Towards ARSPI-Net: development of an efficient hybrid deep learning framework
2023 IEEE LONG ISLAND SYSTEMS, APPLICATIONS AND TECHNOLOGY CONFERENCE, LISAT(2023)
摘要
In this paper, we implement and experiment with a portion of our overall hybrid deep learning framework, An Affective hYbrid SPIking Neural Network [ARSPI-NET]. In order to motivate and show the usage of liquid state machines as an efficient, feature extraction framework we perform experiments on connection architecture as well as neuron model and their effect on overall classification performance. We perform our initial experimentation on the MNIST dataset and achieve a 87% classification using a liquid state machine and logistic regression classifier. Our results suggest that our framework can compare with current models in terms of accuracy, however, we outperform traditional deep learning methods in terms of energy consumption and the potential to move to energy-efficient neuromorphic platforms. In addition, our framework has the advantage of being more interpretable, as it allows us to model the spatiotemporal dynamics of signals through the usage of a liquid reservoir and an interpretable readout vector. This paper sets precedence for future experimentation and development of ARSPI-Net as a hybrid deep learning framework.
更多查看译文
关键词
Deep Learning,Recurrent Neural Networks,Spiking Neural Networks,Affective Computing,Neuomorphic,energy efficiency neural networks,TinyML,edge computing
AI 理解论文
溯源树
样例
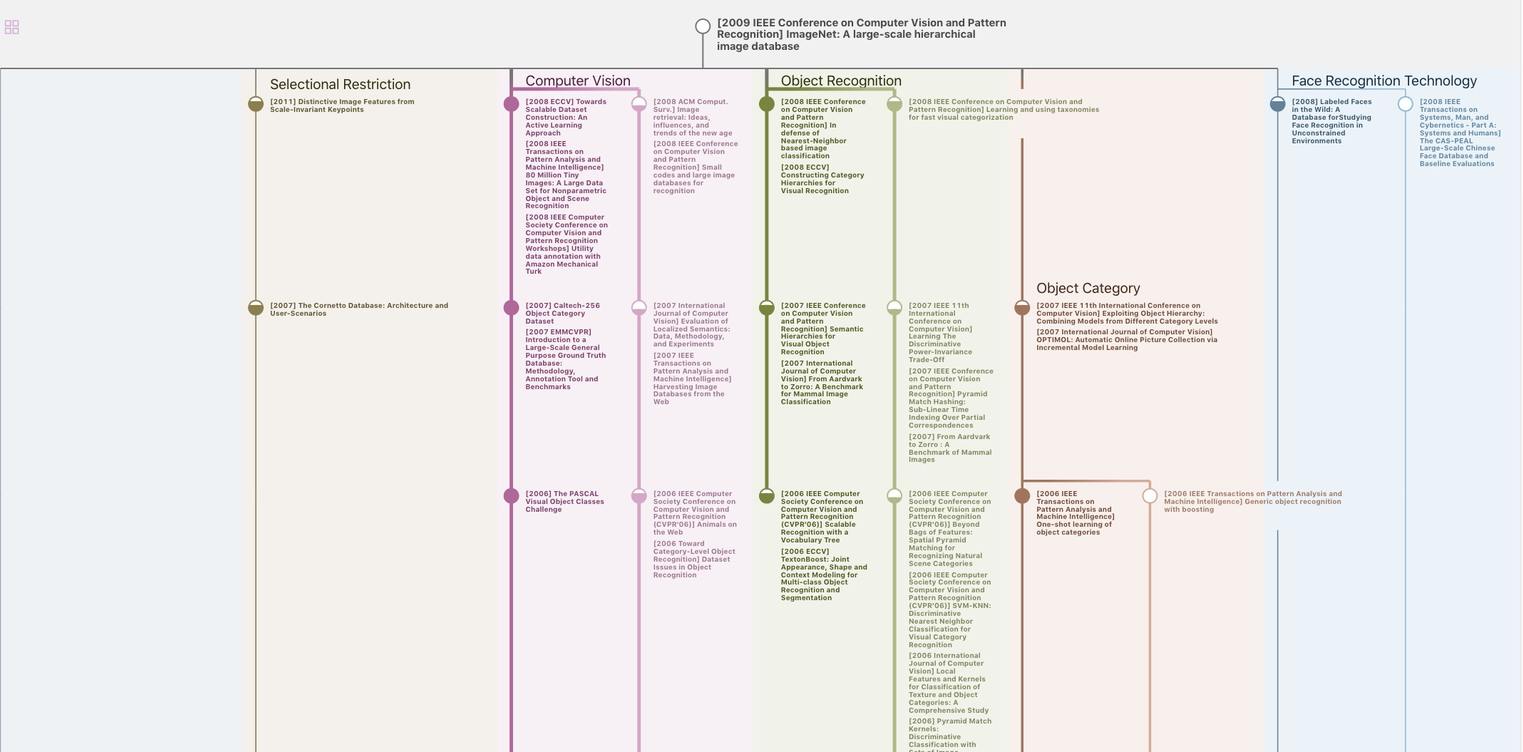
生成溯源树,研究论文发展脉络
Chat Paper
正在生成论文摘要