Solving nonstationary Markov decision processes via contextual decomposition: A military air battle management application
EXPERT SYSTEMS WITH APPLICATIONS(2023)
摘要
Reinforcement learning for nonstationary problems is a subject of widespread research given that most realistic problems do not exist within static environments. Approaching these problems can require significant effort in feature engineering to provide a learning algorithm with enough useful information about the state space to uncover complex system dynamics. As an alternative for problems with sufficient data describing the nonstationary environment, we propose the contextual decomposition Markov decision process (CDMDP) as a collection of stationary sub-problems intended to approximate nonstationary problem dynamics using a linear combination of value functions. We demonstrate the effectiveness of the CDMDP approach with an application in military air battle management. We use a designed computational experiment and analysis of variance to show that a complex, nonstationary learning problem can be effectively approximated with a small set of stationary sub-problems, and that the CDMDP solution significantly improves solution quality over a baseline approach without the need for additional feature engineering. If a researcher suspects that a complex and continuously varying environment can be approximated by a small number of stationary contexts, the CDMDP framework may save significant computational resources and yield decision policies that are much easier to visualize and implement.
更多查看译文
关键词
Reinforcement learning,Nonstationary Markov decision process,Context detection,Dynamic assignment problem,OR in defense,Air battle management
AI 理解论文
溯源树
样例
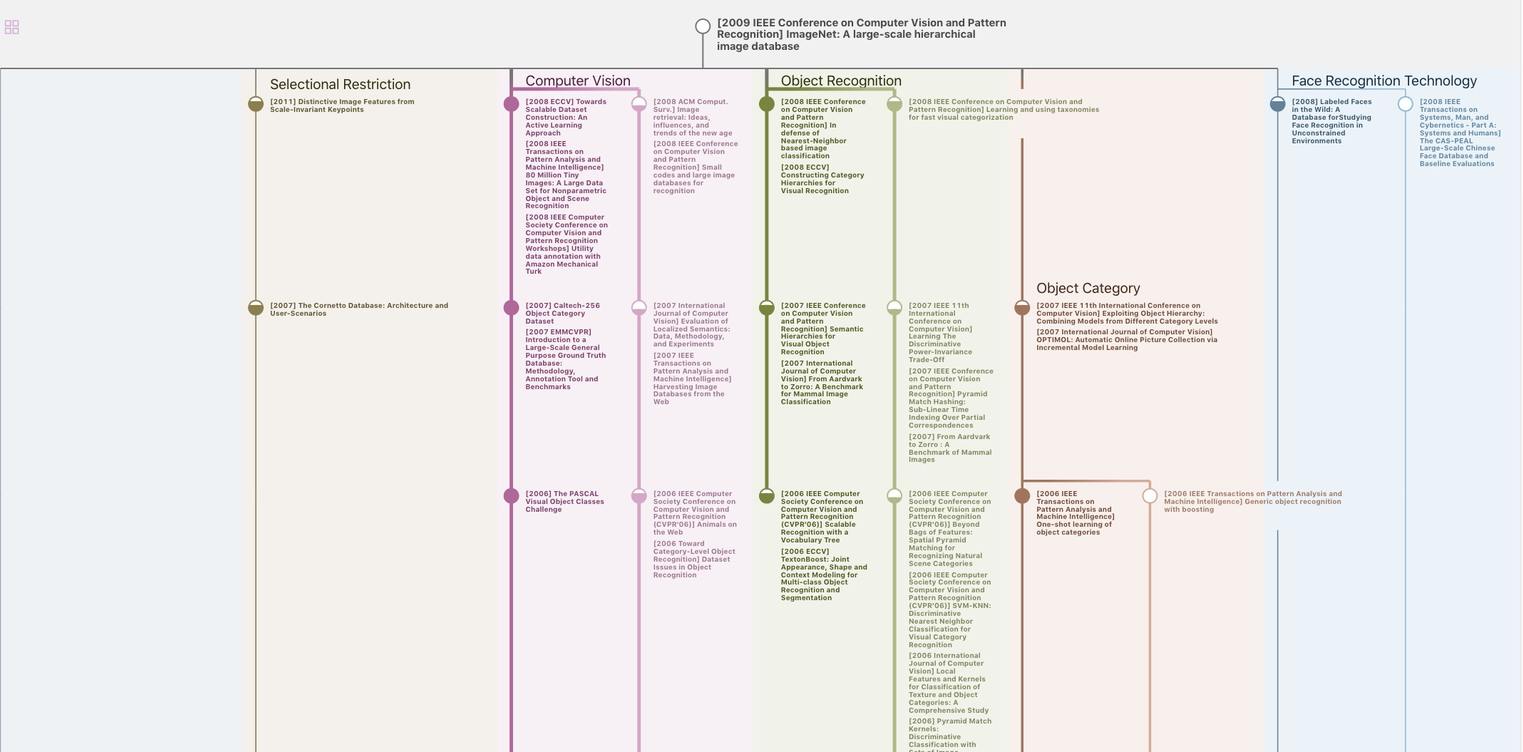
生成溯源树,研究论文发展脉络
Chat Paper
正在生成论文摘要