block (NCLB) is developed to process time slices of operational data with a fixed length. It aims to model the inter-feature correlations and the temporal dependencies jointly. Next, a novel coarse-to-fine strategy is developed to selectively generate and calibrate alarms. Specifically, a statistical process control (SPC) module is developed at the coarse step to generate preliminary alarms with crash scores under an abnormal pattern. A self-calibration module (SCM) is next developed to eliminate false alarms and confirm the ultimate crash alarm. Experimental results verify the effectiveness of the proposed CAMV with crash data collected from Internet of Vehicles. Meanwhile, comparative experiments based on a set of benchmarking methods are conducted to verify the superiority of our proposal.
更多查看译文
关键词
Data-driven model, crash prediction, vehicles, multivariate time series, transportation safety
AI 理解论文
溯源树
样例
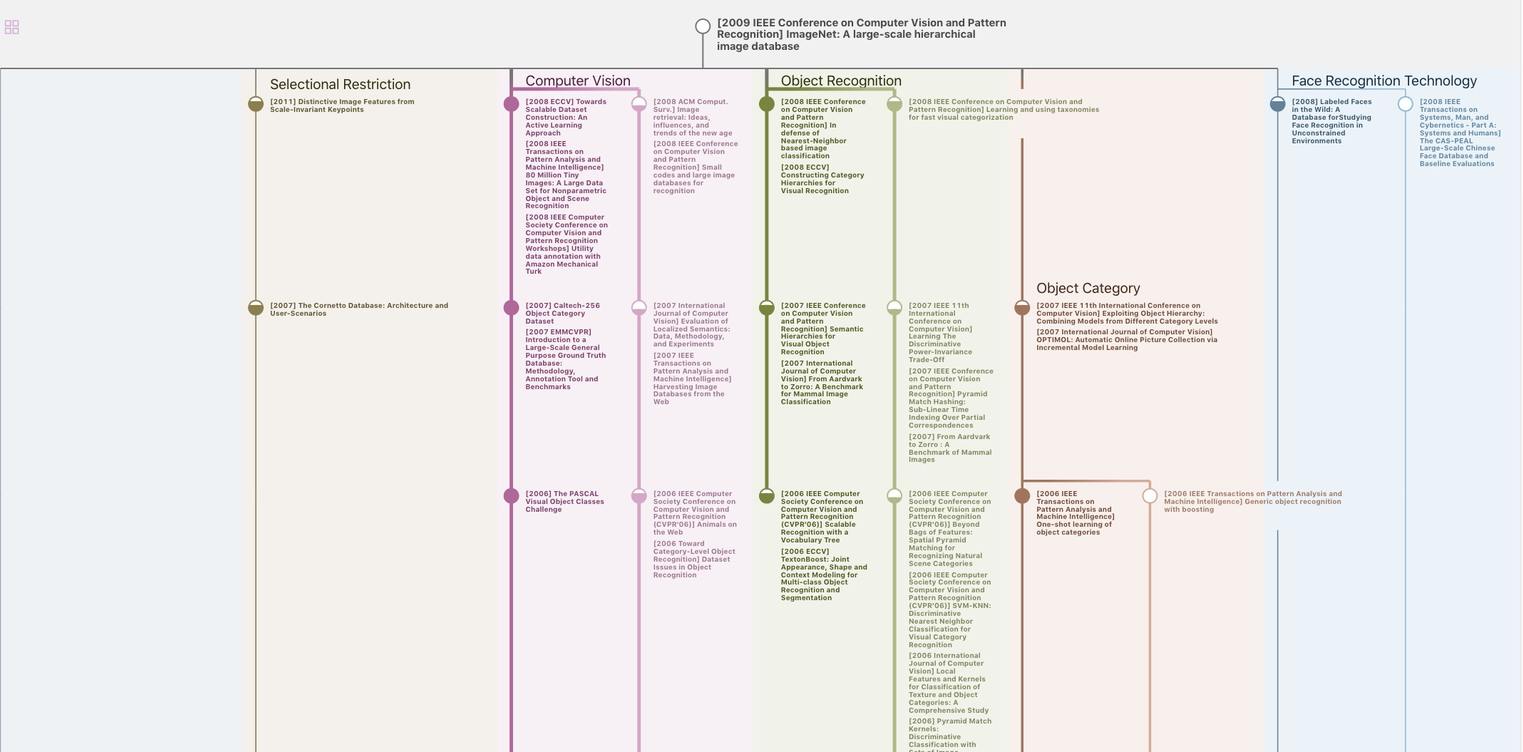
生成溯源树,研究论文发展脉络
Chat Paper
正在生成论文摘要