Developing an Optimal Ensemble Model to Estimate Building Demolition Waste Generation Rate
SUSTAINABILITY(2023)
摘要
Smart management of construction and demolition (C & D) waste is imperative, and researchers have implemented machine learning for estimating waste generation. In Korea, the management of demolition waste (DW) is important due to old buildings, and it is necessary to predict the amount of DW to manage it. Thus, this study employed decision tree (DT)-based ensemble models (i.e., random forest-RF, extremely randomized trees-ET, gradient boosting machine-GBM), and extreme gradient boost-XGboost) based on data characteristics (i.e., small datasets with categorical inputs) to predict the demolition waste generation rate (DWGR) of buildings in urban redevelopment areas. As a result of the study, the RF and GBM algorithms showed better prediction performance than the ET and XGboost algorithms. Especially, RF (6 features, 450 estimators; mean, 1169.94 kg & BULL;m(-2)) and GBM (4 features, 300 estimators; mean, 1166.25 kg & BULL;m(-2)) yielded the top predictive performances. In addition, feature importance affecting DWGR was found to have a significant impact on the order of gross floor area (GFA) > location > roof material > wall material. The straightforward collection of features used here can facilitate benchmarking as a decision-making tool in demolition waste management plans for industry stakeholders and policy makers. Therefore, in the future, it is required to improve the predictive performance of the model by updating additional data and building a reliable dataset.
更多查看译文
关键词
optimal ensemble model,building,waste,estimate
AI 理解论文
溯源树
样例
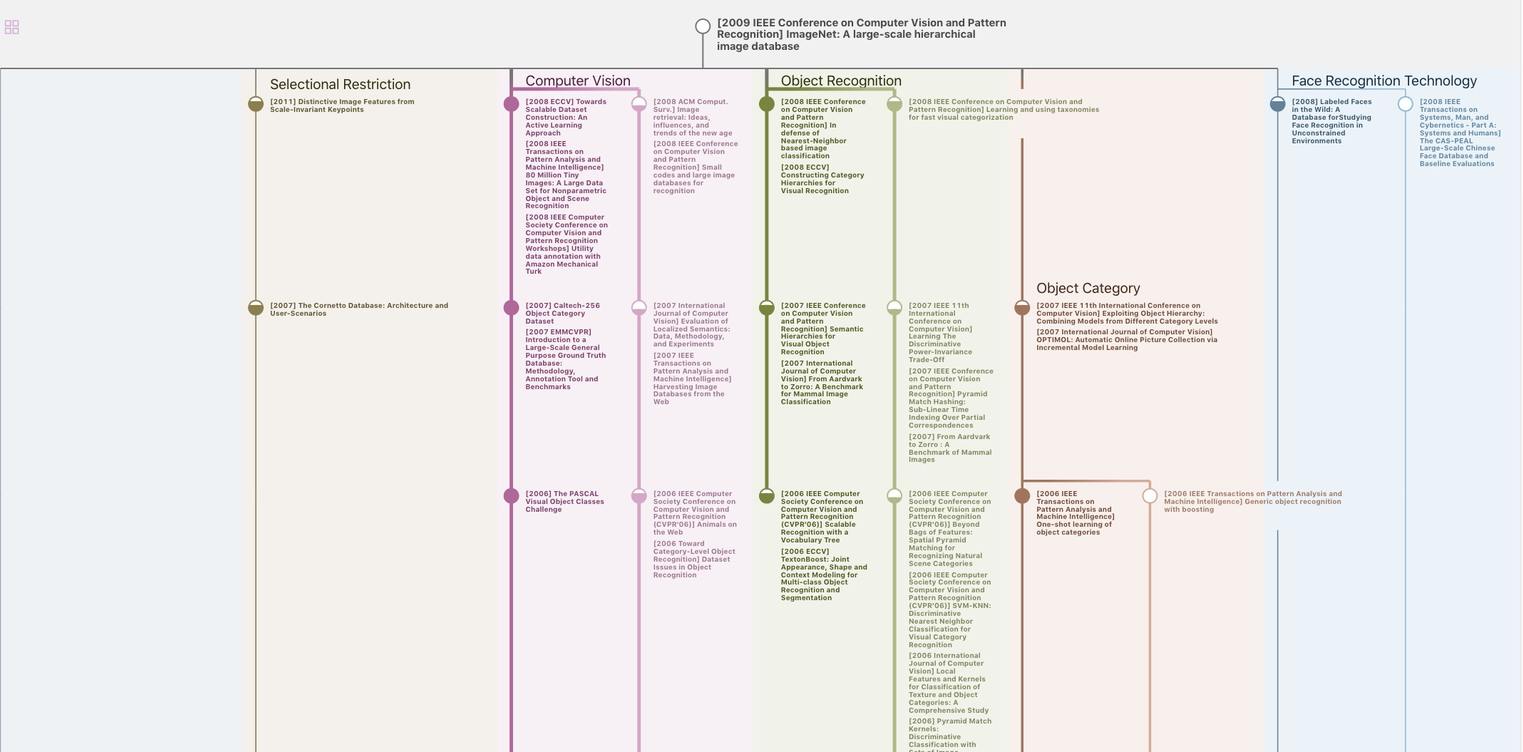
生成溯源树,研究论文发展脉络
Chat Paper
正在生成论文摘要