A pruning extreme learning machine with L2,1/2 regularization for multi-dimensional output problems
INTERNATIONAL JOURNAL OF MACHINE LEARNING AND CYBERNETICS(2024)
摘要
As a fast algorithm for training single-hidden layer feedforward neural networks, extreme learning machine (ELM) has been successfully applied to various classification and regression problems. In recent years, regularization techniques have been widely used in ELM to improve its stability, sparsity and generalization capability. In order to determine the appropriate number of hidden layer nodes, the ELM regularized by l(1/2) quasi-norm (L-1/2-ELM) was developed to prune the redundant hidden nodes. However, in multi-dimensional output tasks, L-1/2-ELM only removes redundant weights of hidden nodes but cannot guarantee the sparsity at the node level. In this paper, we present the L-2,L-1/2-ELM, which is regularized by L-2,L-1/2 quasi-norm to achieve sparsity in multi-dimensional output problems. With the generalization of L-1/2 regularization to L-2,L-1/2 regularization, L-2,L-1/2-ELM can prune the corresponding hidden nodes by setting some rows of the output weight matrix to zero. Since the proximal operator corresponding to L-2,L-1/2 regularization has a closed-form solution, the powerful alternating direction method of multipliers (ADMM) is employed to achieve a fast solution of L-2,L-1/2-ELM. Furthermore, to face the challenge of distributed computing, we extend L-2,L-1/2-ELM to its distributed version, namely DL2,1/2-ELM. DL2,1/2-ELM is solved by the consensus ADMM algorithm. Experiments on multi-classification and multi-target regression datasets demonstrate that our proposed algorithms can achieve competitive sparsity without compromising accuracy.
更多查看译文
关键词
Extreme learning machine,L-2,L-1/2 regularization,Sparsity,Multi-dimensional output,Alternating direction method of multipliers,Distributed algorithm
AI 理解论文
溯源树
样例
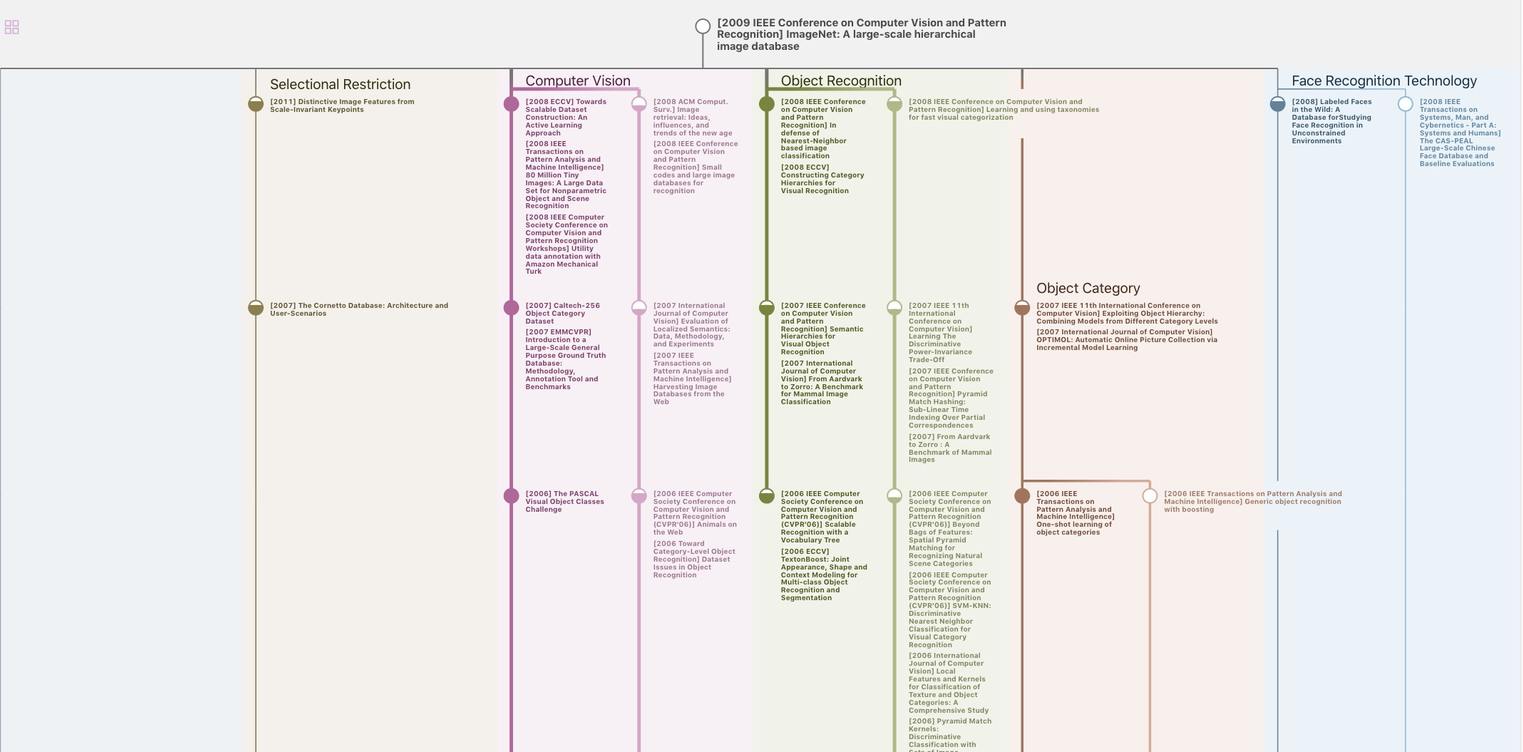
生成溯源树,研究论文发展脉络
Chat Paper
正在生成论文摘要