Towards improving decision tree induction by combining split evaluation measures
KNOWLEDGE-BASED SYSTEMS(2023)
摘要
Explainability is essential for users to effectively understand, trust, and manage powerful artificial intelligence solutions. Decision trees are one of the pioneer explanaible artificial intelligence algorithms widely used by experts in several contexts. C4.5 is a prominent decision tree that continues to serve as the base for subsequent improvements. One of main pillars on the decision tree induction is the split evaluation measure used for assesing the candidate splits. Usually, one single split evaluation measure is used during the induction procedure. However, combining multiple split evaluation measures into the induction procedure could improve the classification results. Our proposal aims to obtain better classification results by selecting the best candidate split taking advantage of the power of voting methods to aggregate the ranking issued by multiple split evaluation measures. Consequently, we performed a set of experiments to appraise the performance of our proposal on 114 databases. From these experiments, two novel split evaluation measures, proposed in this paper, were assessed against 11 individual and prominent state-of-the-art split evaluation measures. Our classification results show that our proposal outperforms the other state-of-the-art split evaluation measures.
更多查看译文
关键词
Supervised classification,Decision tree,Evaluation measure
AI 理解论文
溯源树
样例
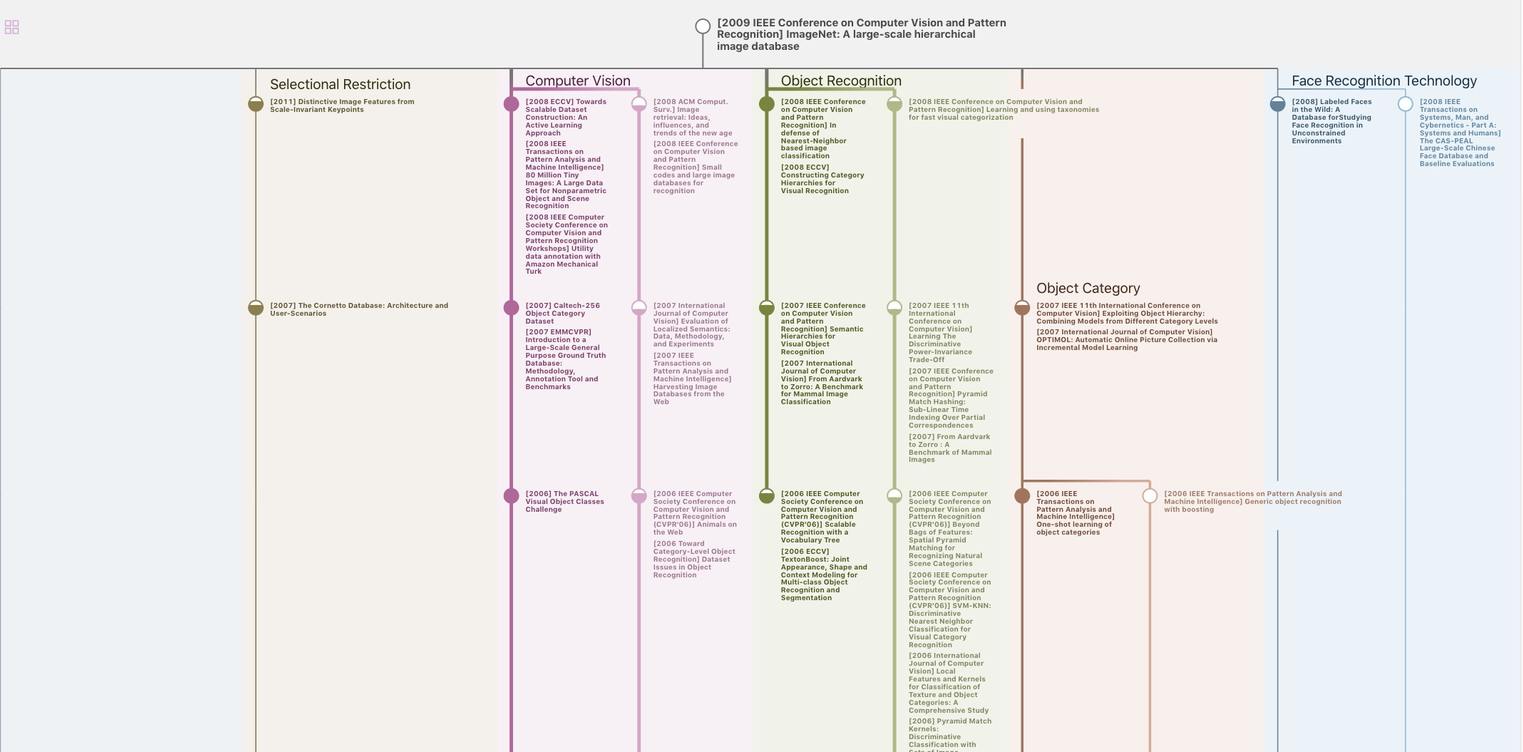
生成溯源树,研究论文发展脉络
Chat Paper
正在生成论文摘要