Improving Artificial IntelligenceLBased on Pediatric Skin Lesions
JOURNAL OF INVESTIGATIVE DERMATOLOGY(2023)
摘要
Artificial intelligence algorithms to classify melanoma are dependent on their training data, which limits generalizability. The objective of this study was to compare the performance of an artificial intelligence model trained on a standard adult-predominant dermoscopic dataset before and after the addition of additional pediatric training images. The performances were compared using held-out adult and pediatric test sets of images. We trained two models: one (model A) on an adult-predominant dataset (37,662 images from the International Skin Imaging Collaboration) and the other (model A thorn P) on an additional 1,536 pediatric images. We compared performance between the two models on adult and pediatric held-out test images separately using the area under the receiver operating characteristic curve. We then used Gradient-weighted Class Activation Maps and background skin masking to understand the contributions of the lesion versus background skin to algorithm decision making. Adding images from a pediatric population with different epidemiological and visual patterns to current reference standard datasets improved algorithm performance on pediatric images without diminishing performance on adult images. This suggests a way that dermatologic artificial intelligence models can be made more generalizable. The presence of background skin was important to the pediatricspecific improvement seen between models. Our study highlights the importance of carefully curated and labeled data from diverse inputs to improve the generalizability of AI models for dermatology, in this case applied to dermoscopic images of adult and pediatric lesions to improve melanoma detection.
更多查看译文
AI 理解论文
溯源树
样例
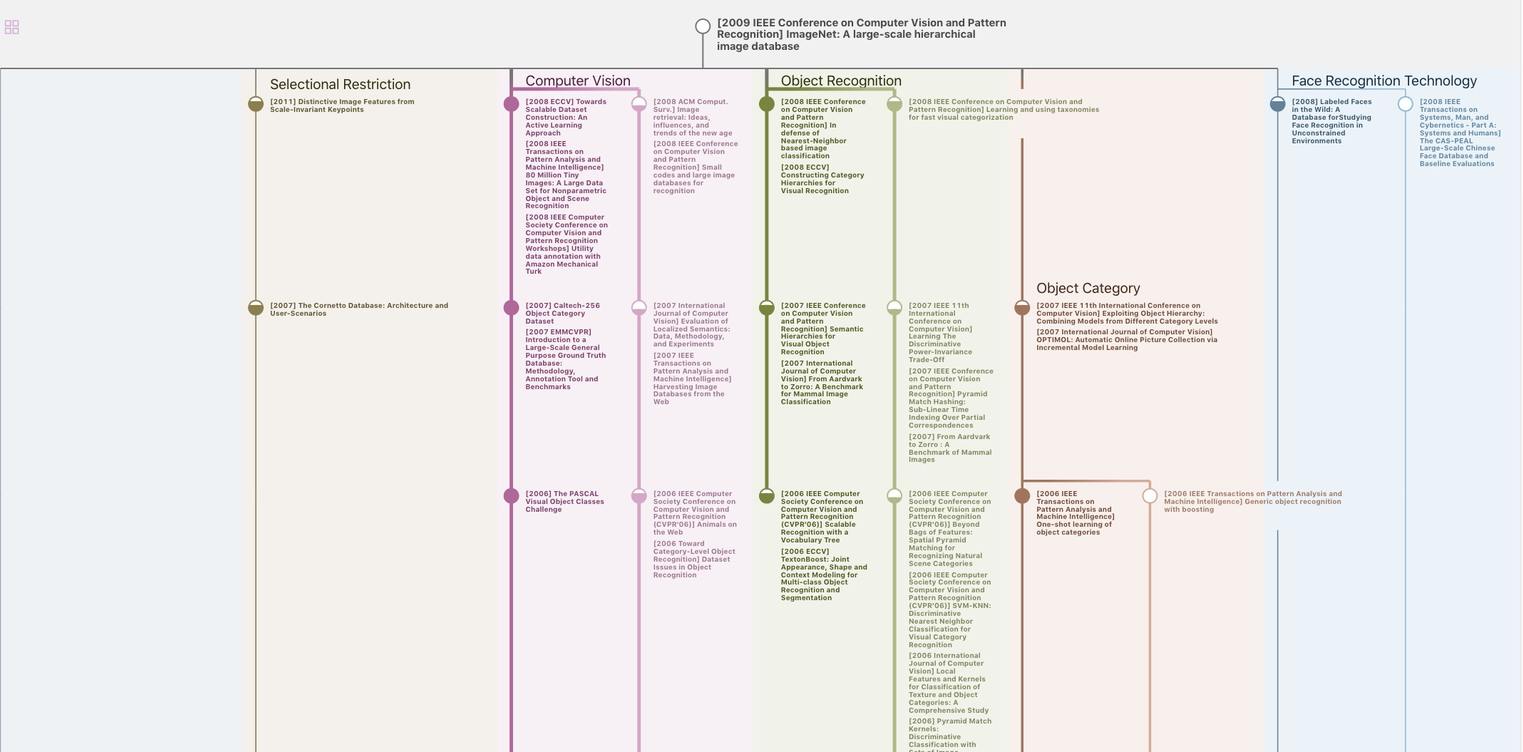
生成溯源树,研究论文发展脉络
Chat Paper
正在生成论文摘要