CrowdDC: Ranking From Crowdsourced Paired Comparison With Divide-and-Conquer
IEEE TRANSACTIONS ON COMPUTATIONAL SOCIAL SYSTEMS(2023)
摘要
This article introduces a novel algorithm, named "CrowdDC", that aims to solve the issue of ranking large datasets based on subjective factors using crowdsourced paired comparisons. In traditional paired comparison analysis, dealing with a sizeable dataset can become impractical as the number of comparisons required increases quadratically. To address this problem, CrowdDC is designed as a divide-and-conquer algorithm that partitions the dataset into smaller subsets and compares them independently. The results of these comparisons are then combined to generate an overall ranking. Simulation results showed that, when ranking more than 100 items, CrowdDC succeeded in reducing the number of requisite tasks by 45%-75%, while concurrently maintaining an accuracy range of 90%-95% relative to the baseline approach.
更多查看译文
关键词
Crowdsourcing,divide-and-conquer,paired comparison,ranking
AI 理解论文
溯源树
样例
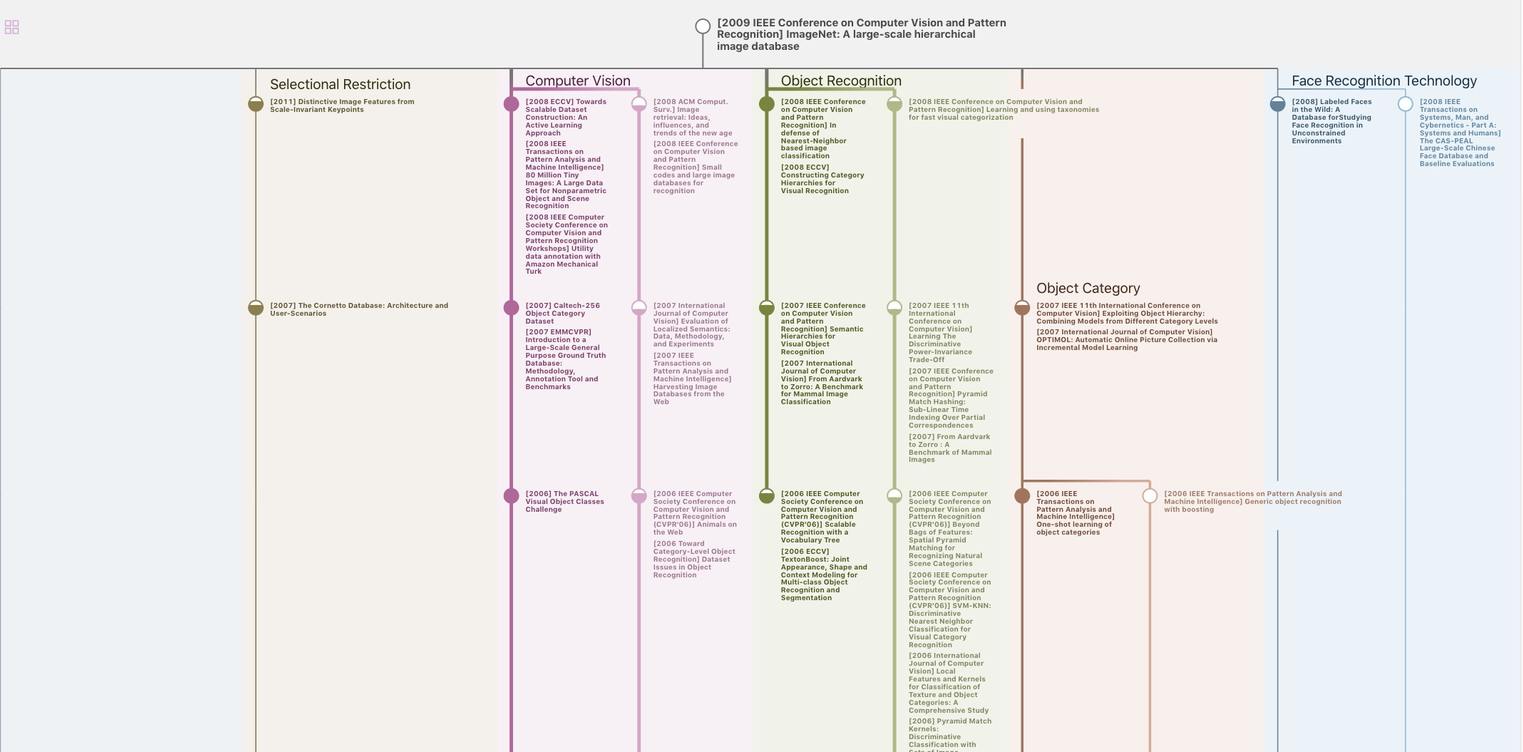
生成溯源树,研究论文发展脉络
Chat Paper
正在生成论文摘要