Improving Energy Consumption and Order Tardiness in Picker-to-Part Warehouses with Electric Forklifts: A Comparison of Four Evolutionary Algorithms
SUSTAINABILITY(2023)
摘要
Improving energy consumption (EC) and order tardiness (OT) for a warehouse picker-to-parts system is a challenging task since these two objectives are interrelated in a complex way with forklift activities. Thus, this research aims to minimize EC and OT with a multi-objective mixed-integer mathematical model by considering electric forklift operations. The proposed model addresses a lack of studies by controlling (i) order batching, (ii) batch assignment, (iii) batch sequencing, (iv) forklift routing, and (v) forklift battery charging schedule. The feasibility of the presented mathematical model is validated by solving small-sized examples. To solve medium- to large-sized case studies, we also propose and compare four multi-objective evolutionary algorithms (MOEAs). In illustrative examples, this study identifies the number of battery charging, orders, and forklifts as significant parameters affecting EC and OT. Our analysis also provides regression models connecting EC and OT from Pareto-optimal frontiers, and these results can help industrial practitioners and academic researchers find and investigate the relationship between EC and OT for making relevant decisions in warehouses served by electric forklifts. Among the four MOEAs developed, we show that the NSGA-II non-dominated sorting variable neighborhood search dynamic learning strategy (NSGA-VNS-DLS) outperforms other algorithms in accuracy, diversity, and CPU time.
更多查看译文
关键词
logistics, energy, multiple objective programming, evolutionary algorithms
AI 理解论文
溯源树
样例
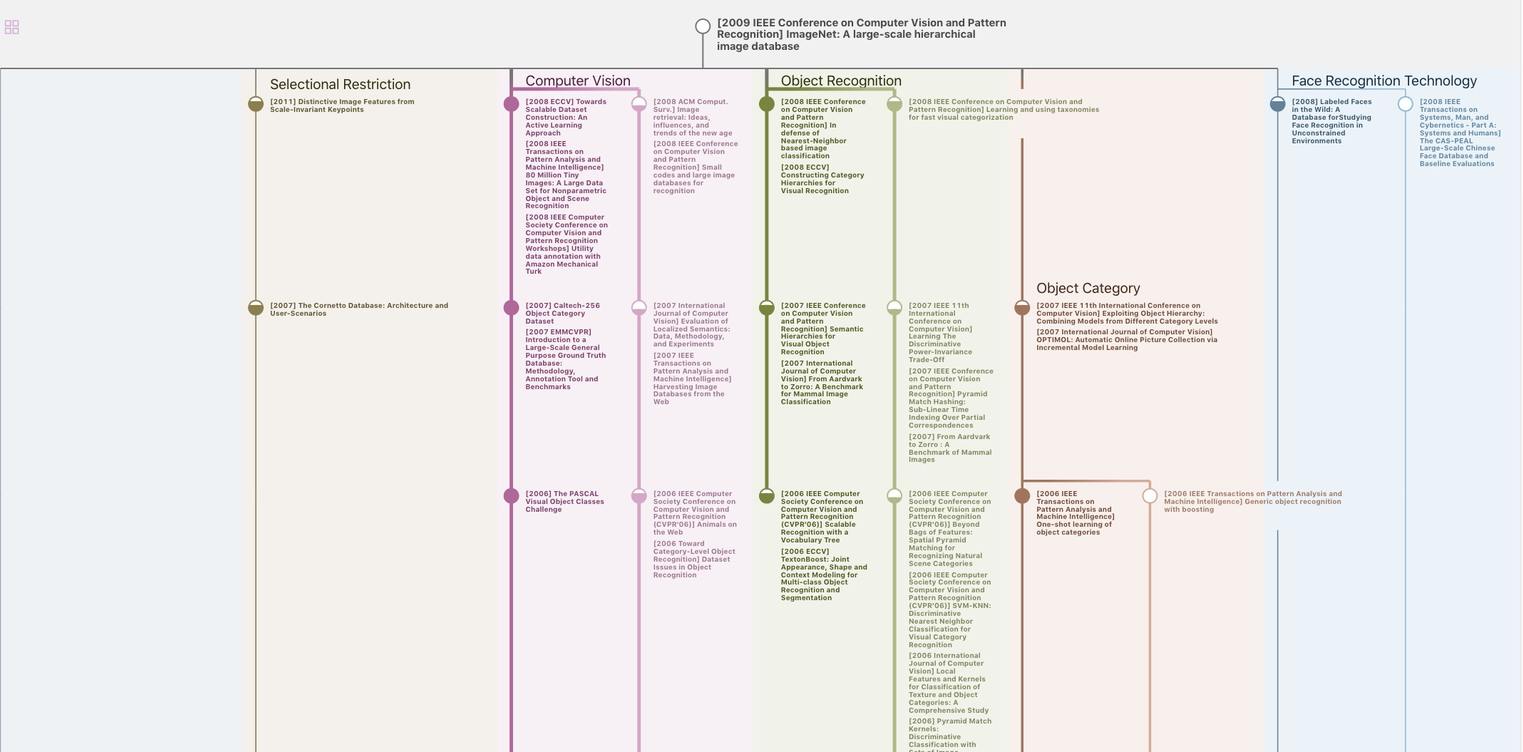
生成溯源树,研究论文发展脉络
Chat Paper
正在生成论文摘要