A survey on conventional and learning-based methods for multi-view stereo
PHOTOGRAMMETRIC RECORD(2023)
摘要
3D reconstruction of scenes using multiple images, relying on robust correspondence search and depth estimation, has been thoroughly studied for the two-view and multi-view scenarios in recent years. Multi-view stereo (MVS) algorithms aim to generate a rich, dense 3D model of the scene in the form of a dense point cloud or a triangulated mesh. In a typical MVS pipeline, the robust estimations for the camera poses along with the sparse points obtained from structure from motion (SfM) are used as input. During this process, the depth of generally every pixel of the scene is to be calculated. Several methods, either conventional or, more recently, learning-based have been developed for solving the correspondence search problem. A vast amount of research exists in the literature using local, global or semi-global stereomatching approaches, with the PatchMatch algorithm being among the most popular and efficient conventional ones in the last decade. Yet, and despite the widespread evolution of the algorithms, yielding complete, accurate and aesthetically pleasing 3D representations of a scene remains an open issue in real-world and large-scale photogrammetric applications. This work aims to provide a concrete survey on the most widely used MVS methods, investigating underlying concepts and challenges. To this end, the theoretical background and relative literature are discussed for both conventional and learning-based approaches, with a particular focus on close-range 3D reconstruction applications.
更多查看译文
关键词
multi‐view
AI 理解论文
溯源树
样例
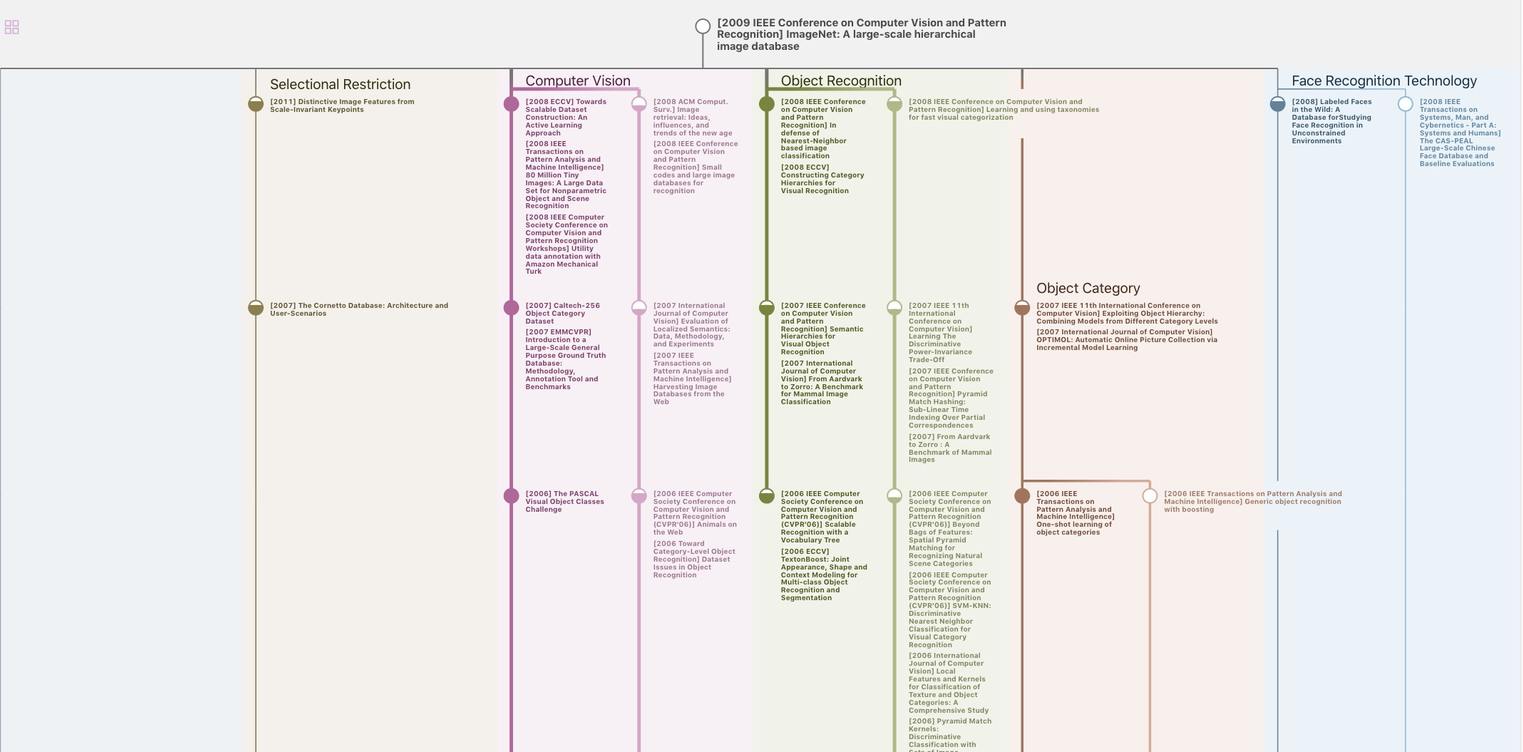
生成溯源树,研究论文发展脉络
Chat Paper
正在生成论文摘要