Auto-Regressive Integrated Moving Average Threshold Influence Techniques for Stock Data Analysis
INTERNATIONAL JOURNAL OF ADVANCED COMPUTER SCIENCE AND APPLICATIONS(2023)
摘要
study focuses on predicting and estimating possible stock assets in a favorable real-time scenario for financial markets without the involvement of outside brokers about broadcast-based trading using various performance factors and data metrics. Sample data from the Y-finance sector was assembled using API-based data series and was quite accurate and precise. Prestigious machine learning algorithmic performances for both classification and regression complexities intensify this assumption. The fallibility of stock movement leads to the production of noise and vulnerability that relate to decision-making. In earlier research investigations, fewer performance metrics were used. In this study, Dickey-Fuller testing scenarios were combined with time series volatility forecasting and the Long Short-Term Memory algorithm, which was used in a futuristic recurrent neural network setting to predict future closing prices for large businesses on the stock market. In order to analyze the root mean squared error, mean squared error, mean absolute percentage error, mean deviation, and mean absolute error, this study combined LSTM methods with ARIMA. With fewer hardware resources, the experimental scenarios were framed, and test case simulations carried out.
更多查看译文
关键词
Dickey-Fuller test case (DF-TC),recurrent neural network (RNN),root mean square error (RMSE),long short-term memory (LSTM),machine learning (ML),auto-regressive integrated moving average (ARIMA)
AI 理解论文
溯源树
样例
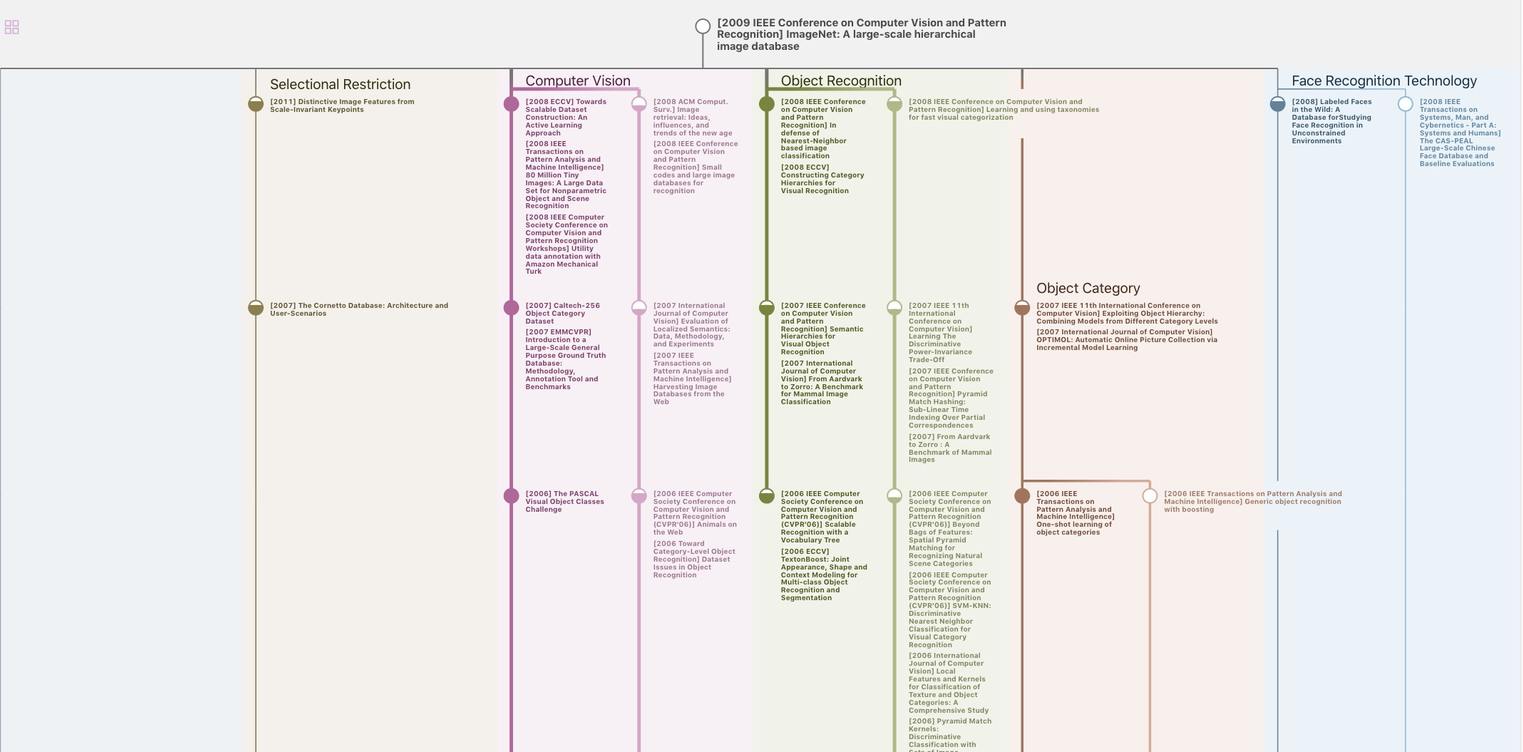
生成溯源树,研究论文发展脉络
Chat Paper
正在生成论文摘要