Active pairwise distance learning for efficient labeling of large datasets by human experts
APPLIED INTELLIGENCE(2023)
摘要
In many machine learning applications, the labeling of datasets is done by human experts, which is usually time-consuming in cases of large data sets. This raises the need for methods to make optimal use of the human expert by selecting model instances for which the expert opinion is of most added value. This paper introduces the problem of active pairwise distance learning (APDL), where the goal is to actively learn the pairwise distances between all instances. Any distance function can be used, which means that APDL techniques can e.g., be used to determine likeness between faces or similarities between users for recommender systems. Starting with an unlabeled dataset, each round an expert determines the distance between one pair of instances. Thus, there is an important choice to make each round: ‘Which combination of instances is presented to the expert?’ The objective is to accurately predict all pairwise distances, while minimizing the usage of the expert. In this research, we establish upper and lower bound approximations (including an update rule) for the pairwise distances and evaluate many domain-independent query strategies. The observations from the experiments are therefore general, and the selection strategies are ideal candidates to function as baseline in future research. We show that using the criterion max degree consistently ranks amongst the best strategies. By using this criterion, the pairwise distances of a new dataset can be labeled much more efficiently.
更多查看译文
关键词
Active learning, Labeling, Pairwise distance, Optimal strategy, Human expert
AI 理解论文
溯源树
样例
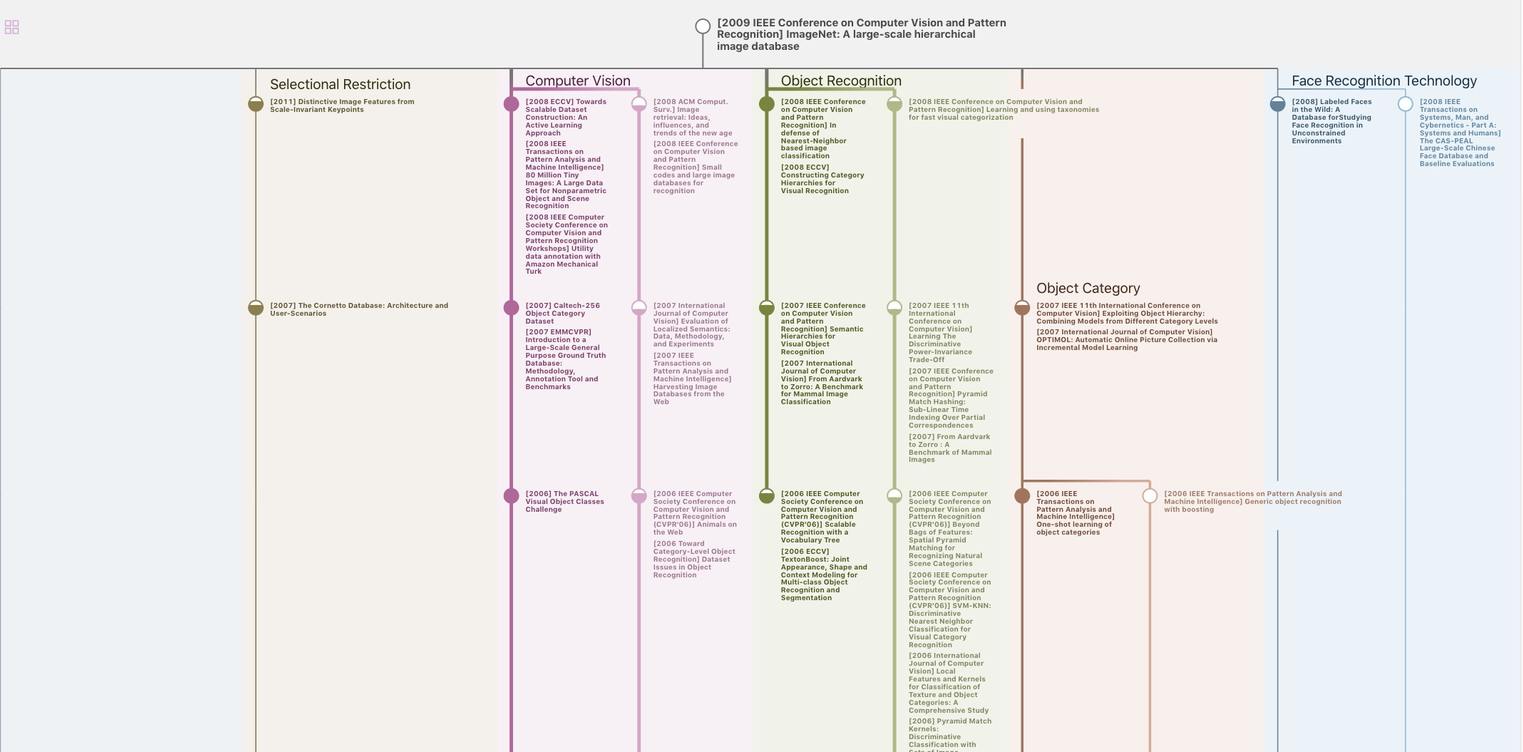
生成溯源树,研究论文发展脉络
Chat Paper
正在生成论文摘要