Airborne Radar Forward-Looking Imaging Algorithm Based on Generative Adversarial Networks
2023 24TH INTERNATIONAL RADAR SYMPOSIUM, IRS(2023)
摘要
Forward-looking radar imaging continues to gain significance due to a variety of convenient applications, like battlefield reconnaissance, target surveillance and precision guidance. Synthetic aperture radar (SAR) techniques are typically used to achieve a high azimuth resolution, but conventional SAR is not applicable in the forward direction because of the poor Doppler resolution and the "left-right" ambiguity problem. In recent years, there has been widespread use of generative adversarial networks (GAN), a common deep learning approach that has produced excellent results in inter-image translation. To apply GAN to radar forward-looking imaging, this paper builds an end-to-end forward-looking imaging network, whose output is a high-resolution image. The network eliminates the design and iterative processes of the observation matrix in imaging, which are common in conventional forward-looking imaging, increasing the effectiveness of real-time imaging. This study also proposes a method for generating training datasets for forward-looking imaging via existed high-resolution synthetic aperture radar images. As is verified by the simulated and real radar data, this approach has a robust recovery and better performance.
更多查看译文
AI 理解论文
溯源树
样例
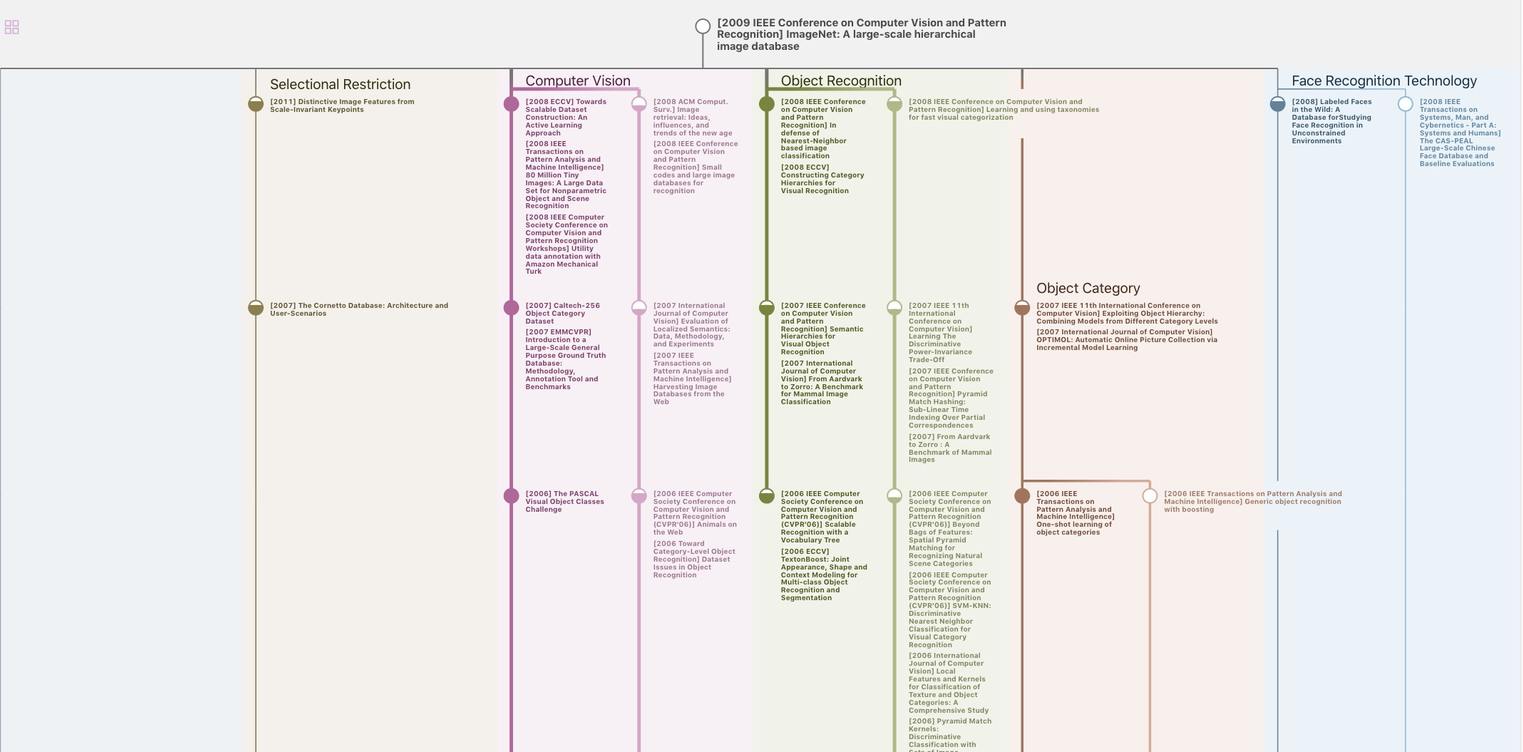
生成溯源树,研究论文发展脉络
Chat Paper
正在生成论文摘要