Empowering Electrochemical Biosensors with AI: Overcoming Interference for Precise Dopamine Detection in Complex Samples
ADVANCED INTELLIGENT SYSTEMS(2023)
摘要
Two significant issues in biosensors that can't be solved by conventional analytical methods are selectivity among likely biological interfering molecules and background noise in clinical samples. The application of embedded machine learning in the removal of background interference, which is extremely common in complex matrix solutions such as cerebrospinal fluid, is still unexplored. The implementation of machine learning into devices and sensors can enhance their reliability to discriminate responses. In addition, implementing these models into portable devices can further improve the usage of point-of-care devices, continuous monitoring, and viral mutation assessments. Herein, the requirements to implement an embedded AI model (TinyML) into low-power portable systems and its use in the electrochemical field are presented. The application of TinyML to discriminate between interference of uric acid and ascorbic acid, both well-known abundant electrochemically active species, in neurotransmitter detection, is explored, reaching overall accuracy of 98.1% for 32-bit float point unit and 96.01% after 8-bit quantization, with the usage of 4.55% of the custom-made potentiostat memory. The model can be improved simply by having the trade-off between memory and accuracy. The research suggests that TinyML can be a key component in future medical devices, allowing data processing in real time and with increasing reliability.
更多查看译文
关键词
electrochemical sensors, internet of medical things, machine learning, neurotransmitters TinyML
AI 理解论文
溯源树
样例
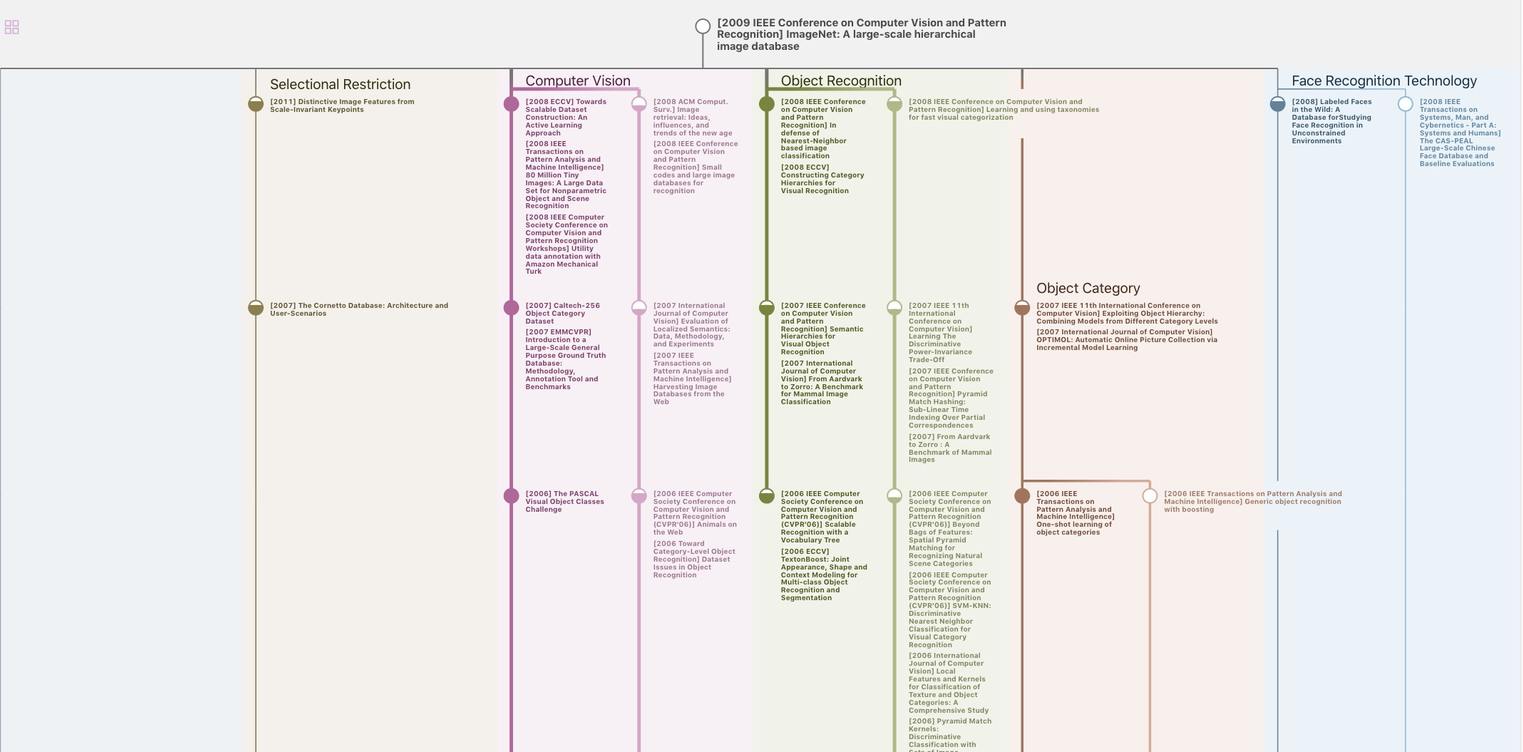
生成溯源树,研究论文发展脉络
Chat Paper
正在生成论文摘要