A High Accuracy and Low Power CNN-Based Environmental Sound Classification Processor
IEEE TRANSACTIONS ON CIRCUITS AND SYSTEMS I-REGULAR PAPERS(2023)
摘要
The environmental sound classification (ESC) has attracted increasing attention as the environmental sound contains a wealth of information that can be used to detect particular events. However, so far, most of the existing work in ESC still remains in the stage of algorithm design and the design of ESC processor has not been thoroughly investigated. The existing ESC processor designs have issues in meeting low power consumption and high accuracy simultaneously due to the lack of joint-optimization between algorithm and hardware, and very few work has demonstrated a complete ESC system containing all the necessary modules. In this work, a high accuracy and low power CNN-based ESC processor has been proposed, featuring: 1) a big-small CNN-based reconfigurable ESC processing hardware architecture to reduce the power consumption and hardware overhead while maintaining high classification accuracy. 2) a Mel feature adaptation engine reusing the neural network processing unit to further reduce the power consumption. 3) an event-driven ESC processing technique to reduce the inference time and the power consumption. The design has been implemented on a Kintex-7 FPGA and achieves low power consumption of 0.313W with high accuracy of 84.5% for the ESC-50 dataset, outperforming other state-of-the-art ESC processors.
更多查看译文
关键词
Environmental sound classification,CNN,processor,high accuracy,low power consumption
AI 理解论文
溯源树
样例
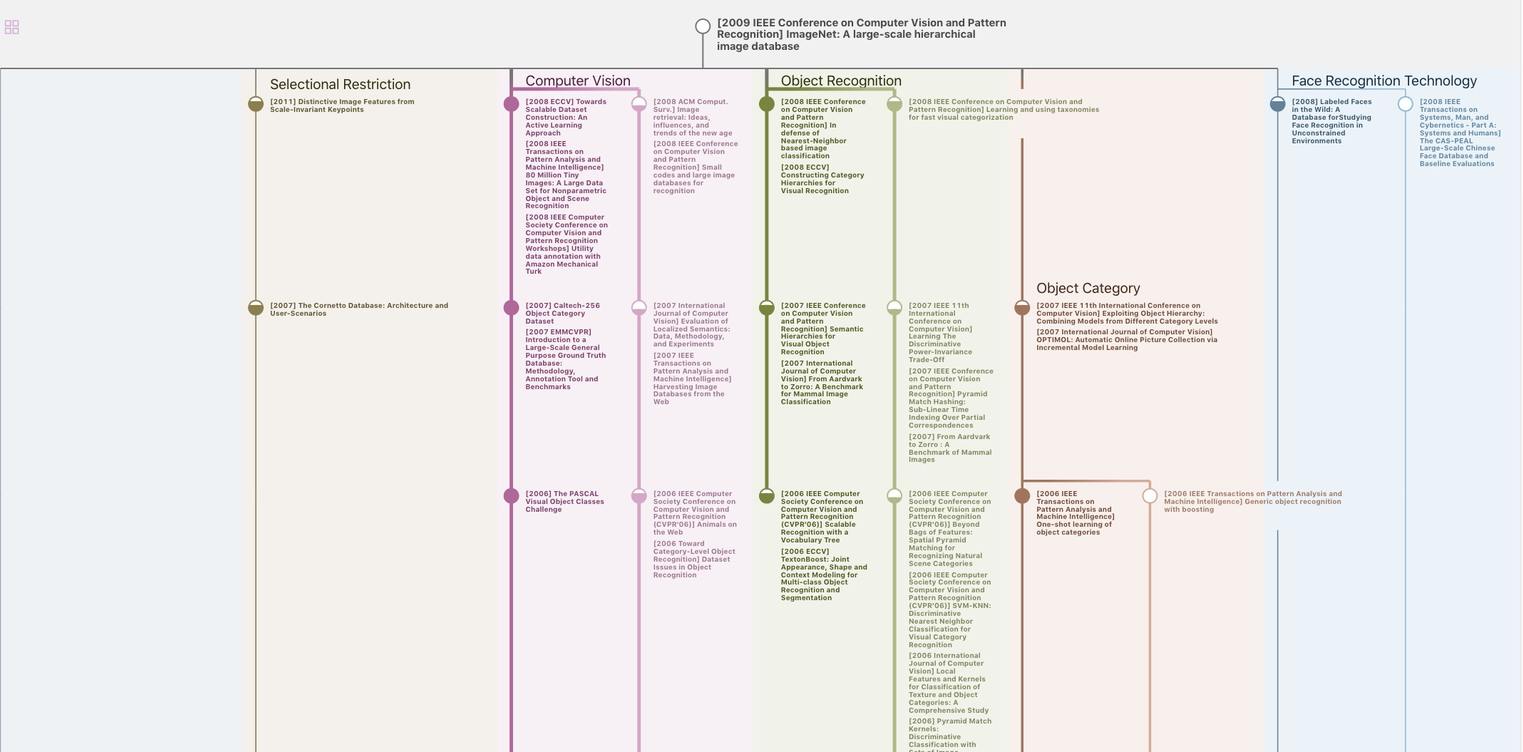
生成溯源树,研究论文发展脉络
Chat Paper
正在生成论文摘要