Local-Utopia Policy Selection For Multi-Objective Reinforcement Learning
PROCEEDINGS OF 2016 IEEE SYMPOSIUM SERIES ON COMPUTATIONAL INTELLIGENCE (SSCI)(2016)
摘要
Many real-world applications are characterized by multiple confticting objectives. In such problems, optimality is replaced by Pareto optimality and the goal is to find the Pareto frontier, a set 01' solutions representing different compromises among the objectives. Despite recent advances in multi-objective optimization, the selection, given the Pareto frontier, 01' a Pareto-optimal policy is still an important problem, prominent in practical applications such as economics and robotics. In this paper, we present a versatile approach for selecting a policy from the Pareto frontier according to user-defined preferences. Exploiting a novel scalarization function and heuristics, our approach provides an easy-to-use and effective method for Pareto-optimal policy selection. Furthermore, the scalarization is applicable in multiple-policy learning strategies tor approximating Pareto frontiers. To show the simplicity and effectiveness of our algorithm, we evaluate it on two problems and compare it to classical multi-objective reinforcement learning approaches.
更多查看译文
关键词
local-utopia policy selection,multiobjective reinforcement learning,Pareto optimality,multiobjective optimization,user-defined preferences,scalarization function,heuristics,Pareto-optimal policy selection,multiple-policy learning strategies,Pareto frontiers
AI 理解论文
溯源树
样例
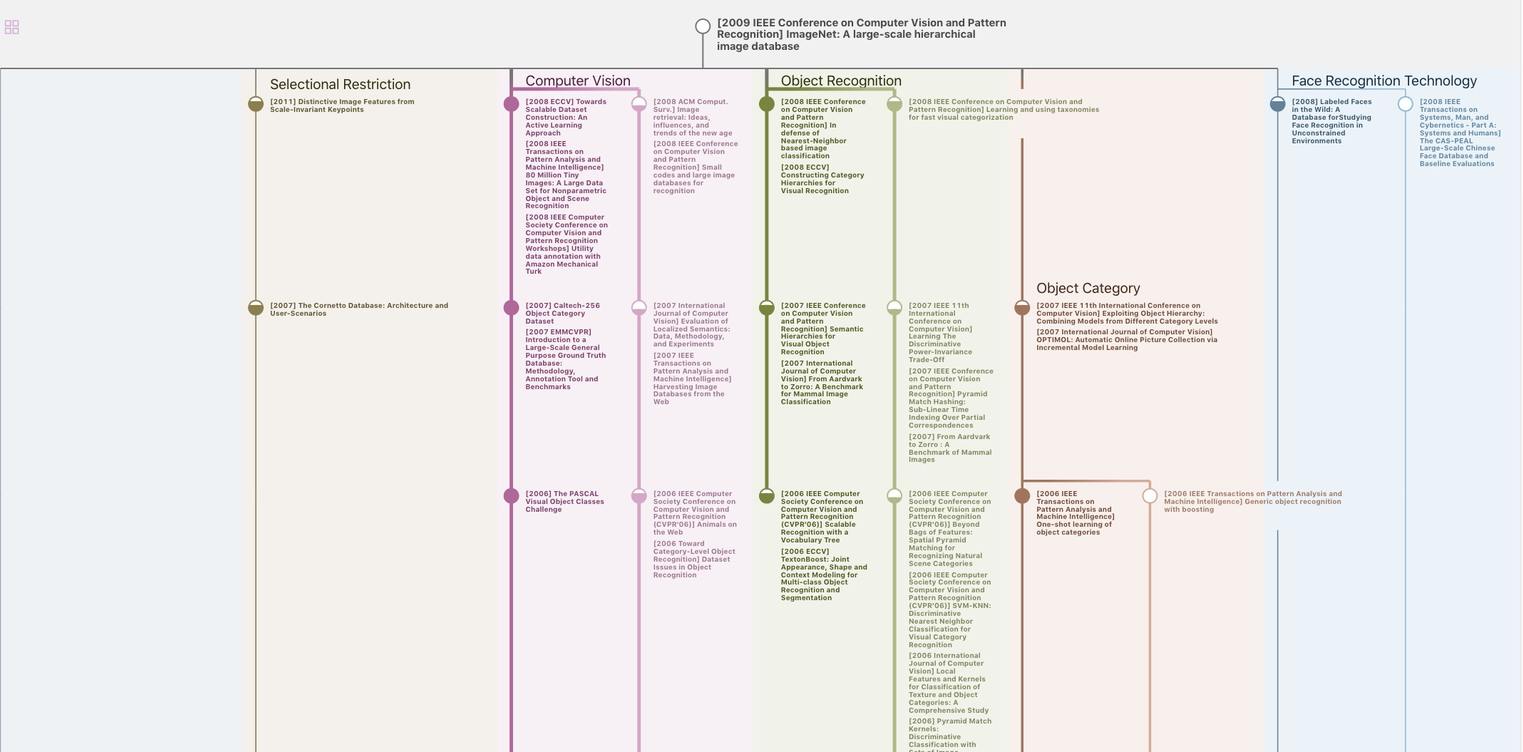
生成溯源树,研究论文发展脉络
Chat Paper
正在生成论文摘要