Modeling Long-range Dependencies and Epipolar Geometry for Multi-view Stereo
ACM TRANSACTIONS ON MULTIMEDIA COMPUTING COMMUNICATIONS AND APPLICATIONS(2023)
摘要
This article proposes a network, referred to as Multi-View Stereo TRansformer (MVSTR) for depth estimation from multi-view images. By modeling long-range dependencies and epipolar geometry, the proposedMVSTR is capable of extracting dense features with global context and 3D consistency, which are crucial for reliable matching in multi-view stereo (MVS). Specifically, to tackle the problem of the limited receptive field of existing CNN-based MVS methods, a global-context Transformer module is designed to establish intra-view long-range dependencies so that global contextual features of each view are obtained. In addition, to further enable features of each view to be 3D consistent, a 3D-consistency Transformer module with an epipolar feature sampler is built, where epipolar geometry is modeled to effectively facilitate cross-view interaction. Experimental results show that the proposed MVSTR achieves the best overall performance on the DTU dataset and demonstrates strong generalization on the Tanks & Temples benchmark dataset.
更多查看译文
关键词
Multi-view stereo, Transformer, feature matching, global context, long-range dependency, 3D consistency, epipolar geometry
AI 理解论文
溯源树
样例
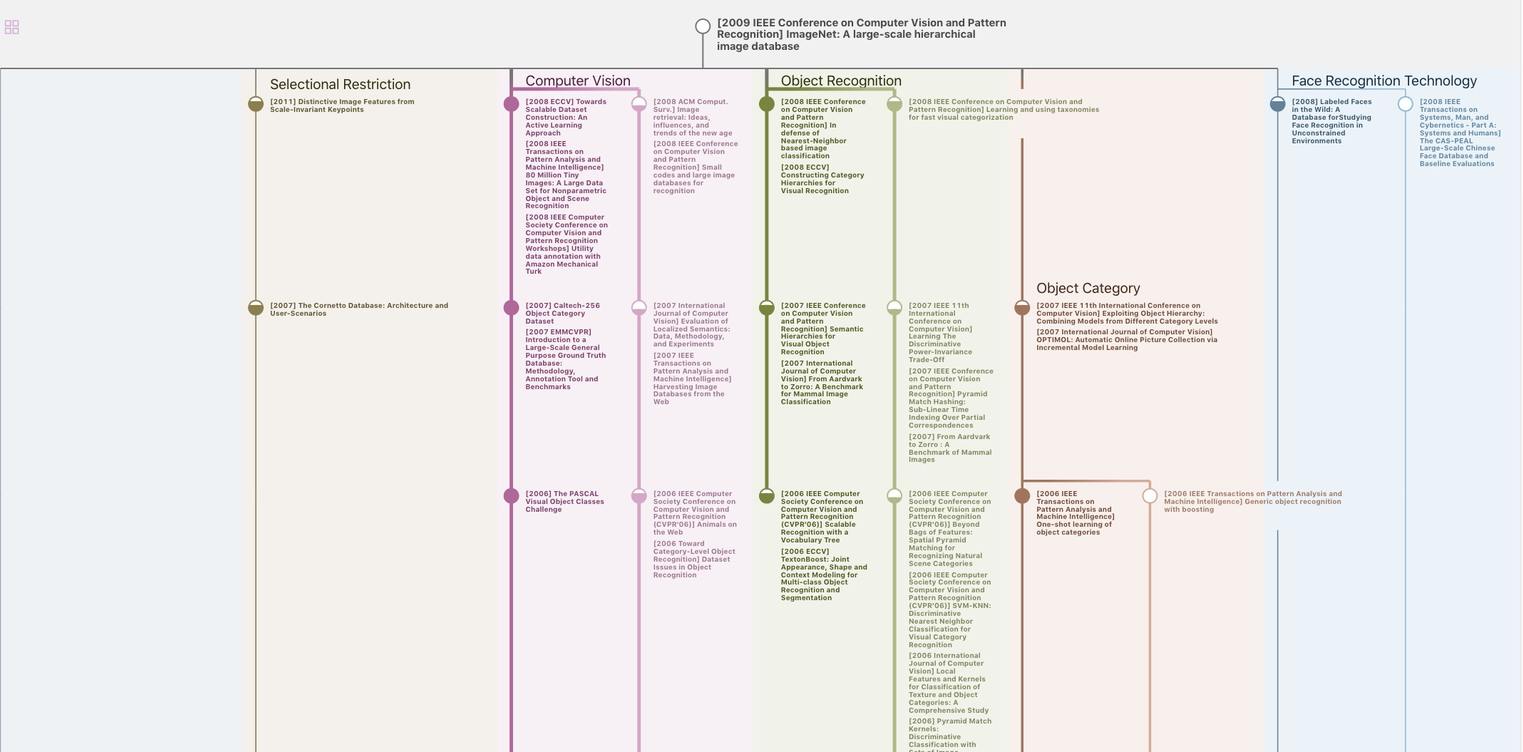
生成溯源树,研究论文发展脉络
Chat Paper
正在生成论文摘要