Feasibility Of Artificial Intelligence Automated Detection And Classification Of Heart Failure From Routine Electronic Health Records
JOURNAL OF CARDIAC FAILURE(2023)
摘要
Introduction The increasing availability of electronic health records (EHRs), including not only medical records but also imaging DICOMs, offers opportunities for enhanced patient care through automated case detection and surveillance. Hypothesis We hypothesized that artificial intelligence (AI) automation could be used to identify cases of heart failure (HF) and classify the type of HF (with preserved or reduced ejection fraction [HFpEF or HFrEF]) from routine EHR surveillance. Methods We performed a population-based cohort study of EHR data covering the population of Tayside and Fife since 1993, covering ∼20% of the Scottish population. Using a patient-specific identifier, linkage to other clinical datasets was possible, including community-dispensed prescriptions, echocardiography DICOM images, hospital admissions (Scottish Morbidity Record), and mortality data (General Registry Office). A combination of keyword search of medical records and AI automated reading of stored echocardiographic DICOM images (Us2.ai) was used to identify patients with HFpEF, HFrEF and controls without HF. Verification of the final diagnosis was performed through manual review of medical records, measurement of natriuretic peptides in stored blood samples of identified patients, and comparison of clinical outcomes among groups. Results Among 3680 patient records with linkage to echocardiographic DICOMs and blood samples, we identified 236 patients with HFpEF, 156 with HFrEF, and 185 controls (Table). Compared to HFrEF, patients with HFpEF were older, more often women and had less coronary artery disease. Compared to controls, patients with HFpEF and HFrEF had greater LV mass, higher mitral E/e’ ratio, higher pulmonary artery systolic pressure, more impaired LV strain and RV dysfunction. While majority of patients with HFrEF were on guideline-directed medical therapy, a gap in treatment was identified for mineralocorticoid receptor antagonists. NT-proBNP was higher in HFpEF, and even higher in HFrEF, compared to controls. During a median follow up time of 1089 days, 39% of HFpEF and 63% of HFrEF experienced hospitalization or death, compared to only 5% of controls. Conclusions We demonstrate the feasibility of AI-automated detection and classification of HF from routine surveillance of electronic health data, yielding cohorts with clinical and echocardiographic characteristics similar to epidemiologic studies, with raised natriuretic peptides and poor outcomes expected in these patient populations. Such AI-assisted electronic surveillance may have application in automated monitoring of clinical records for early disease detection, treatment quality improvement initiatives, and case finding for clinical trials.
更多查看译文
关键词
heart failure,artificial intelligence automated detection,artificial intelligence,classification
AI 理解论文
溯源树
样例
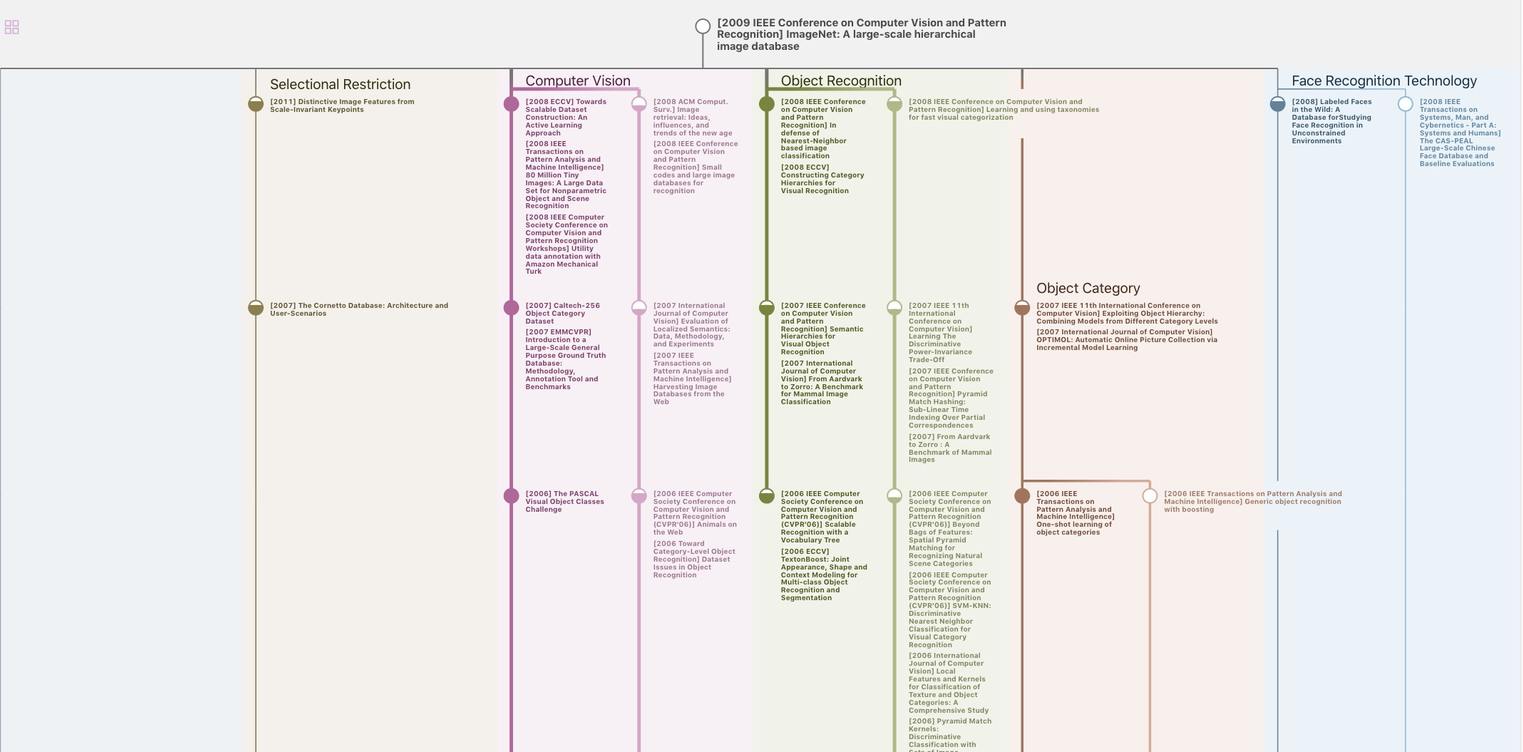
生成溯源树,研究论文发展脉络
Chat Paper
正在生成论文摘要