Comparing a Bayesian Classifier for Acoustic Signals to Machine Learning Classifiers
SIGNAL PROCESSING, SENSOR/INFORMATION FUSION, AND TARGET RECOGNITION XXXII(2023)
摘要
Acoustic signals in complex real-world environments are randomized by processes such as multipath reflections, surface scattering, and volume scattering by turbulence and vegetation. Traditional machine learning classification algorithms require large datasets with substantial variation to mitigate the challenge posed by randomized signals. In this paper, a Bayesian classifier is introduced that incorporates the underlying physics of the scattered signal into a realistic likelihood distribution for the random signal variations. Performance of the Bayesian classifier is compared to machine learning classification algorithms based on two convolutional neural networks (CNN), one for images and one for sound. Given the varying architectures of the three classification algorithms each approach requires a different feature set. The image-based CNN uses a spectrogram as the input, the soundbased CNN uses the normalized waveform, and the Bayesian classifier is developed on features given by the power in the one-third octave bands. For the comparison, we employ the acoustic seismic classification identification data set (ACIDS) and the environmental sound classification data set (ESC-50). To account for the small size of the considered data sets, we use transfer learning for the machine learning classification algorithms. For the ACIDS and ESC-50 data sets, we show that the Bayesian classifier method outperforms the image-based CNN and the sound-based CNN when measured by accuracy on a test set of data. Unlike the physics-based Bayesian classifier, the machine learning algorithms do not take advantage of the underlying physics of the acoustic signal, which impacts the classification accuracy of traditional machine learning approaches.
更多查看译文
关键词
Bayesian reasoning,classification,machine learning,acoustics
AI 理解论文
溯源树
样例
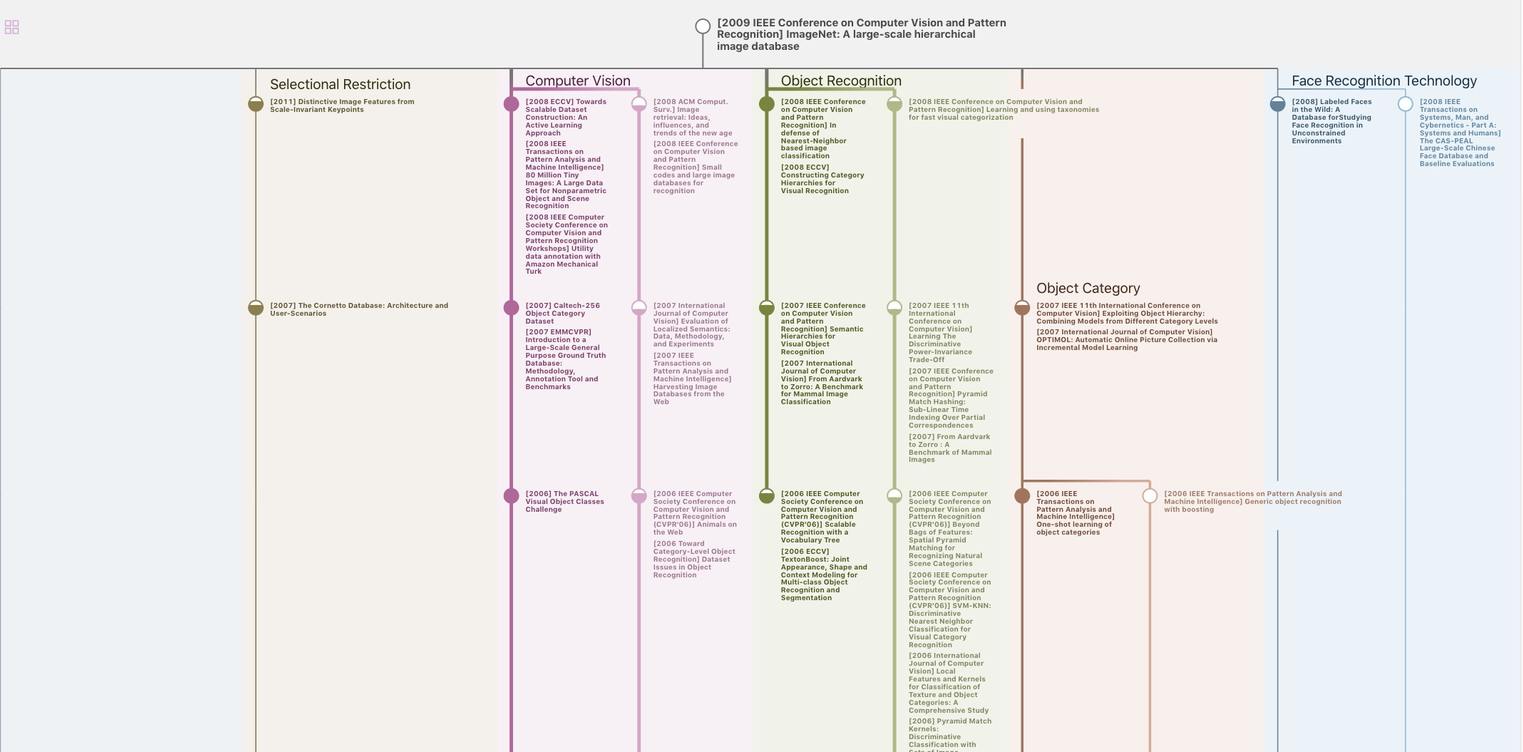
生成溯源树,研究论文发展脉络
Chat Paper
正在生成论文摘要