Topological enhanced graph neural networks for semi-supervised node classification
APPLIED INTELLIGENCE(2023)
摘要
The complexity and non-Euclidean structure of graph data hinders the development of data augmentation methods similar to those in computer vision. In this paper, we propose a feature augmentation method for graph nodes based on topological regularization, in which topological structure information is introduced into an end-to-end model to promote better learning of node representation. Specifically, we first obtain topology embedding of nodes through Node2vec, an unsupervised graph feature learning method based on random walk. Then, the topological embedding as additional features and the original node features are input into a Symmetric Graph Neural Network framework for propagation, and two different high-order neighborhood representations of the nodes are obtained. On this basis, we propose a regularization technique to bridge the differences between the two different node representations, eliminate the adverse effects caused by the topological features of graphs directly used, and greatly improve the performance. Our framework can be effectively combined with other graph neural network models, and can effectively prevent over-smoothing of deep graph network. Experimental results on five datasets confirm the effectiveness of our method.
更多查看译文
关键词
graph,classification,neural networks,semi-supervised
AI 理解论文
溯源树
样例
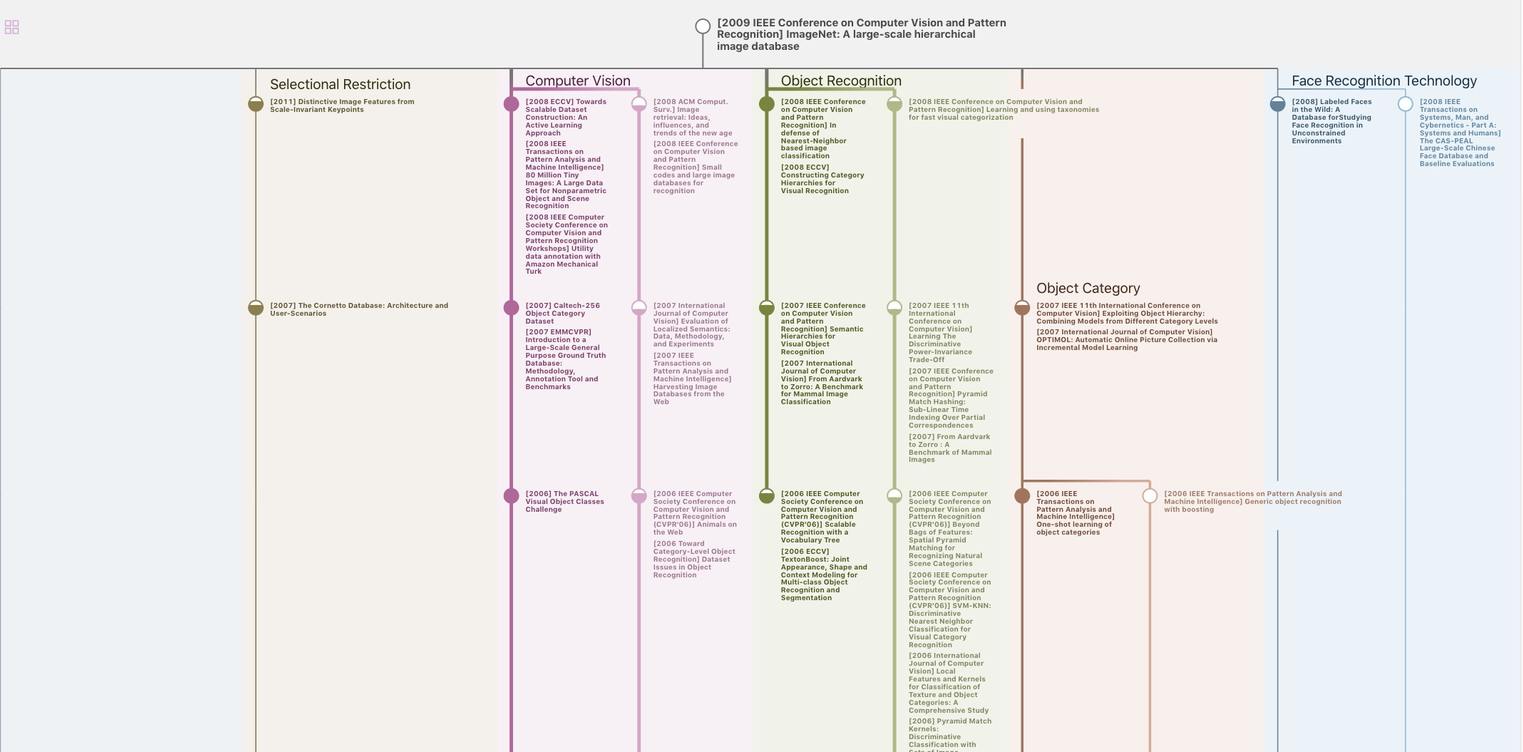
生成溯源树,研究论文发展脉络
Chat Paper
正在生成论文摘要