A Meta-Analysis of State-of-the-Art Automated Fake News Detection Methods
IEEE TRANSACTIONS ON COMPUTATIONAL SOCIAL SYSTEMS(2023)
摘要
Recently, various artificial intelligence (AI)-based methods have been proposed to support humans in detecting disinformation and fake news. The goal of this article is to provide a meta-analysis, and formally evaluate, compare, and benchmark various classes of fake news detection approaches. To this end, the following paper performs a comprehensive analysis of the performance-related results of different models using a range of benchmark datasets. The performed and disclosed meta-analysis compares the statistical significance of differences in a range of performance metrics, including precision, F1-score, recall, and balanced accuracy (BACC). The utilized approach features the 5 x 2 cross-validation methodology. The models undergoing the formal evaluation constitute state-of-the-art (SOTA) solutions meeting acceptance criteria. The evaluated approaches draw from the most recent advancements in natural language processing (NLP). The outcome of this work is the formal benchmarking and meta-analysis of fake news detection methods that can be further utilized by the research community, but more importantly by the practitioners and decision-makers that counter fake news on a daily basis, e.g., in press agencies, homeland security agencies, fact-checkers, and so on. This work is the natural extension of the authors' previous systematic analysis of fake news detection methods and authors' own fake news detection methods based on machine learning (ML)/artificial intelligence (AI) techniques.
更多查看译文
关键词
Detection,fake news,natural language processing (NLP),neural networks,security,text classification
AI 理解论文
溯源树
样例
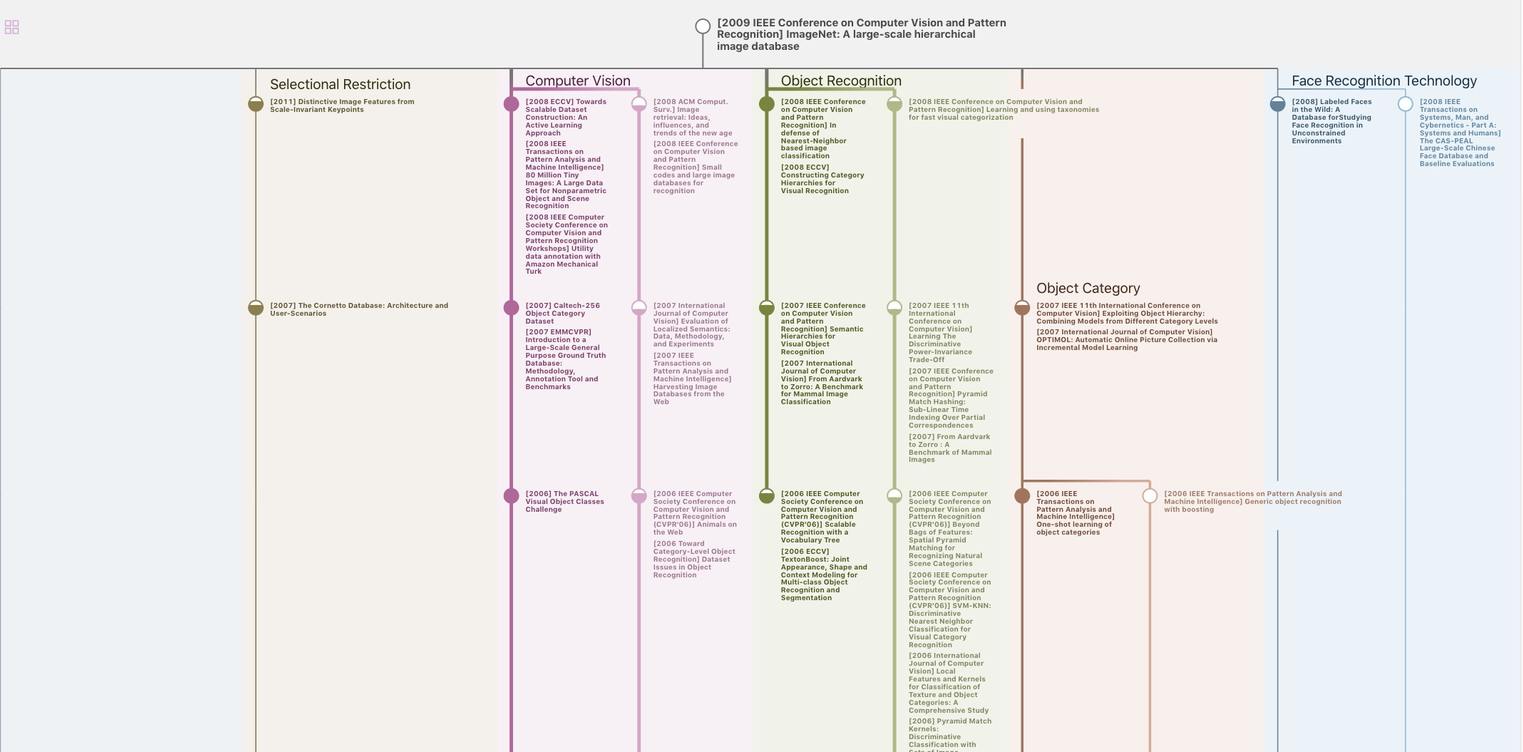
生成溯源树,研究论文发展脉络
Chat Paper
正在生成论文摘要