CycleGAN-Based Visible-Infrared Image Enhancement Method for Infrared Power Equipment Object Detection
2023 IEEE 5th International Conference on Power, Intelligent Computing and Systems (ICPICS)(2023)
摘要
In the realm of electrical engineering, object detection in infrared images is paramount for the maintenance of power equipment. However, the scarcity of infrared image datasets severely impedes the improvement of object detection technology for infrared images. To address this issue, we propose a data enhancement method based on Cycle-consistent Generative Adversarial Networks (CycleGAN), which can translate easily available visible images of power equipment into less accessible infrared images and achieve to expand the pool of infrared images of power equipment. Because of the cycle-consistent loss function, CycleGAN can realize image-to-image translation in the absence of paired data, therefore breaking away from the traditional requirement of needing difficult-to-obtain paired input datasets. In addition, we utilize the LSGAN loss function to prevent mode collapse. The experimental results show that our method can generate high-quality infrared images which are nearly indistinguishable from the real captured images. And the performance of the object detection algorithm is significantly improved by the enhanced dataset, proving our method is effective in infrared dataset enhancement. As an unsupervised data enhancement approach, it can also provide an idea that can be employed in other fields with limited data.
更多查看译文
关键词
Cycle-consistent Generative Adversarial Networks,data enhancement,object detection,infrared image generation,power equipment
AI 理解论文
溯源树
样例
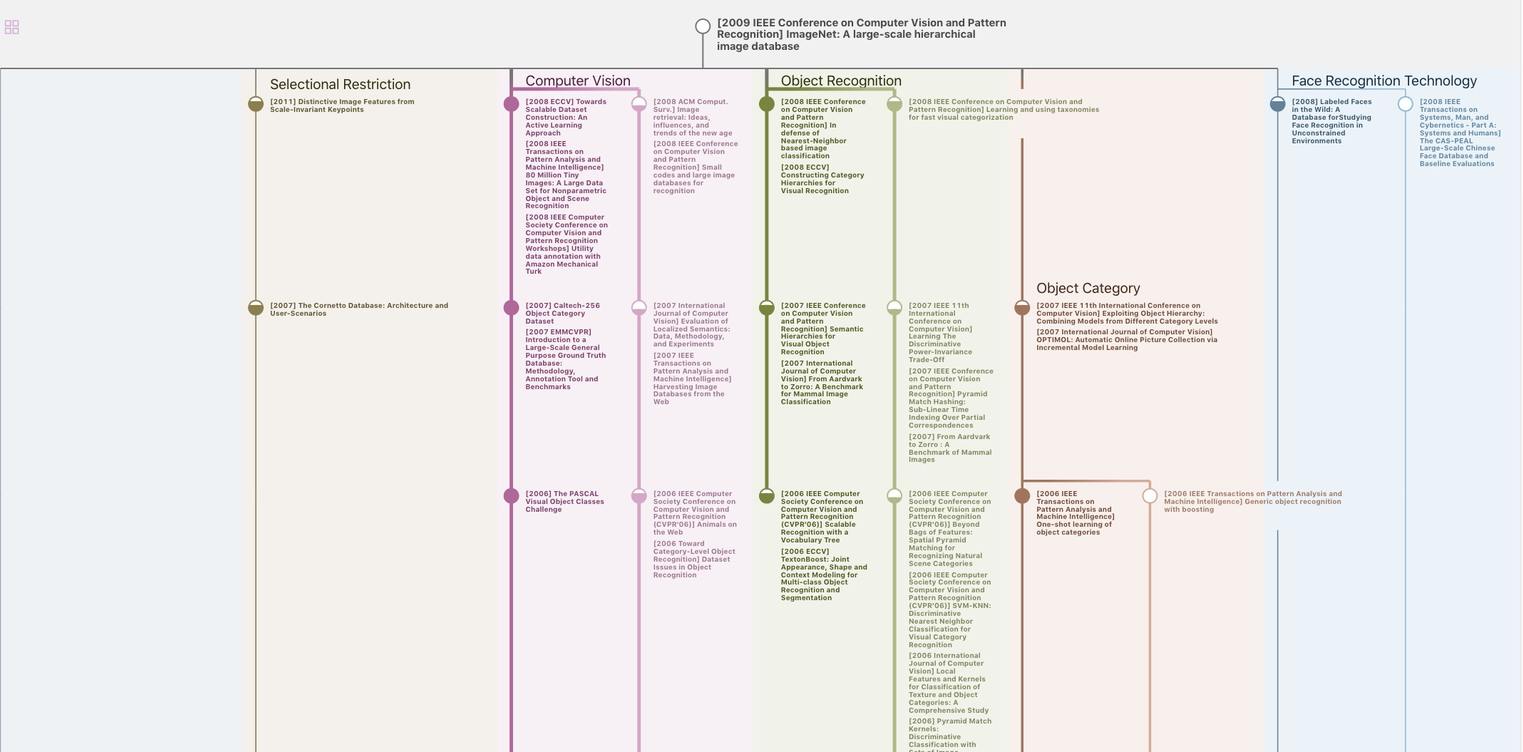
生成溯源树,研究论文发展脉络
Chat Paper
正在生成论文摘要