Low-Cost Sensor Performance Intercomparison, Correction Factor Development, and 2+Years of Ambient PM2.5 Monitoring in Accra, Ghana
ENVIRONMENTAL SCIENCE & TECHNOLOGY(2023)
摘要
Particulate matter air pollution is a leading cause ofglobal mortality,particularly in Asia and Africa. Addressing the high and wide-rangingair pollution levels requires ambient monitoring, but many low- andmiddle-income countries (LMICs) remain scarcely monitored. To addressthese data gaps, recent studies have utilized low-cost sensors. Thesesensors have varied performance, and little literature exists aboutsensor intercomparison in Africa. By colocating 2 QuantAQ Modulair-PM,2 PurpleAir PA-II SD, and 16 Clarity Node-S Generation II monitorswith a reference-grade Teledyne monitor in Accra, Ghana, we presentthe first intercomparisons of different brands of low-cost sensorsin Africa, demonstrating that each type of low-cost sensor PM2.5 is strongly correlated with reference PM2.5,but biased high for ambient mixture of sources found in Accra. Whencompared to a reference monitor, the QuantAQ Modulair-PM has the lowestmean absolute error at 3.04 & mu;g/m(3), followed by PurpleAirPA-II (4.54 & mu;g/m(3)) and Clarity Node-S (13.68 & mu;g/m(3)). We also compare the usage of 4 statistical or machine learningmodels (Multiple Linear Regression, Random Forest, Gaussian MixtureRegression, and XGBoost) to correct low-cost sensors data, and findthat XGBoost performs the best in testing (R (2): 0.97, 0.94, 0.96; mean absolute error: 0.56, 0.80, and 0.68 & mu;g/m(3) for PurpleAir PA-II, Clarity Node-S, and Modulair-PM,respectively), but tree-based models do not perform well when correctingdata outside the range of the colocation training. Therefore, we usedGaussian Mixture Regression to correct data from the network of 17Clarity Node-S monitors deployed around Accra, Ghana, from 2018 to2021. We find that the network daily average PM2.5 concentrationin Accra is 23.4 & mu;g/m(3), which is 1.6 times the WorldHealth Organization Daily PM2.5 guideline of 15 & mu;g/m(3). While this level is lower than those seen in some largerAfrican cities (such as Kinshasa, Democratic Republic of the Congo),mitigation strategies should be developed soon to prevent furtherimpairment to air quality as Accra, and Ghana as a whole, rapidlygrow. We develop four correctionfactor models for PurpleAir PA-II,Clarity Node-S, and QuantAQ Modulair-PM PM2.5 monitors,and then apply corrections to a network of 17 Clarity Node-S monitorsaround Accra, Ghana.
更多查看译文
关键词
air quality,low-cost sensors,PurpleAir,clarity,Modulair-PM,statistical methods,machine learning,urban air,sensor network,Ghana
AI 理解论文
溯源树
样例
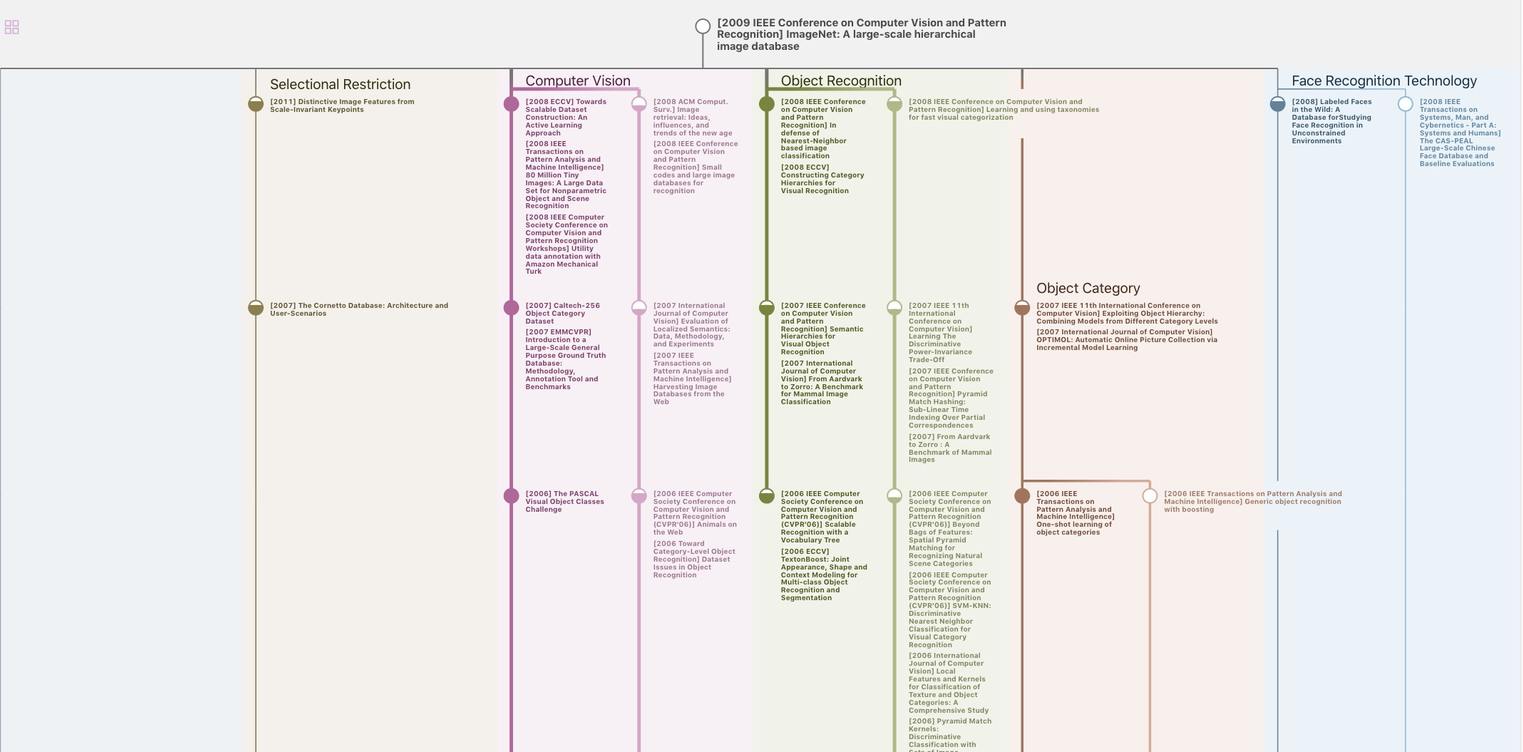
生成溯源树,研究论文发展脉络
Chat Paper
正在生成论文摘要