Kernel Optimization for Reducing Core Vector Machine Classification Error
NEURAL PROCESSING LETTERS(2023)
摘要
Core vector machine (CVM) is considered as a popular machine learning technique, mostly used for classification and regression. Its generalized form can be either applied to kernel methods or used with linear and nonlinear kernels. As a classifier, both the kernel function and its parameters can significantly affect the accuracy of CVM classification. In this paper, a metaheuristic kernel core vector machine (MKCVM) was applied by using comprehensive learning particle swarm optimization. It was done through an innovative parameter selection technique in order to obtain a proper kernel function based on a nonlinear combination of standard kernel functions. The CVM true classification rate and geometric mean accuracy were adopted as the fitness criteria of the proposed approach, to be maximized. In addition, some benchmark datasets were used to evaluate the proposed approach. Based on the experimental results, the proposed method could decrease CVM classification error and increase its geometric mean accuracy. This has a comparative performance rather than support vector machine with lower memory and computational complexity. Moreover, MKCVM showed the acceptable capability to deal with imbalanced data. So, it had a good ability for handling binary and multiclass data classification.
更多查看译文
关键词
Core vector machine, Kernel, Classification, Comprehensive learning particle swarm optimization, Geometric mean accuracy, Imbalanced data
AI 理解论文
溯源树
样例
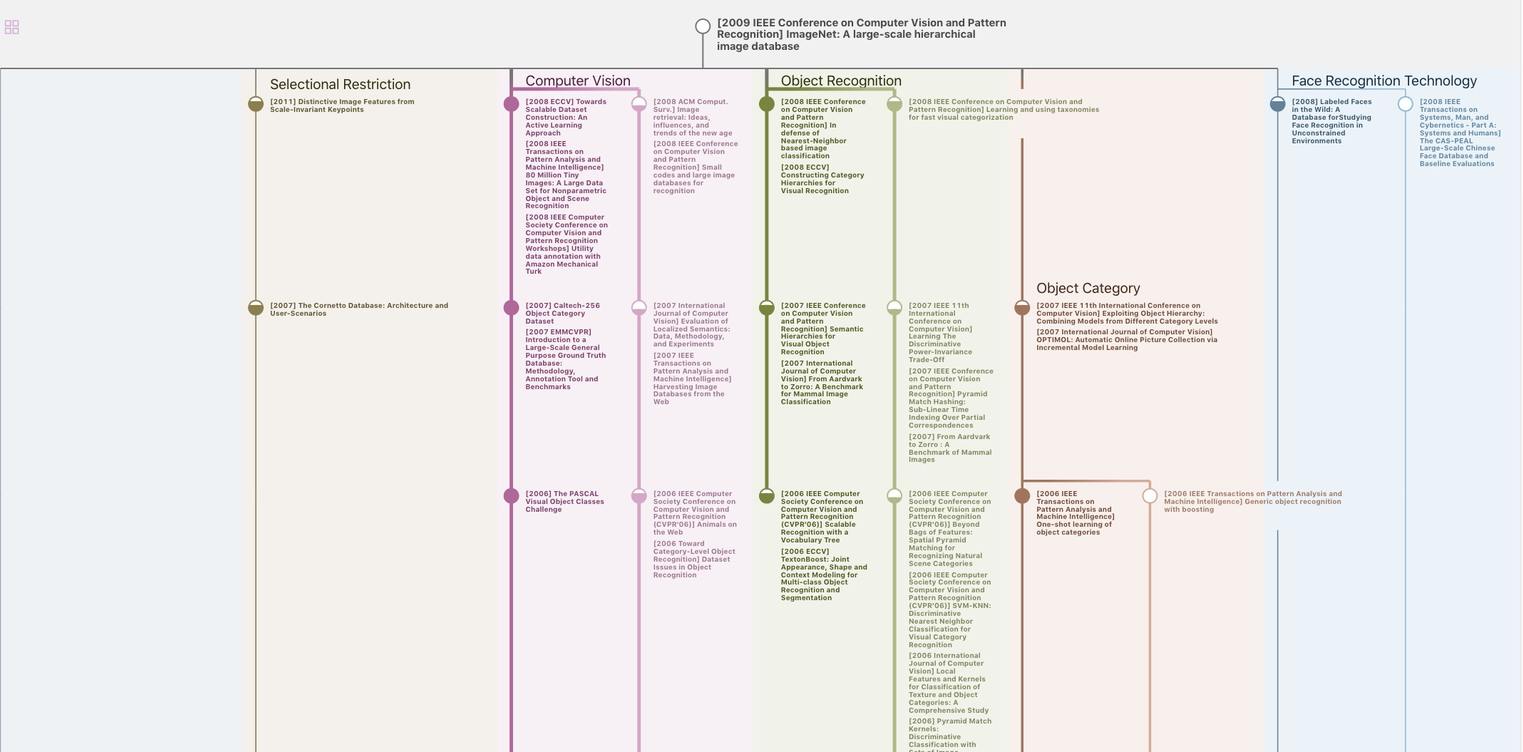
生成溯源树,研究论文发展脉络
Chat Paper
正在生成论文摘要