Learning logic programs by explaining their failures
Mach. Learn.(2023)
摘要
Scientists form hypotheses and experimentally test them. If a hypothesis fails (is refuted), scientists try to explain the failure to eliminate other hypotheses. The more precise the failure analysis the more hypotheses can be eliminated. Thus inspired, we introduce failure explanation techniques for inductive logic programming. Given a hypothesis represented as a logic program, we test it on examples. If a hypothesis fails, we explain the failure in terms of failing sub-programs. In case a positive example fails, we identify failing sub-programs at the granularity of literals. We introduce a failure explanation algorithm based on analysing branches of SLD-trees. We integrate a meta-interpreter based implementation of this algorithm with the test-stage of the Popper ILP system. We show that fine-grained failure analysis allows for learning fine-grained constraints on the hypothesis space. Our experimental results show that explaining failures can drastically reduce hypothesis space exploration and learning times.
更多查看译文
关键词
Relational learning,Inductive logic programming,Failure explanation
AI 理解论文
溯源树
样例
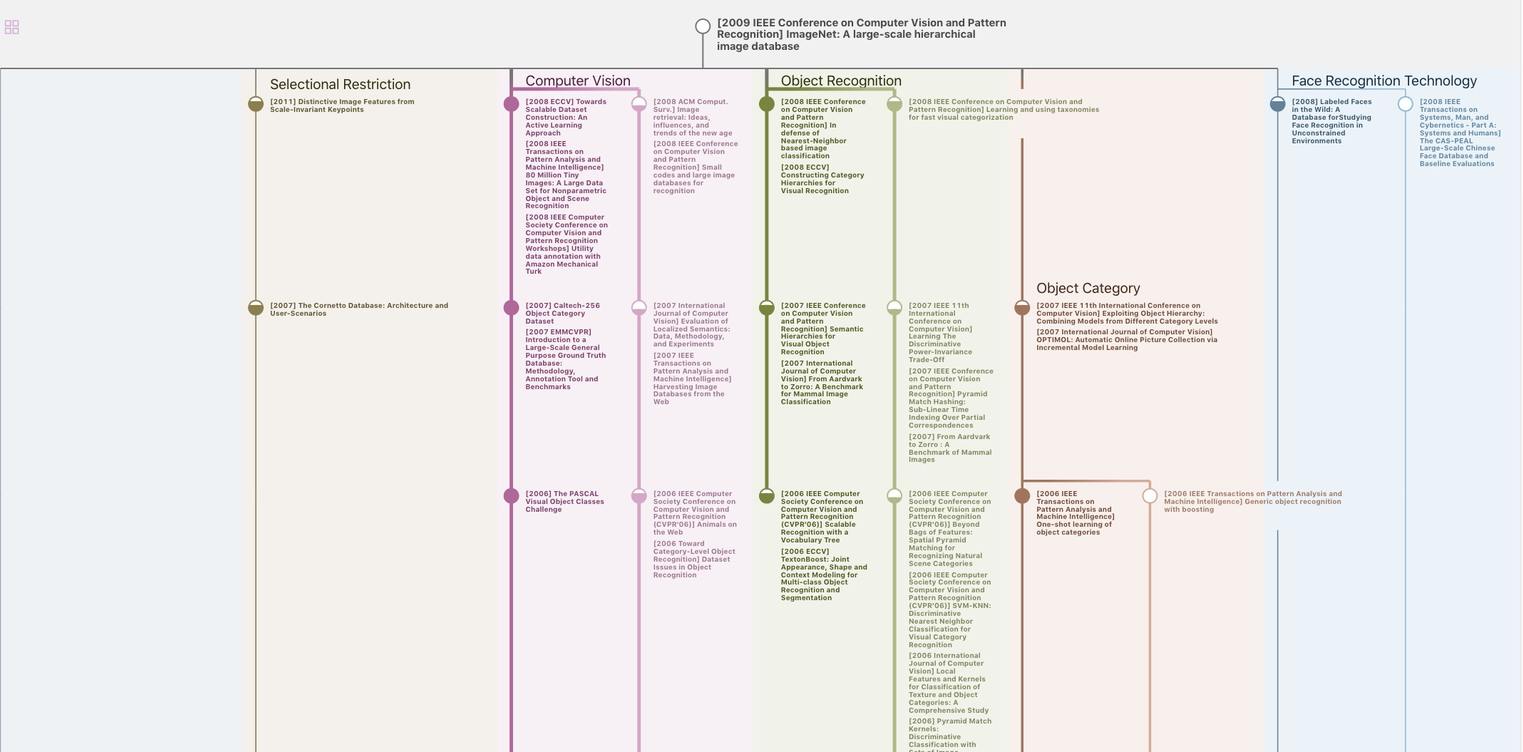
生成溯源树,研究论文发展脉络
Chat Paper
正在生成论文摘要