Estimation of Planning Investments with Scarce Data - comparing LASSO, Bayesian and CMLR
2023 19TH INTERNATIONAL CONFERENCE ON THE EUROPEAN ENERGY MARKET, EEM(2023)
摘要
A common problem in distribution planning is the scarcity of historic data (training examples) relative to the number of variables, meaning that most data-driven techniques cannot be applied in such situations, due to the risk of overfitting. Thus, the suitable regression techniques are restrained to efficient models, preferably with embedded regularization features. This article compares three of these techniques: LASSO, Bayesian and CMLR (Conditioned multi-linear regression - a new approach developed within the scope of a project with a distribution company). The results showed that each technique has its own advantages and limitations. The Bayesian regression has the main advantage of providing inherent confidence intervals. The LASSO is a very economic and efficient regression tool. The CMLR is versatile and provided the best performance.A common problem in distribution planning is the scarcity of historic data (training examples) relative to the number of variables, meaning that most data-driven techniques cannot be applied in such situations, due to the risk of overfitting. Thus, the suitable regression techniques are restrained to efficient models, preferably with embedded regularization features. This article compares three of these techniques: LASSO, Bayesian and CMLR (Conditioned multi-linear regression - a new approach developed within the scope of a project with a distribution company). The results showed that each technique has its own advantages and limitations. The Bayesian regression has the main advantage of providing inherent confidence intervals. The LASSO is a very economic and efficient regression tool. The CMLR is versatile and provided the best performance.
更多查看译文
关键词
Mandatory Investments,Distribution Network Planning,Cost Estimation,LASSO,Bayesian regression,Spike and slab
AI 理解论文
溯源树
样例
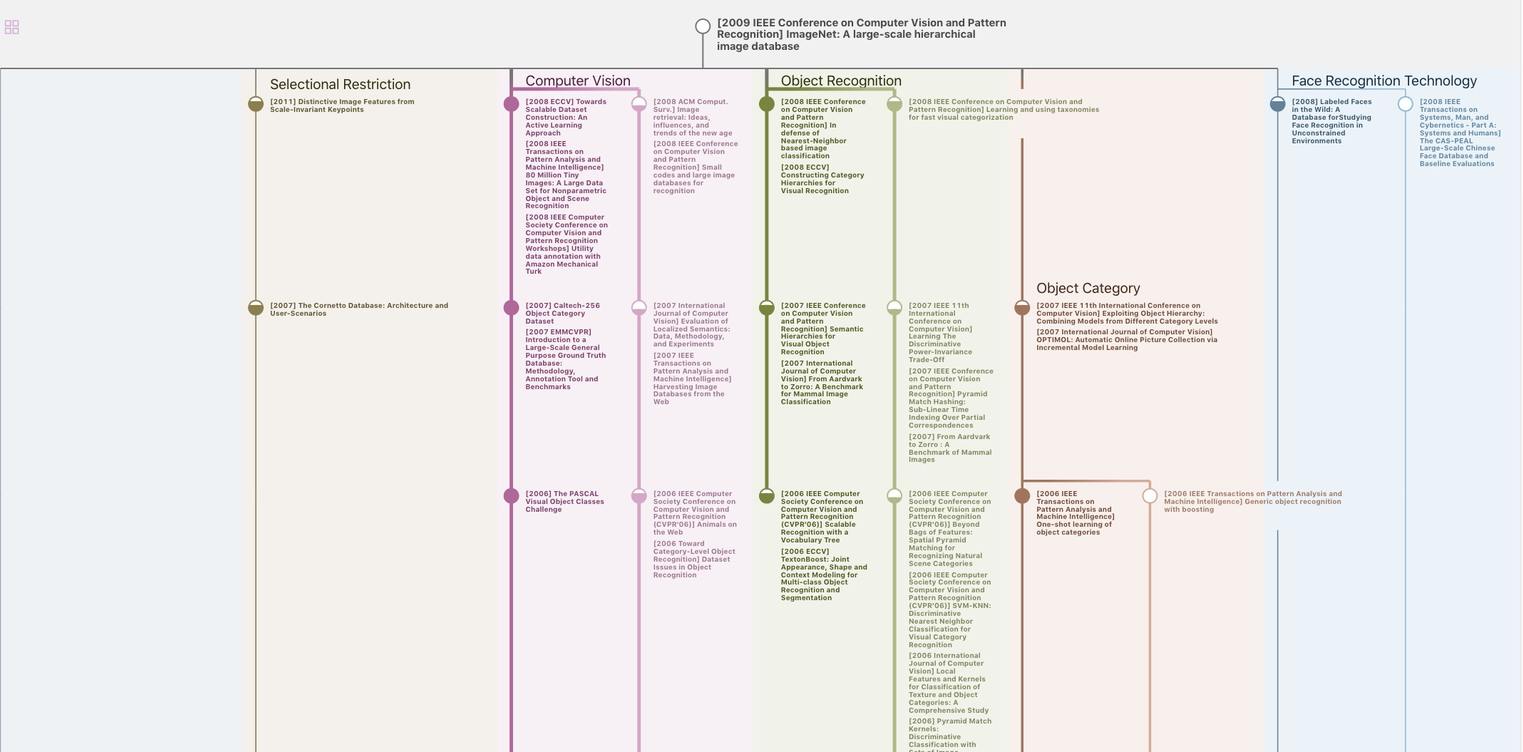
生成溯源树,研究论文发展脉络
Chat Paper
正在生成论文摘要