Traffic Flow Forecasting of Graph Convolutional Network Based on Spatio-Temporal Attention Mechanism
INTERNATIONAL JOURNAL OF AUTOMOTIVE TECHNOLOGY(2023)
摘要
Accurate traffic flow forecasting is a prerequisite guarantee for the realization of intelligent transportation. Due to the complex time and space features of traffic flow, its forecasting has always been a research hotspot in this field. Aiming at the difficulty of capturing and modelling the temporal and spatial correlation and dynamic features of traffic flow, this paper proposes a novel graph convolutional network traffic flow forecasting model (STAGCN) based on the temporal and spatial attention mechanism. STAGCN model is mainly composed of three modules: Spatio-temporal Attention (STA-Block), Graph Convolutional Network (GCN) and Standard Convolutional Network (CN), model the periodicity, spatial correlation and time dependence of traffic flow respectively. STA-Block module models the spatio-temporal correlation between different time steps through the spatio-temporal attention mechanism and gating fusion mechanism, and uses GCN and CN to capture the spatial and temporal features of traffic flow respectively. Finally, the output of the three components is predicted through a gated fusion mechanism. A large number of experiments have been conducted on two data sets of PeMS. The experimental results demonstrate that compared with the baseline method, the STAGCN model proposed in this paper has better forecasting performance.
更多查看译文
关键词
traffic flow forecasting,graph convolutional network,spatio-temporal
AI 理解论文
溯源树
样例
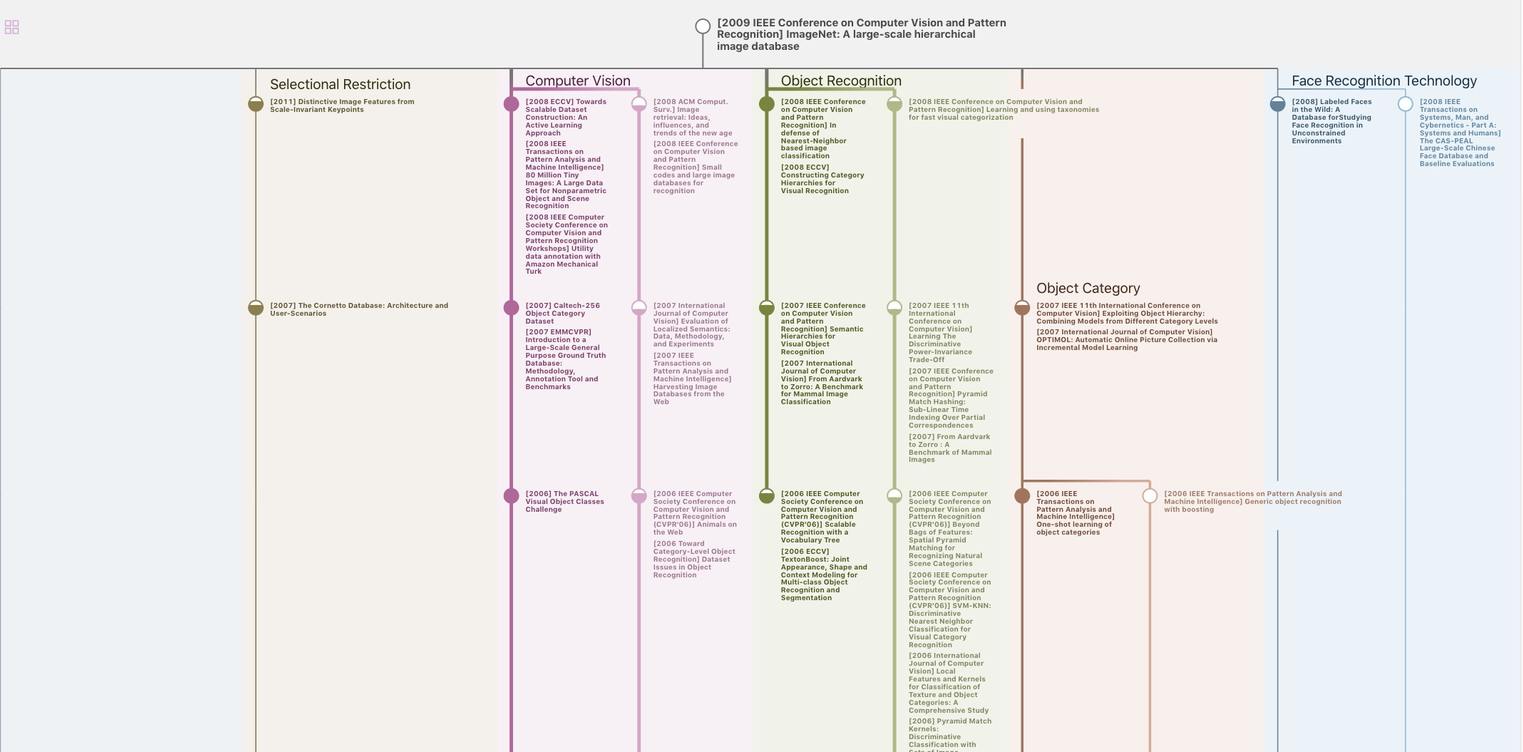
生成溯源树,研究论文发展脉络
Chat Paper
正在生成论文摘要