Novel SVM-based classification approaches for evaluating pancreatic carcinoma
ANNALS OF MATHEMATICS AND ARTIFICIAL INTELLIGENCE(2023)
摘要
In this paper, we develop two SVM-based classifiers named stable nested one-class support vector machines (SN-1SVMs) and decoupled margin-moment based SVMs (DMMB-SVMs), to predict the specific type of pancreatic carcinoma using quantitative histopathological signatures of images. For each patient, the diagnosis can produce hundreds of images, which can be used to classify the pancreatic tissues into three classes: chronic pancreatitis, intraductal papillary mucinous neoplasms, and pancreatic carcinoma. The proposed two approaches tackle the classification problems from two different perspectives: the SN-1SVM treats each image as a classification point in a nested fashion to predict malignancy of the tissues, while the DMMB-SVM treats each patient as a classification point by assembling information across images. One attractive feature of the DMMB-SVM is that, in addition to utilizing the mean information, it also takes into account the covariance of features extracted from images for each patient. We conduct numerical experiments to evaluate and compare performance of the two methods. It is observed that the SN-1SVM can take advantage of the data structure more effectively, while the DMMB-SVM demonstrates better computational efficiency and classification accuracy. To further improve interpretability of the final classifier, we also consider the ℓ _1 -norm in the DMMB-SVM to handle feature selection.
更多查看译文
关键词
Pancreatic carcinoma, Karyometry data, Support vector machines, Nested classification, Covariance
AI 理解论文
溯源树
样例
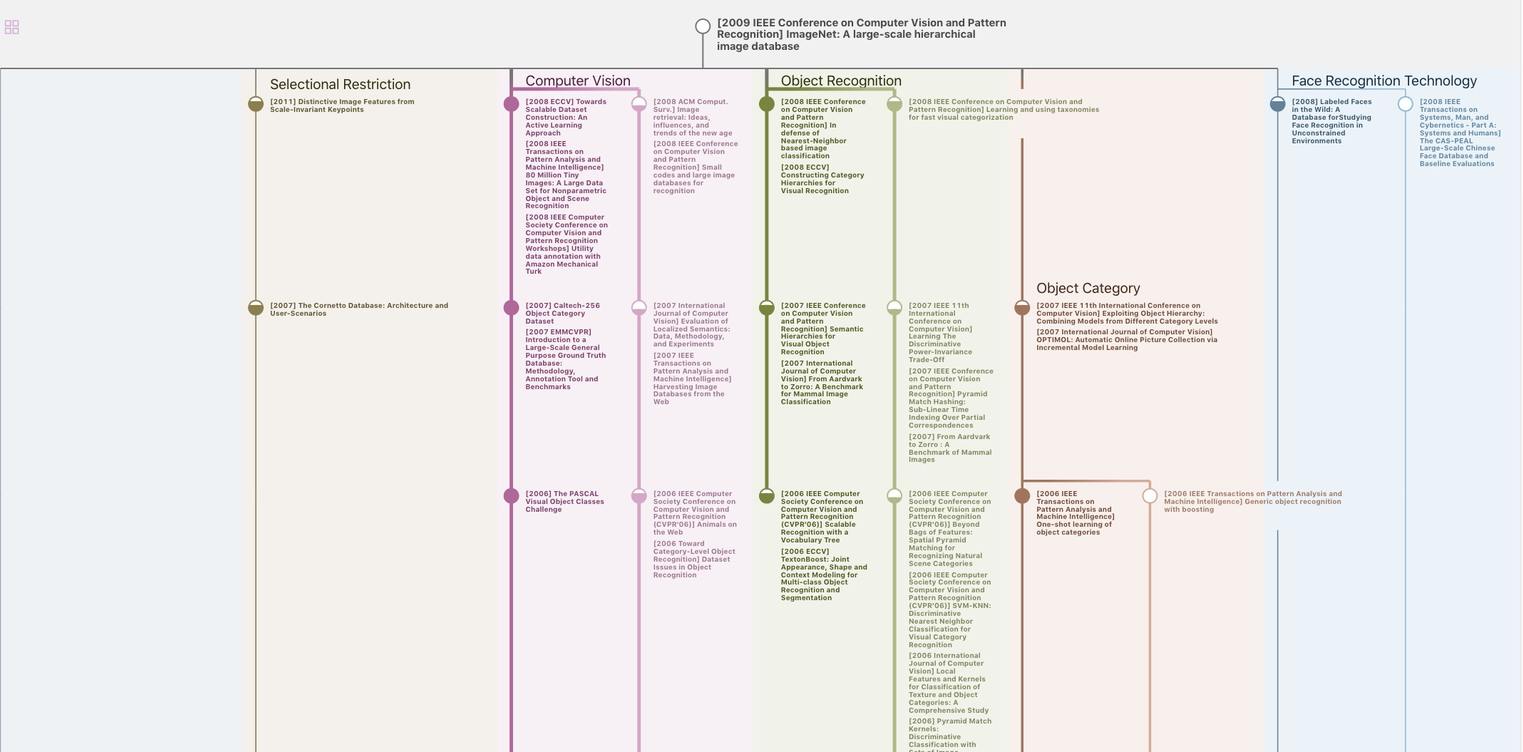
生成溯源树,研究论文发展脉络
Chat Paper
正在生成论文摘要