Exploring the Potential of Machine Learning to Predict Student Performance in an EM Course
2023 32ND ANNUAL CONFERENCE OF THE EUROPEAN ASSOCIATION FOR EDUCATION IN ELECTRICAL AND INFORMATION ENGINEERING, EAEEIE(2023)
摘要
In this paper, machine learning is explored to predict student performance based on data from Student-Led Tutorials (SLT) in an Electromagnetics Course from Eindhoven University of Technology. Since 2018, the course has seen an innovative approach to increase students' learning using SLTs. The amount and variety of student interactions captured through these SLTs provide data for gaining insight into the student learning process. The analysis presented here is part of the process towards the development of an Automated Prognostic Student Progress Monitoring System (APSPMS). Such a system, would allow for timely and direct feedback to both teachers and students. As an intermediate step to create this model, this paper discusses the indicators and predictors of student progress originating from the SLT as gathered in the previous years. Before each tutorial session, students indicate through a system which exercises they have prepared for that week SLTs. During the SLT, moderated by a teaching assistant, students are randomly selected to present the solution for an exercise that they have indicated preparedness for. This innovative intervention has increased the passing rates of the course from 40% to 60%. Two major indicators are used: the number of exercises the students have stated preparedness for, and voice recordings. Machine learning is applied to determine the accuracy of predicting whether a student will pass or fail using these indicators. For the voice recordings a prediction accuracy is achieved of 57.4% on our dataset. Although the data from the SLTs resulting from a four year study- can be implemented in different methods, it seems in this study that more data does not mean more information. In the end, the SLTs show an accuracy of 65+%. This shows that learning analytic and machine learning are promising techniques to predict student performance.
更多查看译文
关键词
student performance,education,machine learning,student-led tutorials
AI 理解论文
溯源树
样例
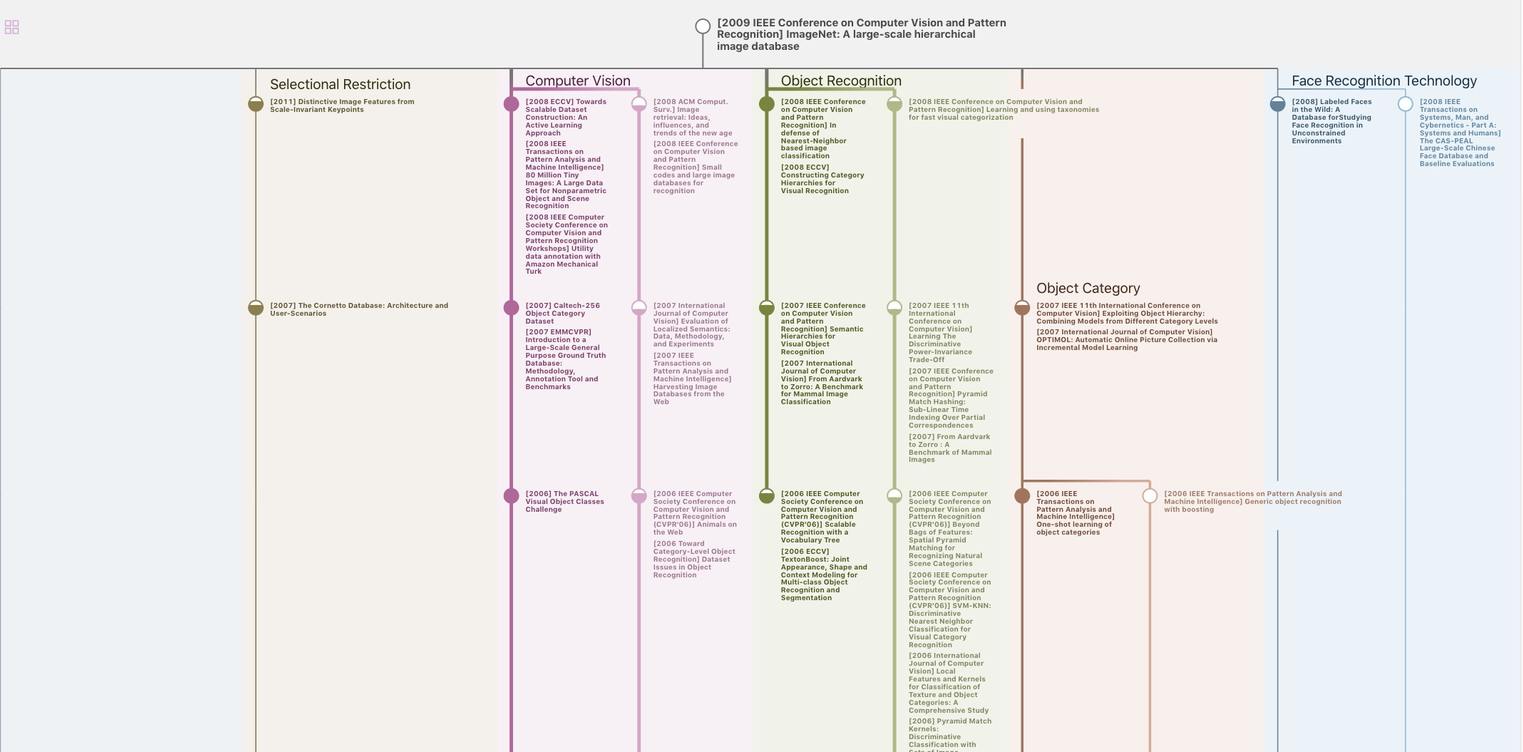
生成溯源树,研究论文发展脉络
Chat Paper
正在生成论文摘要