Insights into Heteroatom-Doped Graphene Supercapacitor Data through Manual Data Separation and Statistical Analysis
JOURNAL OF PHYSICAL CHEMISTRY C(2023)
摘要
The integration of data science with graphene-based supercapacitorshas emerged as a promising approach for optimizing their performance.Graphene-based electrodes exhibit unique pseudo-capacitive properties,but optimizing their supercapacitors requires balancing multiple variables,including electrode features, electrolyte composition, and operatingconditions. This is because the pseudo-capacitive effect can createdifferent charge-storage mechanisms via surface redox reactions duringcharging/discharging. In this paper, we discuss the use of statisticaldata analysis for gaining insights into the complex interplay betweenmaterial properties and electrochemical performance. We shed morelight on the analysis of large datasets generated from experimentsof heteroatom-doped graphene supercapacitors using data preprocessingtechniques, such as scattered interpolation-extrapolation andPearson correlation, instead of traditional machine learning models.We investigate the effects of various electrochemical features oncapacitive performance and the influence of heteroatom doping, suchas nitrogen, sulfur, and oxygen. Our analysis provides valuable insightsinto the essential electrochemical features and heteroatom dopingthat relate to capacitive-boosting performance, serving as a guidelinefor accelerating the development of energy storage, particularly forgraphene-based supercapacitors, in both experimental and machine learningaspects.
更多查看译文
关键词
graphene,heteroatom-doped
AI 理解论文
溯源树
样例
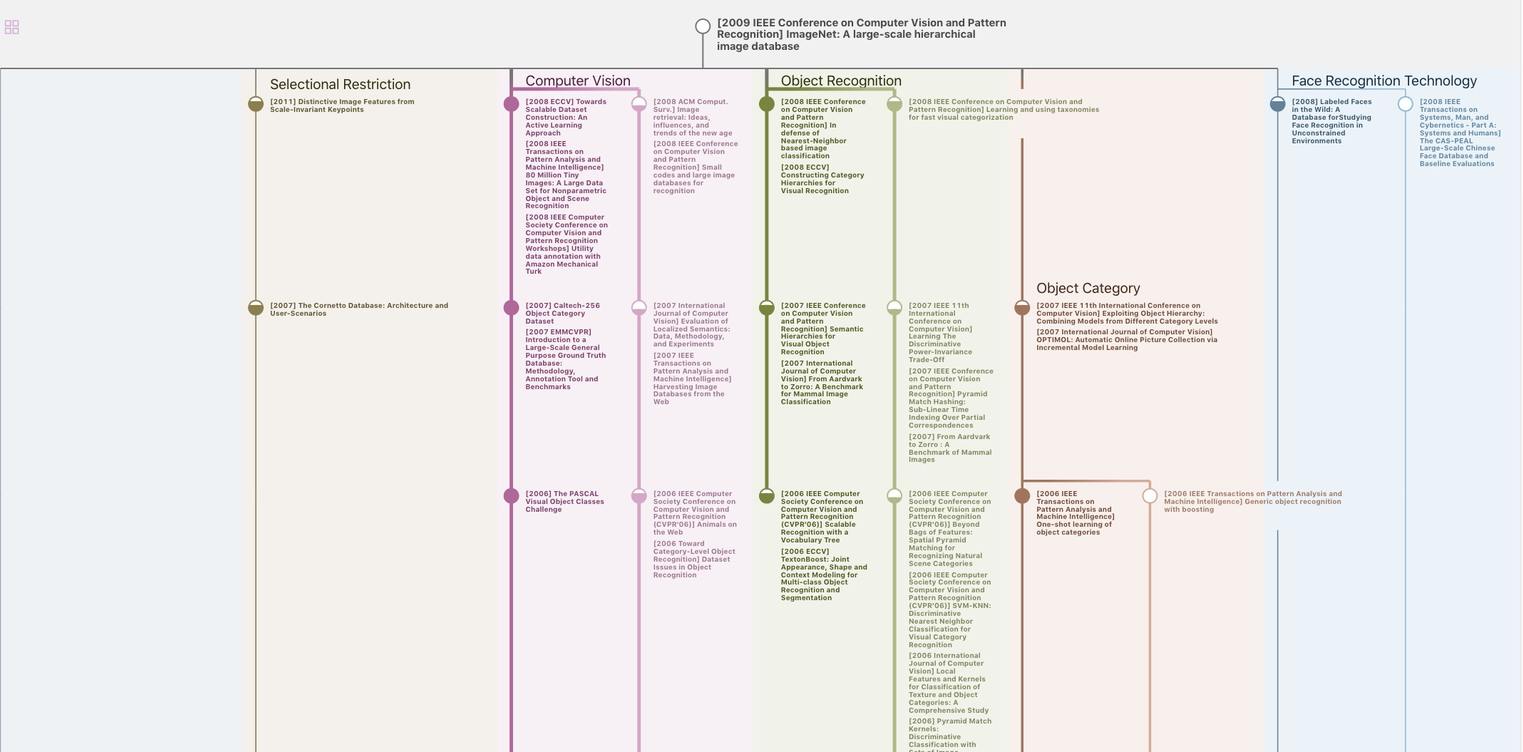
生成溯源树,研究论文发展脉络
Chat Paper
正在生成论文摘要