Text Image Super-Resolution Guided by Text Structure and Embedding Priors
ACM TRANSACTIONS ON MULTIMEDIA COMPUTING COMMUNICATIONS AND APPLICATIONS(2023)
摘要
We aim to super-resolve text images from unrecognizable low-resolution inputs. Existing super-resolution methods mainly learn a direct mapping from low-resolution to high-resolution images by exploring low-level features, which usually generate blurry outputs and suffer from severe structure distortion for text parts, especially when the resolution is quite low. Both the visual quality and the readability will suffer. To tackle these issues, we propose a new text super-resolution paradigm by recovering with understanding. Specifically, we extract a text-embedding prior and a text-structure prior from the upsampled image by learning to understand the text. The two priors with rich structure information and text-embedding information are then used as auxiliary information to recover the clear text structure. In addition, we introduce a text-feature loss to guide the training for better text recognizability. Extensive evaluations on both screen and scene text image datasets show that our method largely outperforms the state-of-the-art in both visual quality and recognition accuracy.
更多查看译文
关键词
text structure,image,super-resolution
AI 理解论文
溯源树
样例
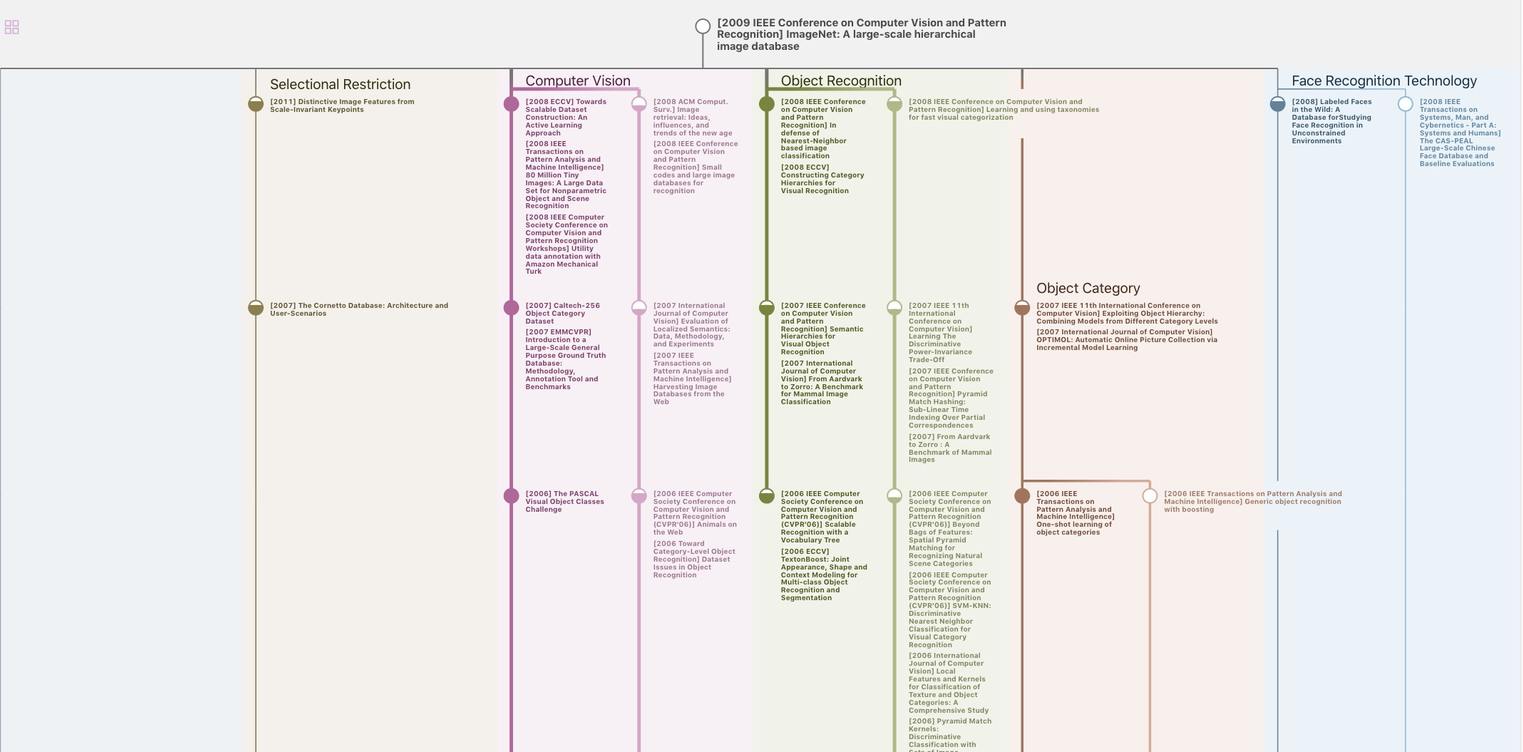
生成溯源树,研究论文发展脉络
Chat Paper
正在生成论文摘要