Geometry-Aware Network for Unsupervised Learning of Monocular Camera's Ego-Motion
IEEE TRANSACTIONS ON INTELLIGENT TRANSPORTATION SYSTEMS(2023)
摘要
Deep neural networks have been shown to be effective for unsupervised monocular visual odometry that can predict the camera's ego-motion based on an input of monocular video sequence. However, most existing unsupervised monocular methods haven't fully exploited the extracted information from both local geometric structure and visual appearance of the scenes, resulting in degraded performance. In this paper, a novel geometry-aware network is proposed to predict the camera's ego-motion by learning representations in both 2D and 3D space. First, to extract geometry-aware features, we design an RGB-PointCloud feature fusion module to capture information from both geometric structure and the visual appearance of the scenes by fusing local geometric features from depth-map-derived point clouds and visual features from RGB images. Furthermore, the fusion module can adaptively allocate different weights to the two types of features to emphasize important regions. Then, we devise a relevant feature filtering module to build consistency between the two views and preserve informative features with high relevance. It can capture the correlation of frame pairs in the feature-embedding space by attention mechanisms. Finally, the obtained features are fed into the pose estimator to recover the 6-DoF poses of the camera. Extensive experiments show that our method achieves promising results among the unsupervised monocular deep learning methods on the KITTI odometry and TUM-RGBD datasets.
更多查看译文
关键词
Monocular visual odometry,geometry-aware,point clouds,visual appearance,6-DoF poses
AI 理解论文
溯源树
样例
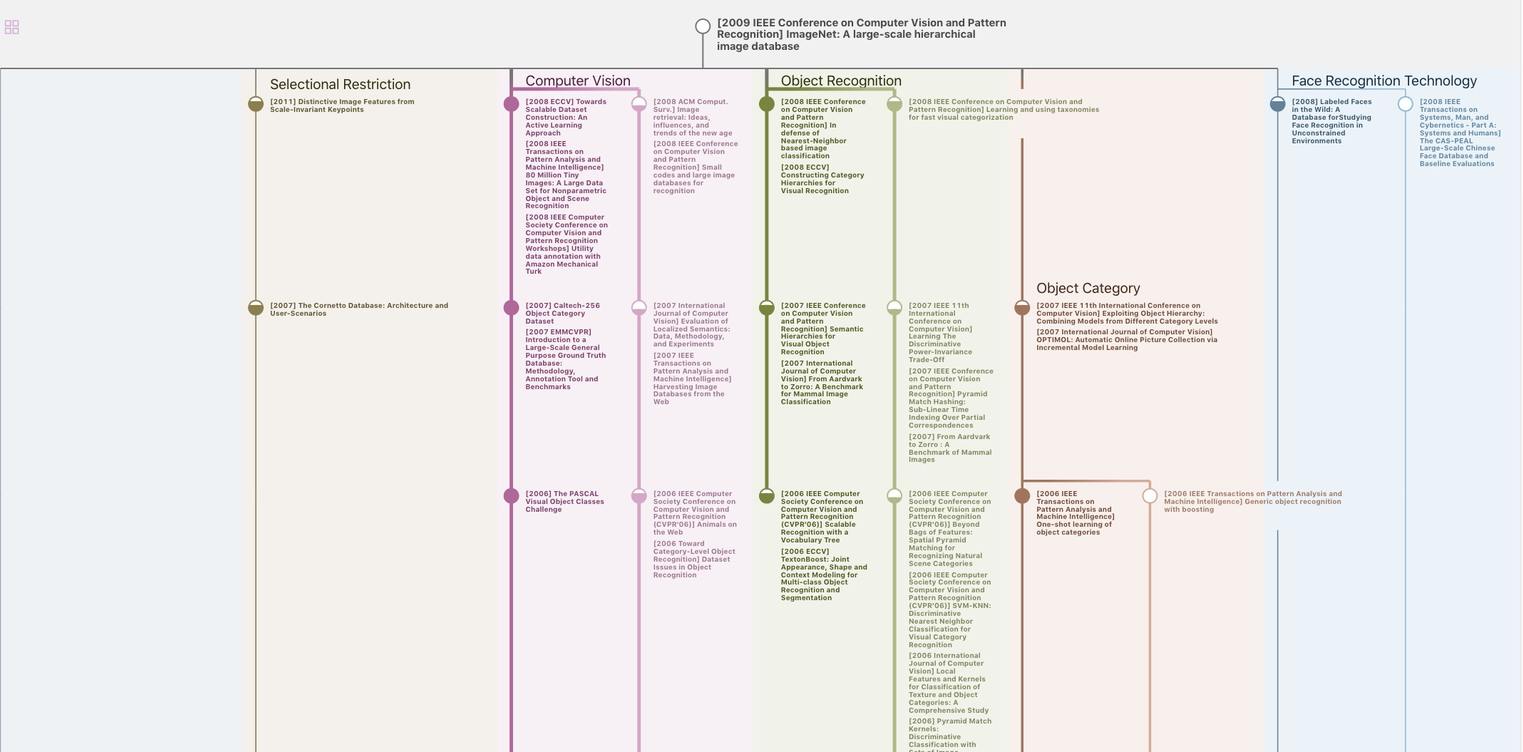
生成溯源树,研究论文发展脉络
Chat Paper
正在生成论文摘要