RSDiff: Remote Sensing Image Generation from Text Using Diffusion Model
CoRR(2023)
摘要
Satellite imagery generation and super-resolution are pivotal tasks in remote sensing, demanding high-quality, detailed images for accurate analysis and decision-making. In this paper, we propose an innovative and lightweight approach that employs two-stage diffusion models to gradually generate high-resolution Satellite images purely based on text prompts. Our innovative pipeline comprises two interconnected diffusion models: a Low-Resolution Generation Diffusion Model (LR-GDM) that generates low-resolution images from text and a Super-Resolution Diffusion Model (SRDM) conditionally produced. The LR-GDM effectively synthesizes low-resolution by (computing the correlations of the text embedding and the image embedding in a shared latent space), capturing the essential content and layout of the desired scenes. Subsequently, the SRDM takes the generated low-resolution image and its corresponding text prompts and efficiently produces the high-resolution counterparts, infusing fine-grained spatial details and enhancing visual fidelity. Experiments are conducted on the commonly used dataset, Remote Sensing Image Captioning Dataset (RSICD). Our results demonstrate that our approach outperforms existing state-of-the-art (SoTA) models in generating satellite images with realistic geographical features, weather conditions, and land structures while achieving remarkable super-resolution results for increased spatial precision.
更多查看译文
关键词
remote sensing image generation,remote sensing,diffusion model,text
AI 理解论文
溯源树
样例
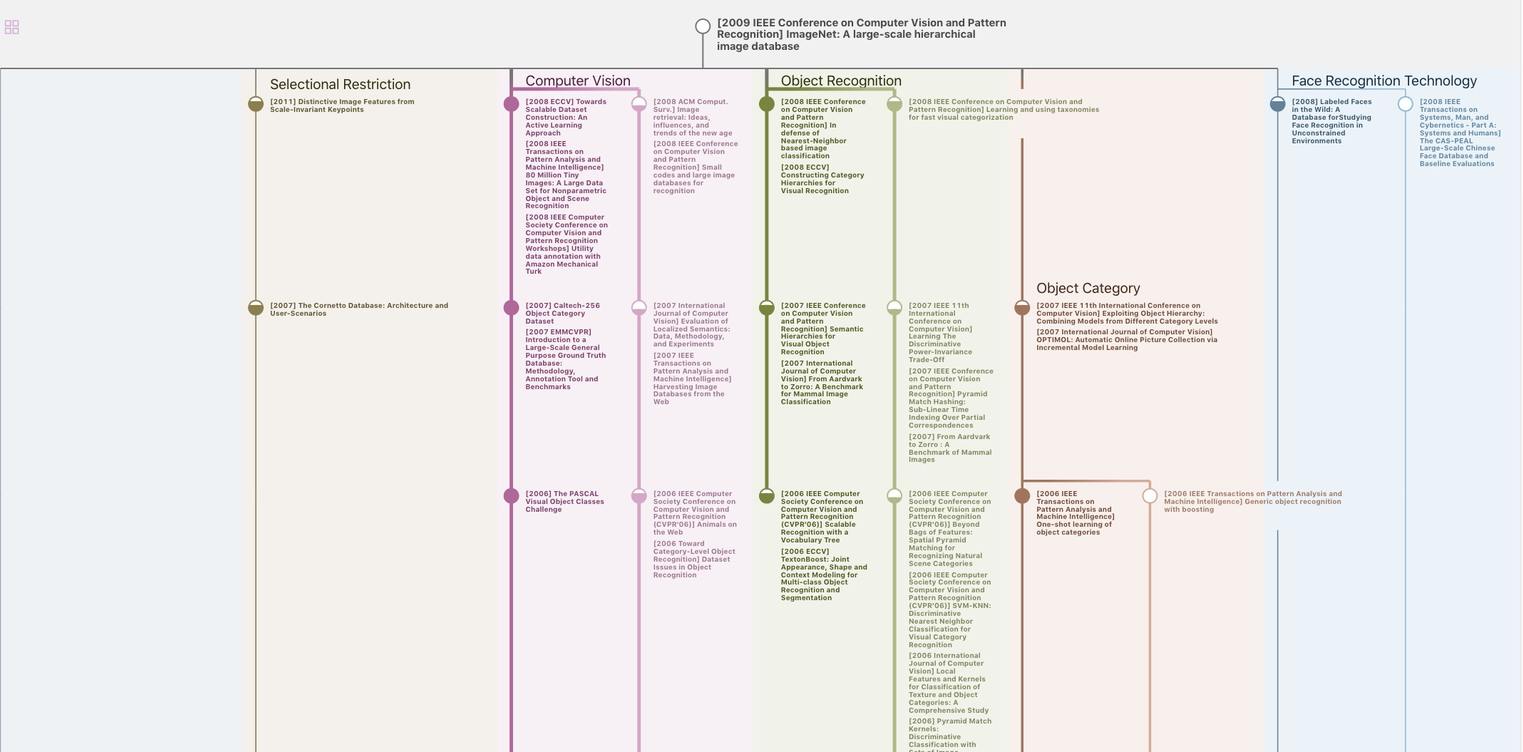
生成溯源树,研究论文发展脉络
Chat Paper
正在生成论文摘要