Fairness of linear regression in decision making
INTERNATIONAL JOURNAL OF DATA SCIENCE AND ANALYTICS(2023)
摘要
Ranking systems conceived on historical data are central to our societies. Given a set of applicants and the information as to whether a past-applicant should have been selected or not, the task of fairly ranking the applicants (either by humans or by computers) is critical to the success of any institution. These tasks are typically carried out using regression methods, and considering the impact of these selection processes on our lives, it is natural to expect various fairness guarantees. In this article, we assume that affirmative action is enforced and that the number of candidates to admit from each protected group is predetermined. We demonstrate that even with this safety-net, classical linear regression methods may increase discrimination in the selection process, reinforcing implicit biases against minorities, in particular by poorly ranking the top minority applicants. We show that this phenomenon is intrinsic to linear regression methods and may happen even if the sensitive attribute is explicitly part of the input, or if a linear regression is computed on each minority group individually. We show that to better rank applicants it might be needed to adapt the choice of the regression methods (linear, polynomial, etc.) to each minority group individually.
更多查看译文
关键词
fairness,decision making,linear regression
AI 理解论文
溯源树
样例
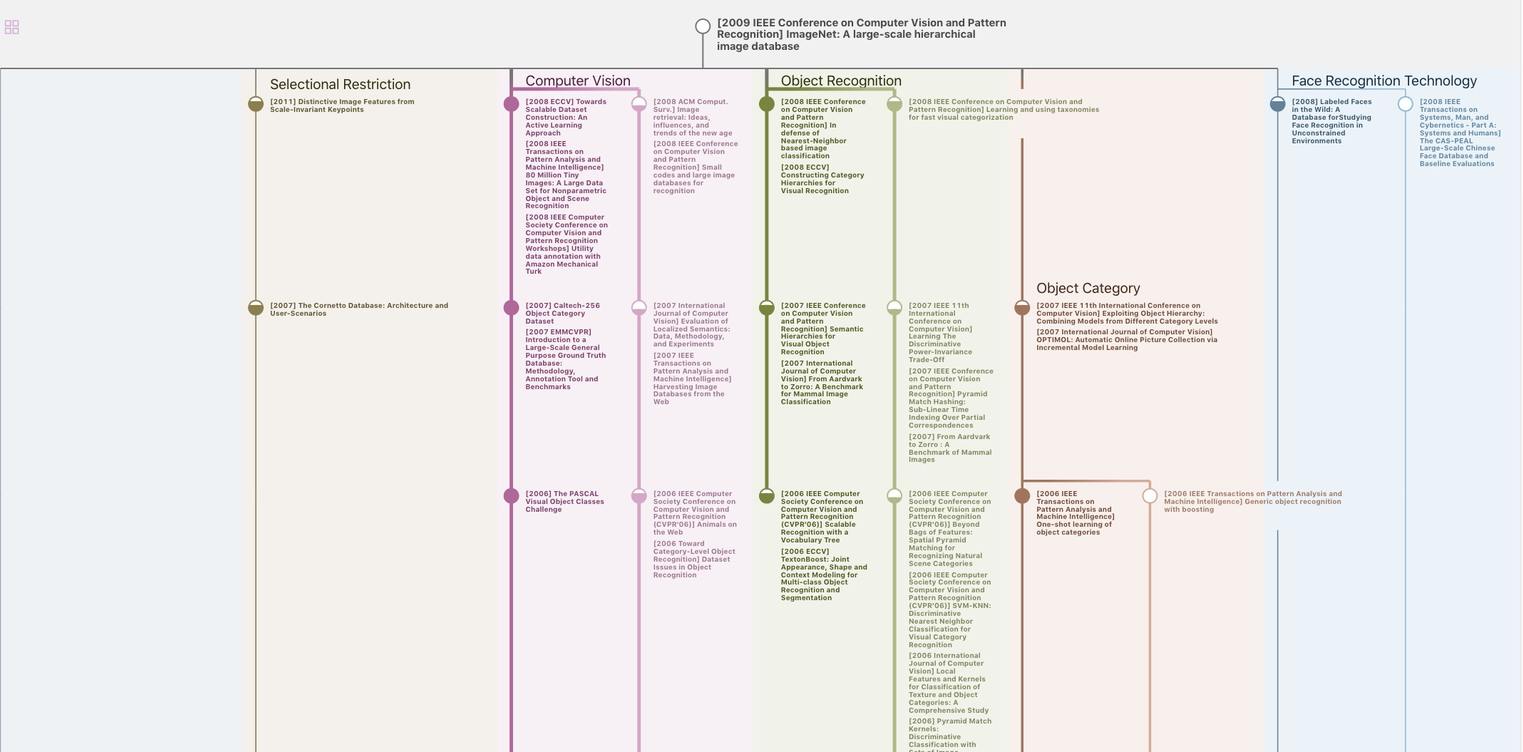
生成溯源树,研究论文发展脉络
Chat Paper
正在生成论文摘要