Swarm intelligence-based framework for accelerated and optimized assembly line design in the automotive industry
JOURNAL OF INTELLIGENT MANUFACTURING(2023)
摘要
This study proposes a dynamic simulation-based framework that utilizes swarm intelligence algorithms to optimize the design of hybrid assembly lines in the automotive industry. Two recent discrete versions of Whale Optimization Algorithm (named VNS-DWOA) and Gorilla Troops Optimizer (named DGTO) were developed to solve the assembly line balancing problem. The effectiveness of these algorithms was compared to six conventional meta-heuristics as well as the solution proposed by process design experts. The experimental results show that our methods outperform the conventional meta-heuristics and achieve comparable or better results than the experts’ solution. Particulary, VNS-DWOA, being the top performer, has consistently provided averagely remarkable enhancements of cycle time, ranging from 7% when compared to the process expert’s solution to 20% maximum improvement compared to all other methods. The findings of this study highlight the effectiveness of utilizing swarm intelligence algorithms and dynamic simulation-based frameworks as well as the potential benefits of implementing these digital methods in industrial settings, as they can significantly accelerate and enhance the optimization of assembly line design particularly and reduce time to market generally.
更多查看译文
关键词
Industry 4, 0, Assembly line balancing, Swarm intelligence, Discrete metaheuristics, Combinatorial optimization, Distributed algorithms
AI 理解论文
溯源树
样例
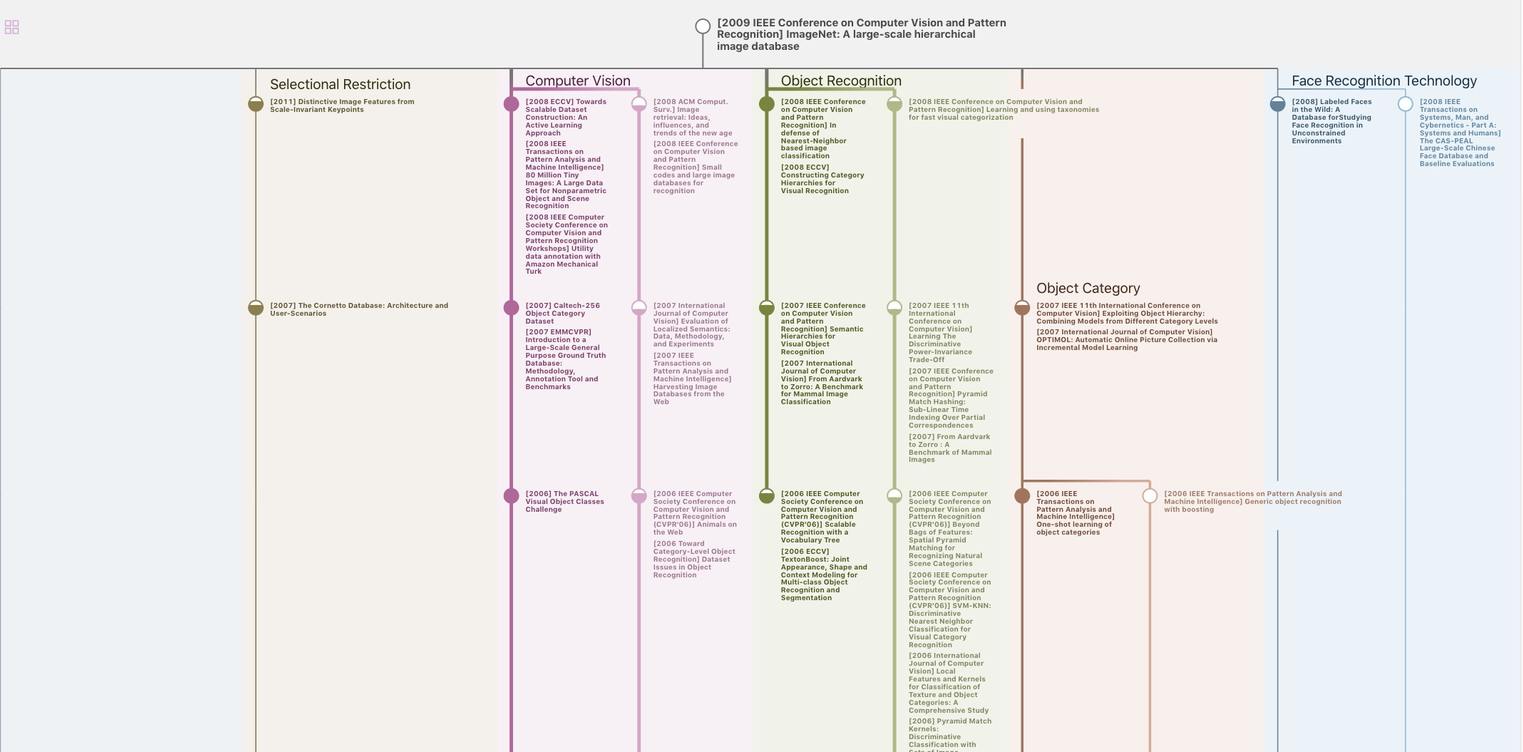
生成溯源树,研究论文发展脉络
Chat Paper
正在生成论文摘要