CSAT: Contrastive Sampling-Aggregating Transformer for Community Detection in Attribute-Missing Networks
IEEE TRANSACTIONS ON COMPUTATIONAL SOCIAL SYSTEMS(2023)
摘要
Community detection aims to identify dense subgroups of nodes within a network. However, in real-world networks, node attributes are often missing, making traditional methods less effective. In networks with missing attributes, the main challenge of community detection is to deal with the missing attribute information efficiently and use network structure information to make accurate predictions. This article proposes an innovative method called contrastive sampling-aggregating transformer (CSAT) for community detection in attribute-missing networks. CSAT incorporates the contrastive learning principle to capture hidden patterns among nodes and to aggregate information from different samples to create a more robust and accurate methodology for community detection. Specifically, CSAT utilizes a sampling and propagation strategy to obtain different samples and smooth attribute features of the network structure and leverages the Transformer architecture to model the pairwise relationships between nodes. Therefore, our method can address the attribute-missing issue by integrating the auxiliary information from both the network structure and other sources. Extensive experiments on several benchmark datasets demonstrate CSAT's superior performance compared to the state-of-the-art methods for community detection.
更多查看译文
关键词
Attribute-missing graph,community detection,deep graph representation learning,graph contrastive learning,Transformer
AI 理解论文
溯源树
样例
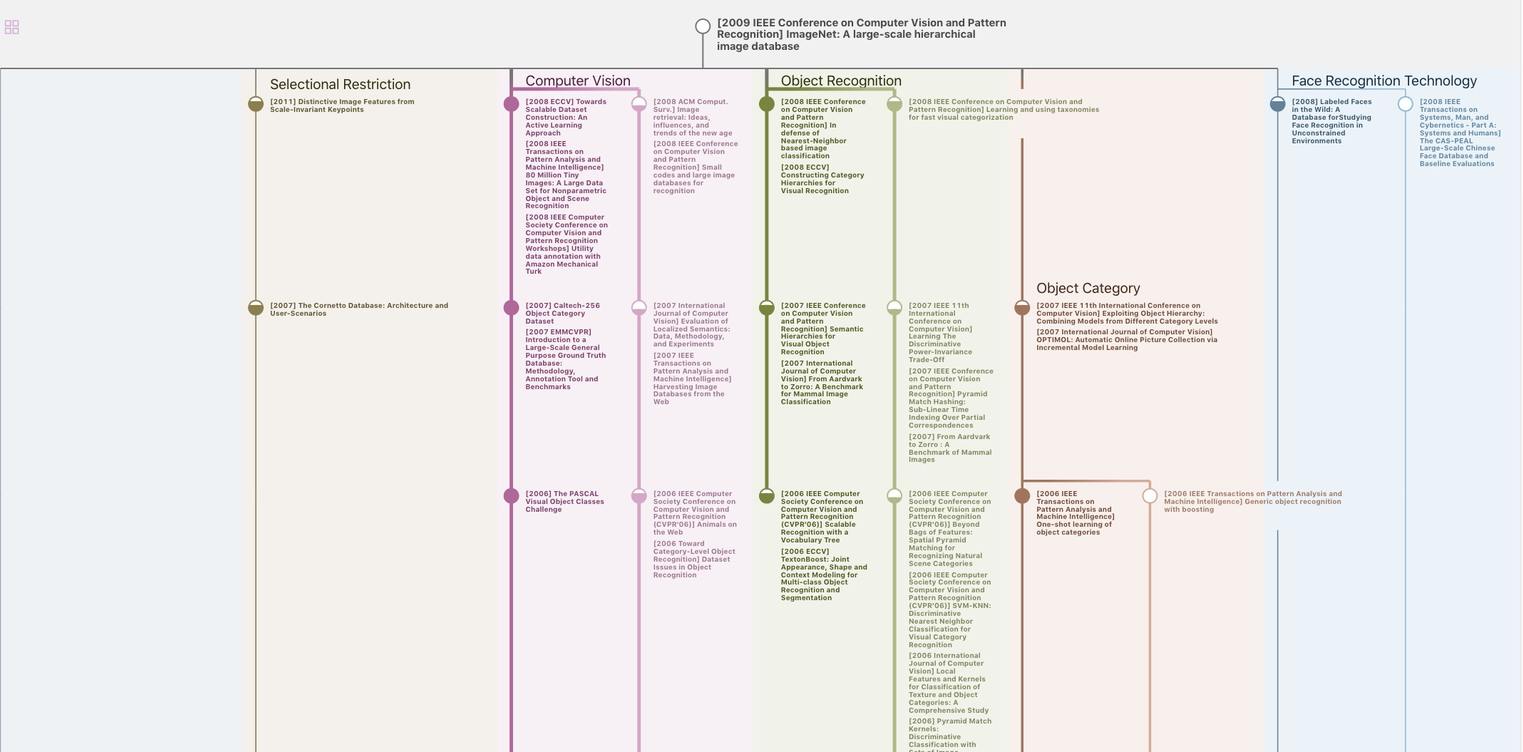
生成溯源树,研究论文发展脉络
Chat Paper
正在生成论文摘要