Evaluation of convolutional neural network for fast extreme ultraviolet lithography simulation using imec 3 nm node mask patterns
JOURNAL OF MICRO-NANOPATTERNING MATERIALS AND METROLOGY-JM3(2023)
摘要
Background: Mask 3D (M3D) effects distort diffraction amplitudes from extreme ultraviolet masks. In our previous work, we developed a convolutional neural network (CNN) that very quickly predicted the distorted diffraction amplitudes from input mask patterns. The mask patterns were restricted to Manhattan patterns.Aim: We verify the potentials and the limitations of CNN using imec 3 nm node (iN3) mask patterns.Approach: We apply the same CNN architecture in the previous work to mask patterns, which mimic iN3 logic metal or via layers. In addition, to study more general mask patterns, we apply the architecture to iN3 metal/via patterns with optical proximity correction (OPC) and curvilinear via patterns. In total, we train five different CNNs: metal patterns w/wo OPC, via patterns w/wo OPC, and curvilinear via patterns. After the training, we validate each CNN using validation data with the above five different characteristics.Results: When we use the training and validation data with the same characteristics, the validation loss becomes very small. Our CNN architecture is flexible enough to be applied to iN3 metal and via layers. The architecture has the capability to recognize curvilinear mask patterns. On the other hand, using the training and validation data with different characteristics will lead to large validation loss. The selection of training data is very important for obtaining high accuracy. We examine the impact of M3D effects on iN3 metal layers. A large difference is observed in the tip to tip (T2T) critical dimension calculated by the thin mask model and thick mask model. This is due to the mask shadowing effect at T2T slits.Conclusions: The selection of training data is very important for obtaining high accuracy. Our test results suggest that layer specific CNN could be constructed, but further development of CNN architecture could be required.
更多查看译文
关键词
convolutional neural network,neural network
AI 理解论文
溯源树
样例
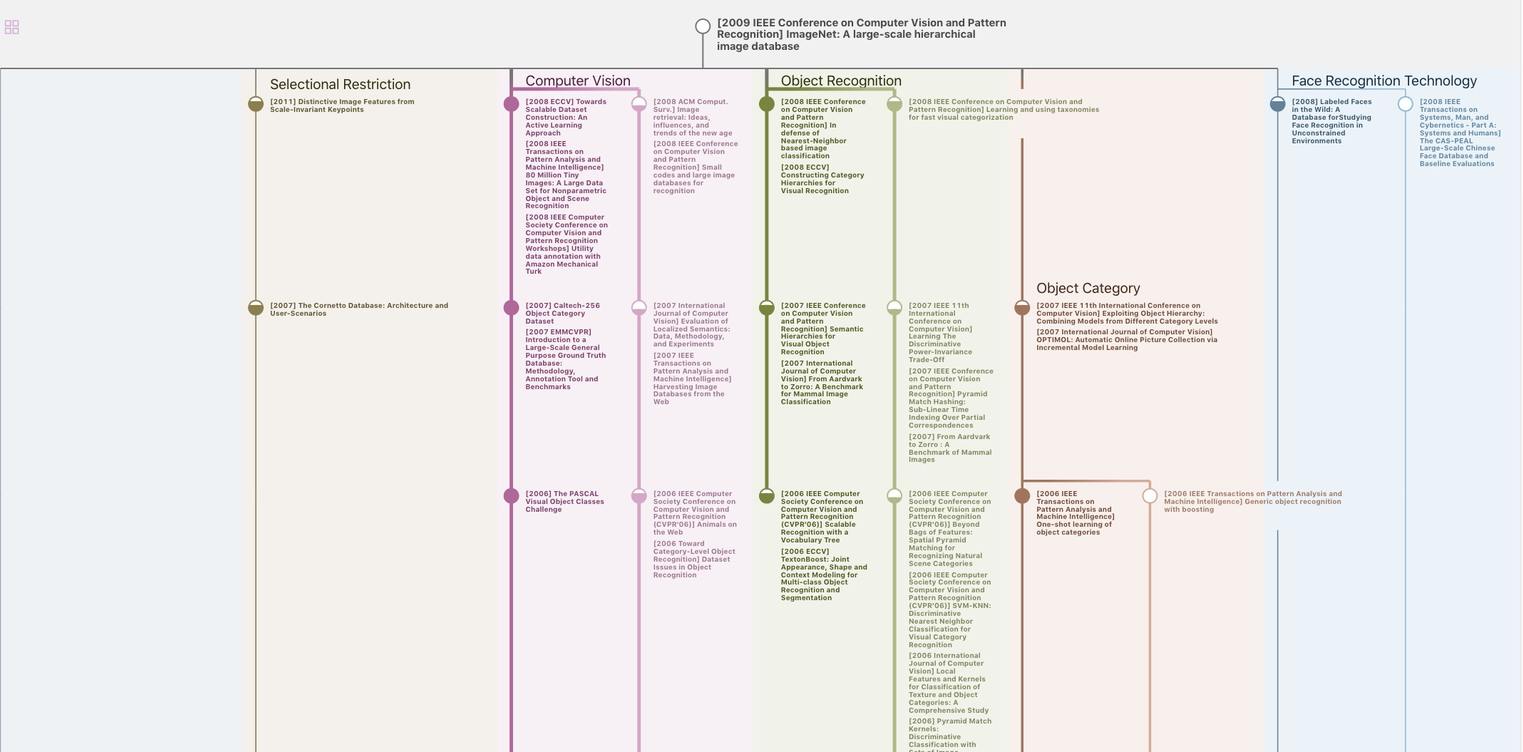
生成溯源树,研究论文发展脉络
Chat Paper
正在生成论文摘要