Discovering the ultralow thermal conductive A 2 B 2 O 7-type high-entropy oxides through the hybrid knowle dge-assiste d data-driven machine learning
JOURNAL OF MATERIALS SCIENCE & TECHNOLOGY(2024)
摘要
Lattice engineering and distortion have been considered one kind of effective strategies for discovering advanced materials. The instinct chemical flexibility of high-entropy oxides (HEOs) motivates/accelerates to tailor the target properties through phase transformations and lattice distortion. Here, a hybrid knowledge-assisted data-driven machine learning (ML) strategy is utilized to discover the A2B2O7-type HEOs with low thermal conductivity ( K) through 17 rare-earth (RE = Sc, Y, La-Lu) solutes optimized A-site. A designing routine integrating the ML and high throughput first principles has been proposed to predict the key physical parameter (KPPs) correlated to the targeted K of advanced HEOs. Among the smart-designed 6188 (5RE0.2)2Zr2O7 HEOs, the best candidates are addressed and validated by the princi-ples of severe lattice distortion and local phase transformation, which effectively reduce K by the strong multi-phonon scattering and weak interatomic interactions. Particularly, (Sc0.2Y0.2La0.2Ce0.2Pr0.2)2Zr2O7 with predicted K below 1.59 Wm -1 K -1 is selected to be verified, which matches well with the ex-perimental K = 1.69 Wm -1 K -1 at 300 K and could be further decreased to 0.14 Wm -1 K -1 at 1473 K. Moreover, the coupling effects of lattice vibrations and charges on heat transfer are revealed by the cross-validations of various models, indicating that the weak bonds with low electronegativity and few bond -ing charge density and the lattice distortion ( r *) identified by cation radius ratio (rA/rB) should be the KPPs to decrease K efficiently. This work supports an intelligent designing strategy with limited atomic and electronic KPPs to accelerate the development of advanced multi-component HEOs with proper-ties/performance at multi-scales.& COPY; 2023 Published by Elsevier Ltd on behalf of The editorial office of Journal of Materials Science & Technology.
更多查看译文
关键词
High-entropy oxides,Thermal conductivity,Pyrochlore,Key physical parameter,First-Principles
AI 理解论文
溯源树
样例
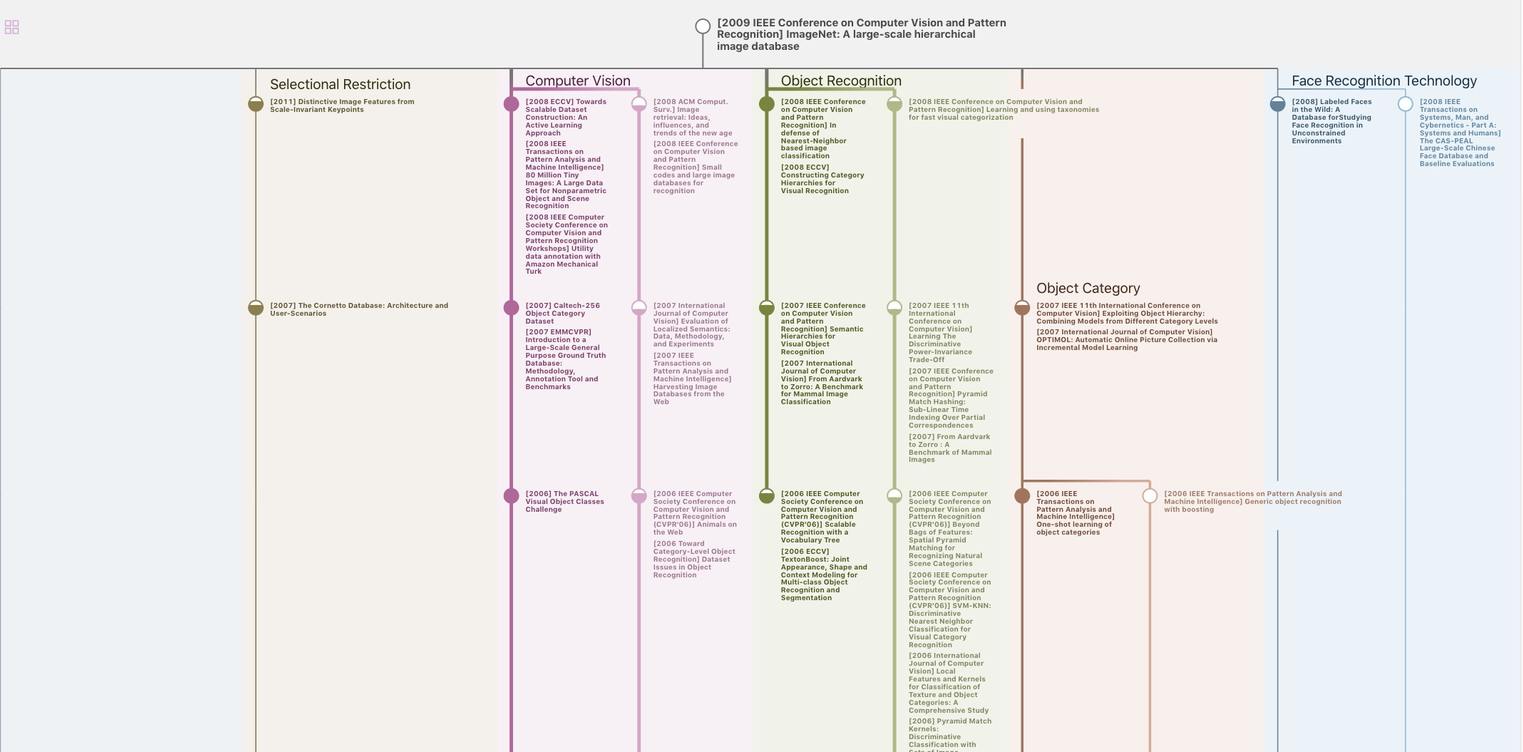
生成溯源树,研究论文发展脉络
Chat Paper
正在生成论文摘要