M3D-NCA: Robust 3D Segmentation with Built-In Quality Control
MEDICAL IMAGE COMPUTING AND COMPUTER ASSISTED INTERVENTION, MICCAI 2023, PT III(2023)
摘要
Medical image segmentation relies heavily on large-scale deep learning models, such as UNet-based architectures. However, the real-world utility of such models is limited by their high computational requirements, which makes them impractical for resource-constrained environments such as primary care facilities and conflict zones. Furthermore, shifts in the imaging domain can render these models ineffective and even compromise patient safety if such errors go undetected. To address these challenges, we propose M3D-NCA, a novel methodology that leverages Neural Cellular Automata (NCA) segmentation for 3D medical images using n-level patchification. Moreover, we exploit the variance in M3D-NCA to develop a novel quality metric which can automatically detect errors in the segmentation process of NCAs. M3D-NCA outperforms the two magnitudes larger UNet models in hippocampus and prostate segmentation by 2% Dice and can be run on a Raspberry Pi 4 Model B (2GB RAM). This highlights the potential of M3D-NCA as an effective and efficient alternative for medical image segmentation in resource-constrained environments.
更多查看译文
关键词
Neural Cellular Automata,Medical Image Segmentation,Automatic Quality Control
AI 理解论文
溯源树
样例
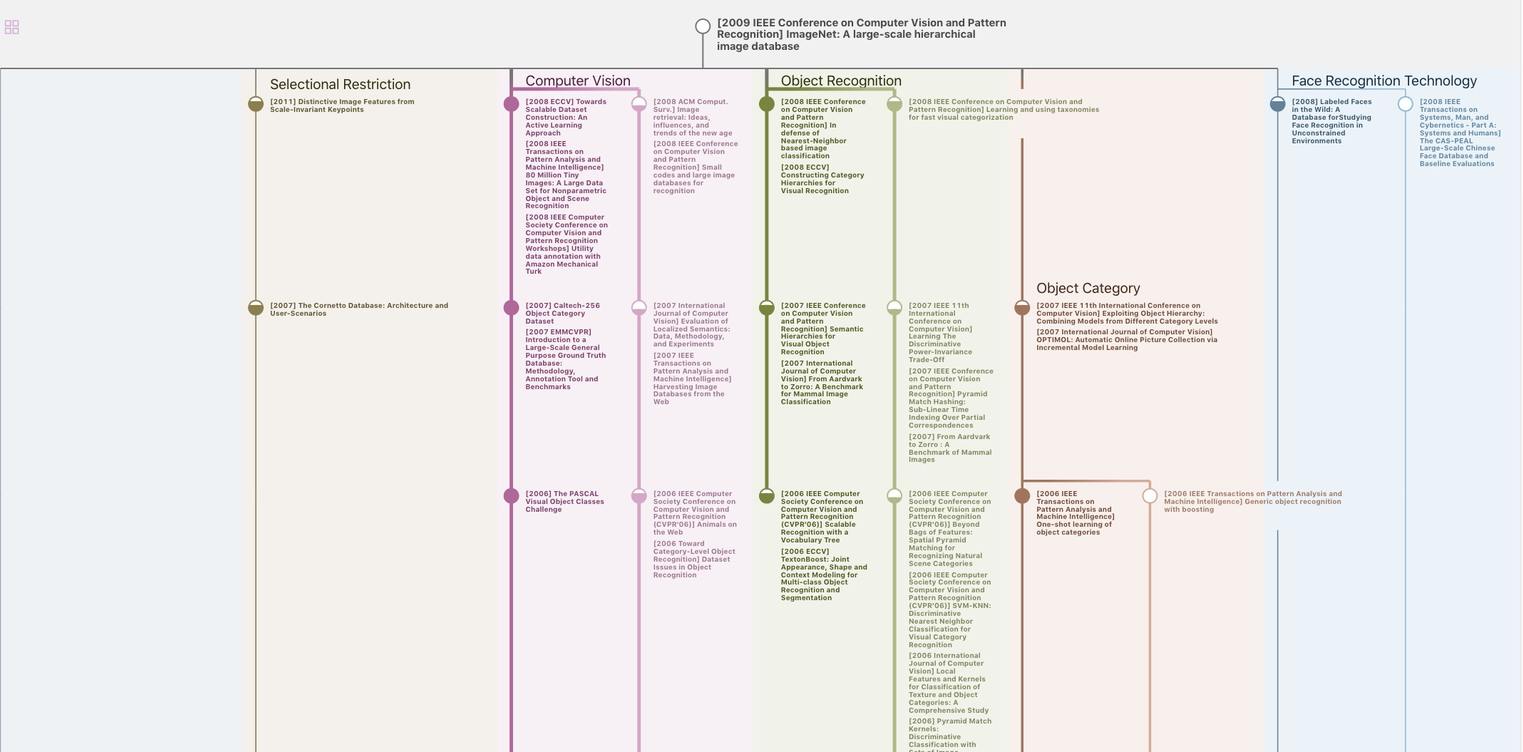
生成溯源树,研究论文发展脉络
Chat Paper
正在生成论文摘要